Hybridizing machine learning with metaheuristics for preventing convergence failures in mechanical models based on compression field theories.
Appl. Soft Comput.(2022)
摘要
Some materials, such as reinforced concrete (RC), introduce non-linear constitutive relationships into structural models. The characterization of their structural load-strain response is usually approached by using iterative strategies, such as Newton-type methods. However, these iterative strategies may not predict the non-linear structural response of RC elements (beams) due to convergence problems (non-consistent elements). In this work we propose a novel solution to overcome the convergence failures of RC structural elements subjected to shear force modeled by Compression Field Theories (CFTs). First, a Coral Reef Optimization with Substrate Layers (CRO-SL) metaheuristic is used to solve a fitting problem between numerical and experimental results on a set of real beams tested in shear, for which the system is solvable. Then, the outputs of the CRO-SL, plus the mechanical and geometric parameters of the RC beams, are used to train different Machine Learning (ML) regression methods. These regressors are then able to predict the tensile strains of the non-consistent RC elements. The characterization of such strains allows modifying the parameterization of the system of equations, overcoming thus the convergence problem of Newton-type methods in these cases. Experiments over a set of 72 real RC beams have shown the good performance of our approach. In them we have carried
更多查看译文
关键词
Compression field theories,Solvability,ML regressors,Metaheuristics
AI 理解论文
溯源树
样例
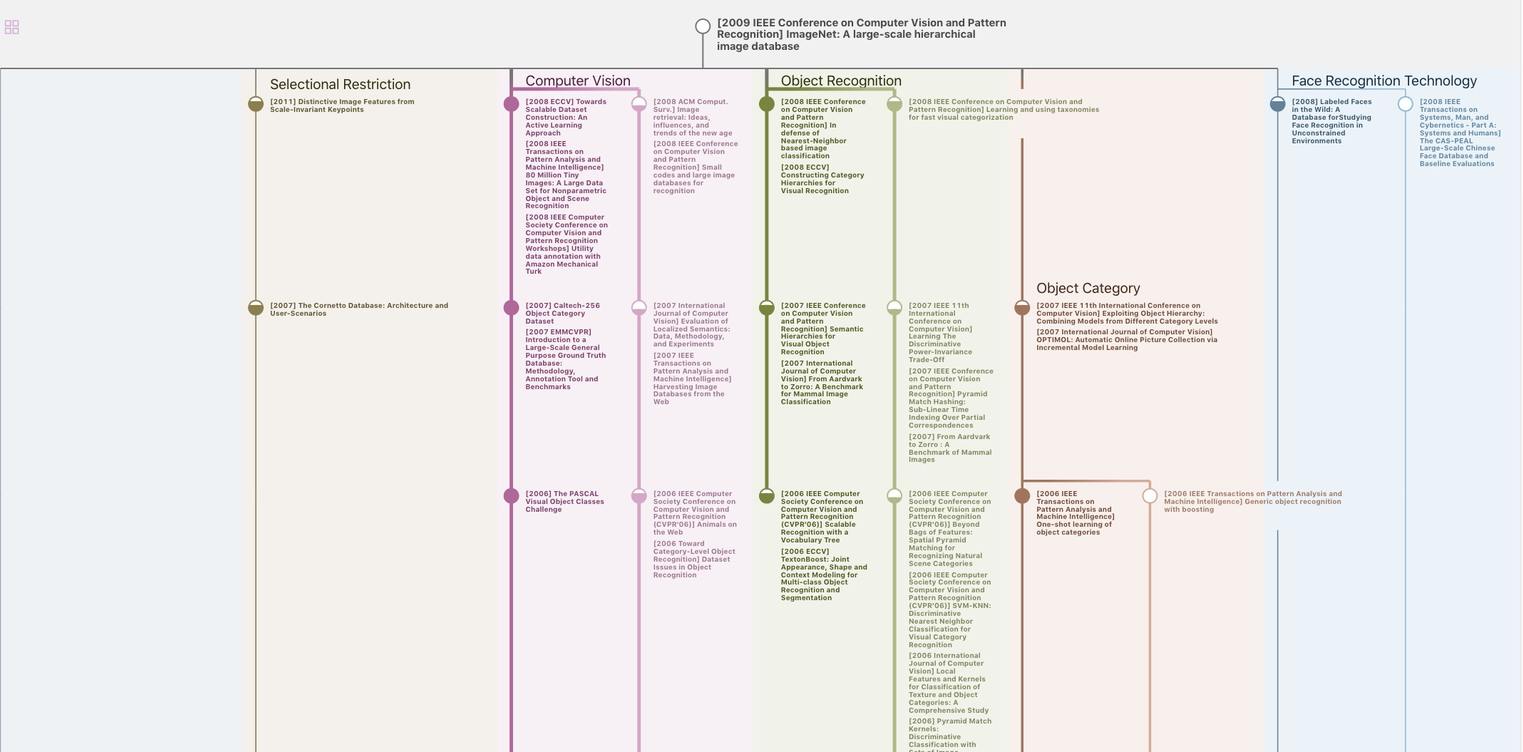
生成溯源树,研究论文发展脉络
Chat Paper
正在生成论文摘要