Learning to Predict Keypoints and Structure of Articulated Objects without Supervision
ICPR(2022)
摘要
Reasoning about the structure and motion of novel object classes is a core ability in human cognition, crucial for manipulating objects and predicting their possible motion. We present a method that learns to infer the skeleton structure of a novel articulated object from a single image, in terms of joints and rigid links connecting them. The model learns without supervision from a dataset of objects having diverse structures, in different poses and states of articulation. To achieve this, it is trained to explain the differences between pairs of images in terms of a latent skeleton that defines how to transform one into the other. Experiments on several datasets show that our model predicts joint locations significantly more accurately than prior works on unsupervised keypoint discovery; moreover, unlike existing methods, it can predict varying numbers of joints depending on the observed object. It also successfully predicts the connections between joints, even for structures not seen during training.
更多查看译文
关键词
articulated objects,keypoints,learning,supervision
AI 理解论文
溯源树
样例
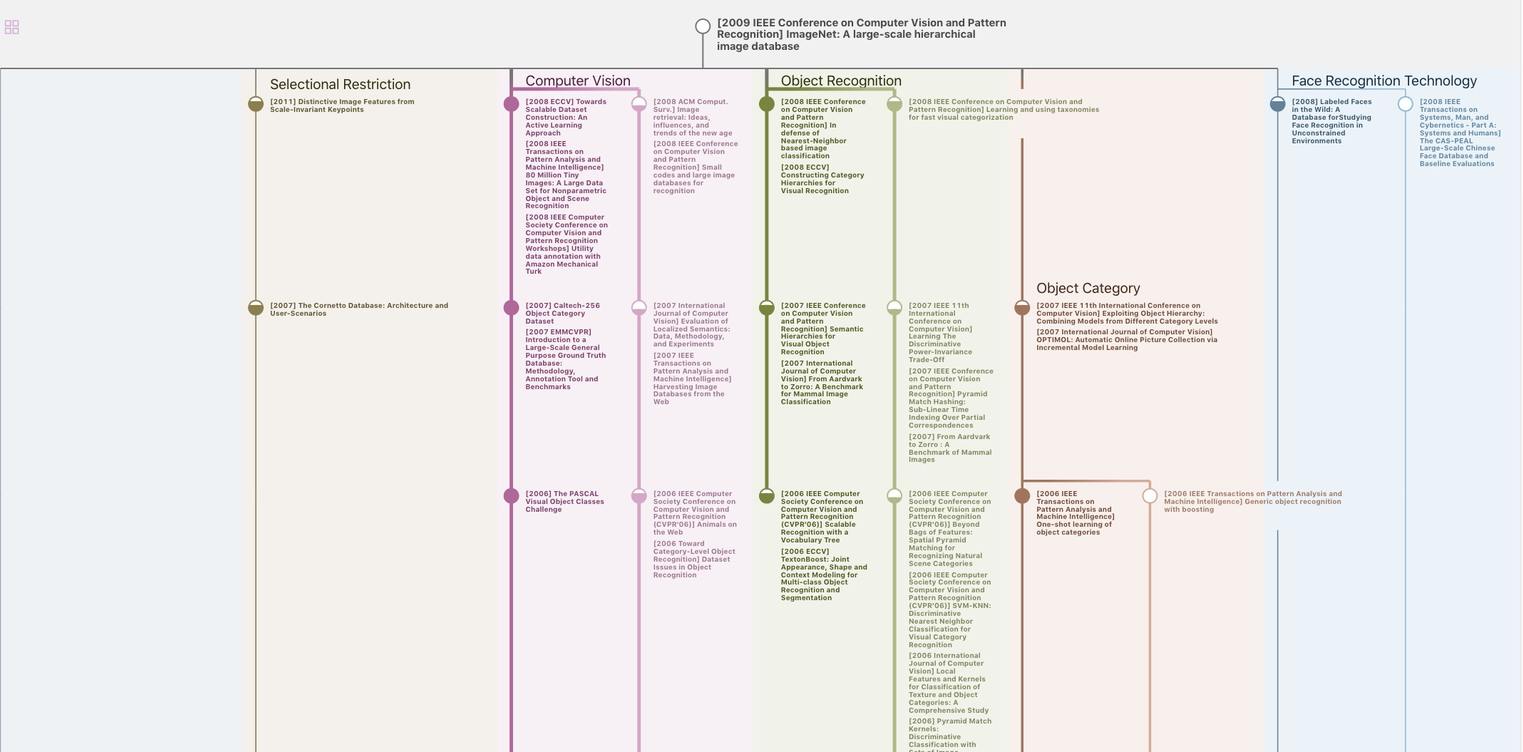
生成溯源树,研究论文发展脉络
Chat Paper
正在生成论文摘要