U-YOLOXs: An improved YOLOXs for Uncommon Unregular Unbalance detection of the rape subhealth regions.
Comput. Electron. Agric.(2022)
摘要
Agricultural production in high latitudes could be limited by cold climate. Plant factory allows continuous production all year round, where the detection of plant growth is one of the most important tasks. To achieve non-destructive detection of rape in our plant factory, a feasible approach is to automatically detect subhealth areas from the rape images. However, this task faces the following challenges: (1) Uncommon problem: the subhealth regions on rape are the domain-specific objects, so the mainstream transfer learning-based detector is unreliable; (2) Unregular problem: the subhealth regions are difficult to detect due to their variable shapes, sizes and colors. (3) Unbalance problem: localization and classification of subhealth regions each have low-/highquality bounding box unbalance and easy/hard sample unbalance. In this paper, a novel deep object detector based on the YOLOXs, called U3YOLOXs, is proposed for the detection of subhealth regions on rape at the bolting stage. Specifically, a domain-specific self-supervised pre-training strategy in the backbone is developed for the Uncommon problem; next, a coordinate attention mechanism in the multi-scale neck network is built for the Unregular problem; finally, the focal EIoU and the focal loss in the decoupled head are designed for the Unbalance problem. The experimental results show that the mAP of our U3YOLOXs is 94.38 % with a latency of 20.4 ms per image, which achieves an optimal accuracy-speed tradeoff. Compared to the YOLOXs it achieves a significant improvement of 9.27 % on mAP at the cost of only 3.55 % increase in latency. Experimental analysis further shows the effectiveness of each improvement, and the reliability of porting U3YOLOXs to edge devices for agricultural production.
更多查看译文
关键词
Subhealth detection,Hydroponic rape,U3YOLOXs model
AI 理解论文
溯源树
样例
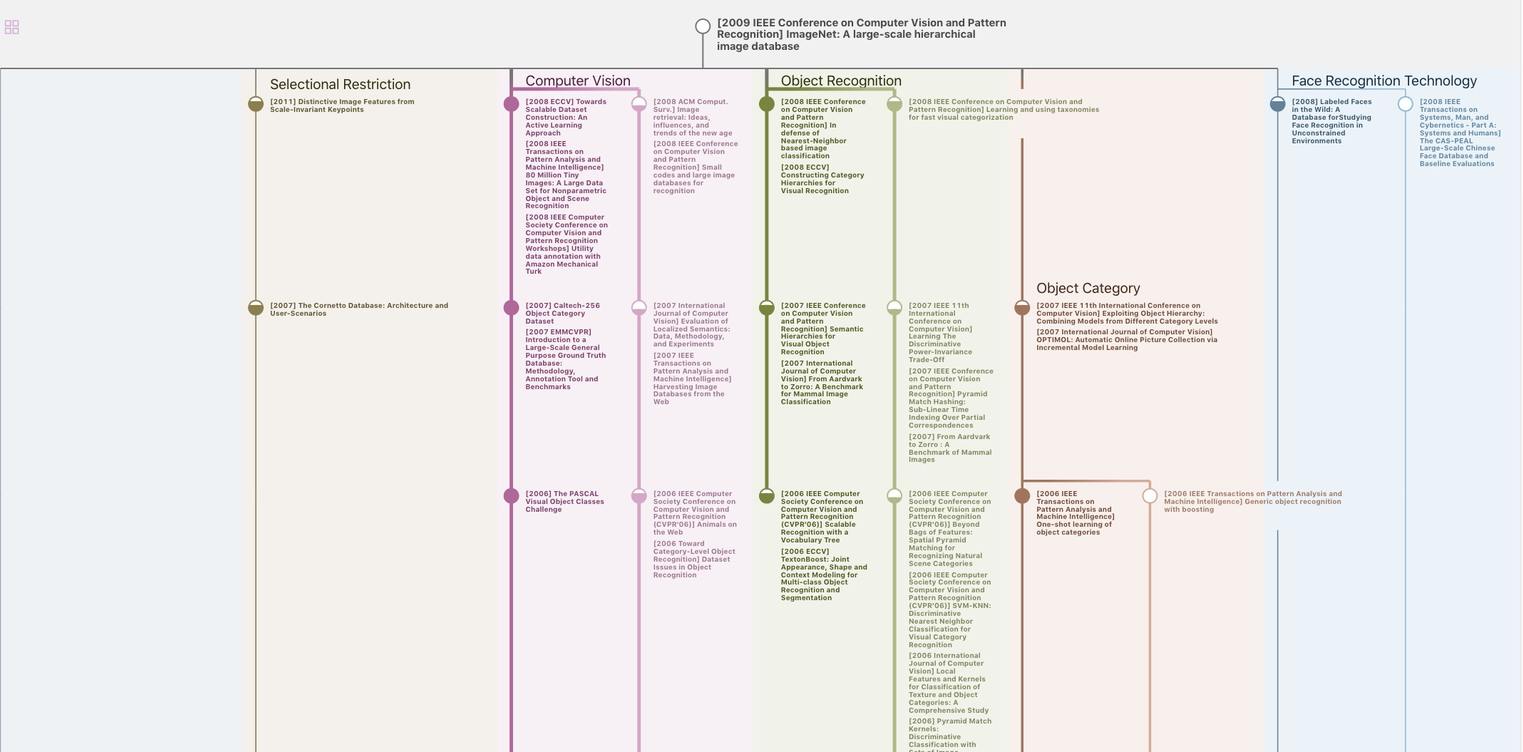
生成溯源树,研究论文发展脉络
Chat Paper
正在生成论文摘要