Multimodal Self-Supervised Learning Networks for In-vehicle Noise Prediction
Gyeongyeong gwahag - Hangug gyeongyeong gwahaghoe/Gyeong'yeong gwahag - Han'gug gyeong'yeong gwahaghoe(2022)
摘要
Predicting in-vehicle noise levels is an important issue in automobile industry. In most previous studies, various supervised learning methods that use both the input and output(labeled) variables are used to predict automobile noise levels. However, collecting labeled data for in-vehicle noise prediction is time consuming and expensive. In this study, we propose a multimodal self-supervised learning framework that can predict in-vehicle noise levels with only a small amount of labeled data, so that resources required to collect labeled data can be saved. In our framework, both original acceleration signals and spectrograms converted from the original data are used as the input to predict in-vehicle noise levels. In the first stage, we pretrain the features of the input data based on the relationship between the signal and spectrogram data using only unlabeled data, which is much easier to collect than labeled data. In the second stage, we use a small amount of data to construct the in-vehicle noise prediction model with the pretrained feature extractor. The effectiveness and applicability of the proposed framework are demonstrated using the actual acceleration signal data collected from various locations of electric power steering vehicle noise levels. The proposed framework outperforms the existing supervised learning method especially when a few labeled data are available.
更多查看译文
AI 理解论文
溯源树
样例
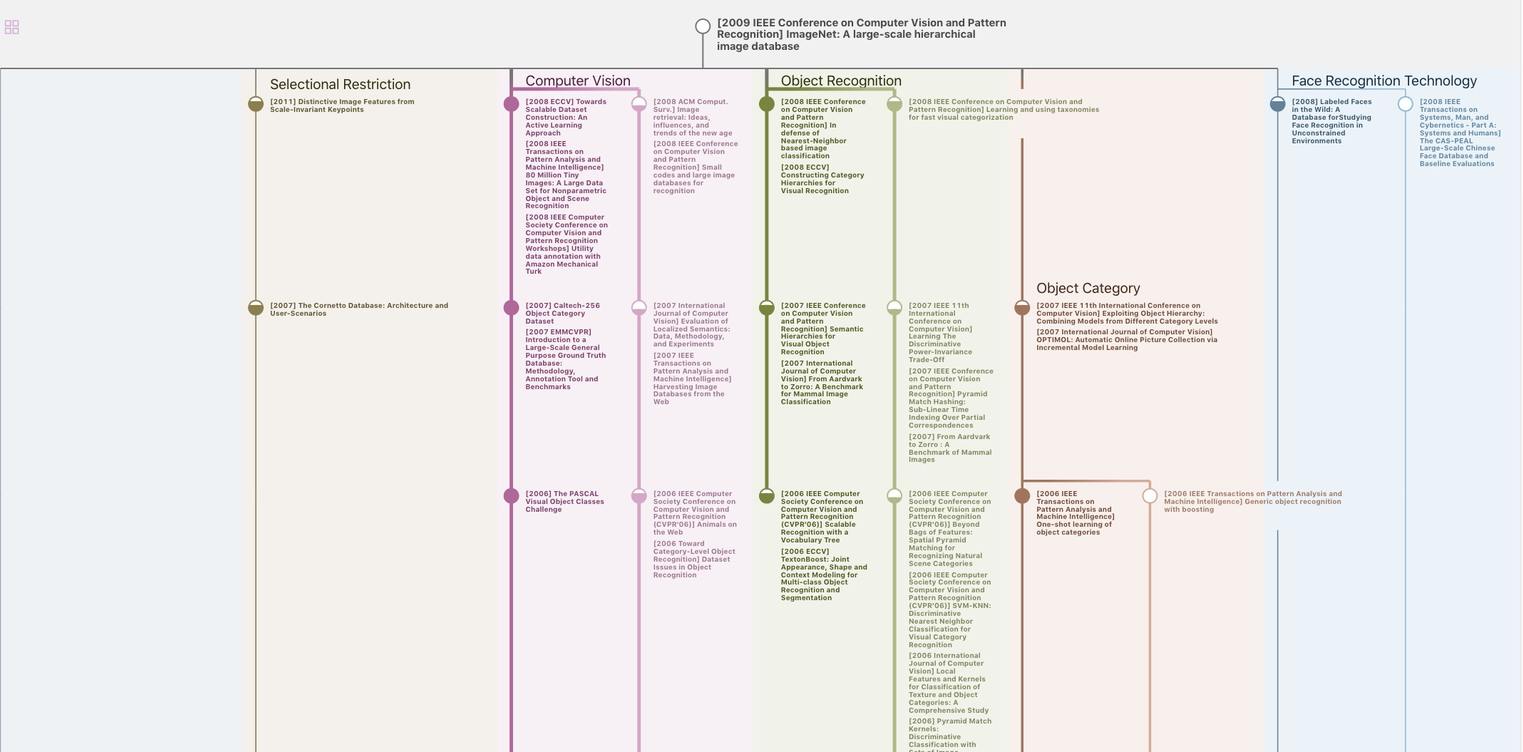
生成溯源树,研究论文发展脉络
Chat Paper
正在生成论文摘要