A novel deviation density peaks clustering algorithm and its applications of medical image segmentation.
IET Image Process.(2022)
摘要
The density peaks clustering (DPC) algorithm can identify clusters with various shapes and densities in the underlying dataset. However, the DPC algorithm cannot exactly find the true quantity of clustering centers when computing the local density, and it is difficult to handle non-convex datasets. Moreover, the DPC algorithm is difficult to identify boundary points and outliers without a reasonable allocation strategy when dealing with low-density points. To solve these limitations, a novel deviation density peaks clustering (DeDPC) algorithm is proposed. First, the local deviation of the spatial distance of datasets with different structures is utilised to replace the local density to generate a more reasonable clustering center decision graph. Second, a threshold is defined to further divide and process low-density points. Finally, outliers in low-density points can be accurately found to accurately cluster the dataset. To evaluate the performance of the DeDPC algorithm, experiments are conducted on synthetic and real-world datasets and the DeDPC is compared with other clustering methods. The DeDPC is also applied to medical image segmentation to further demonstrate its capability for medical image processing. The simulation results show that the DeDPC method has good validity and utility for both non-convex datasets and medical image segmentation.
更多查看译文
AI 理解论文
溯源树
样例
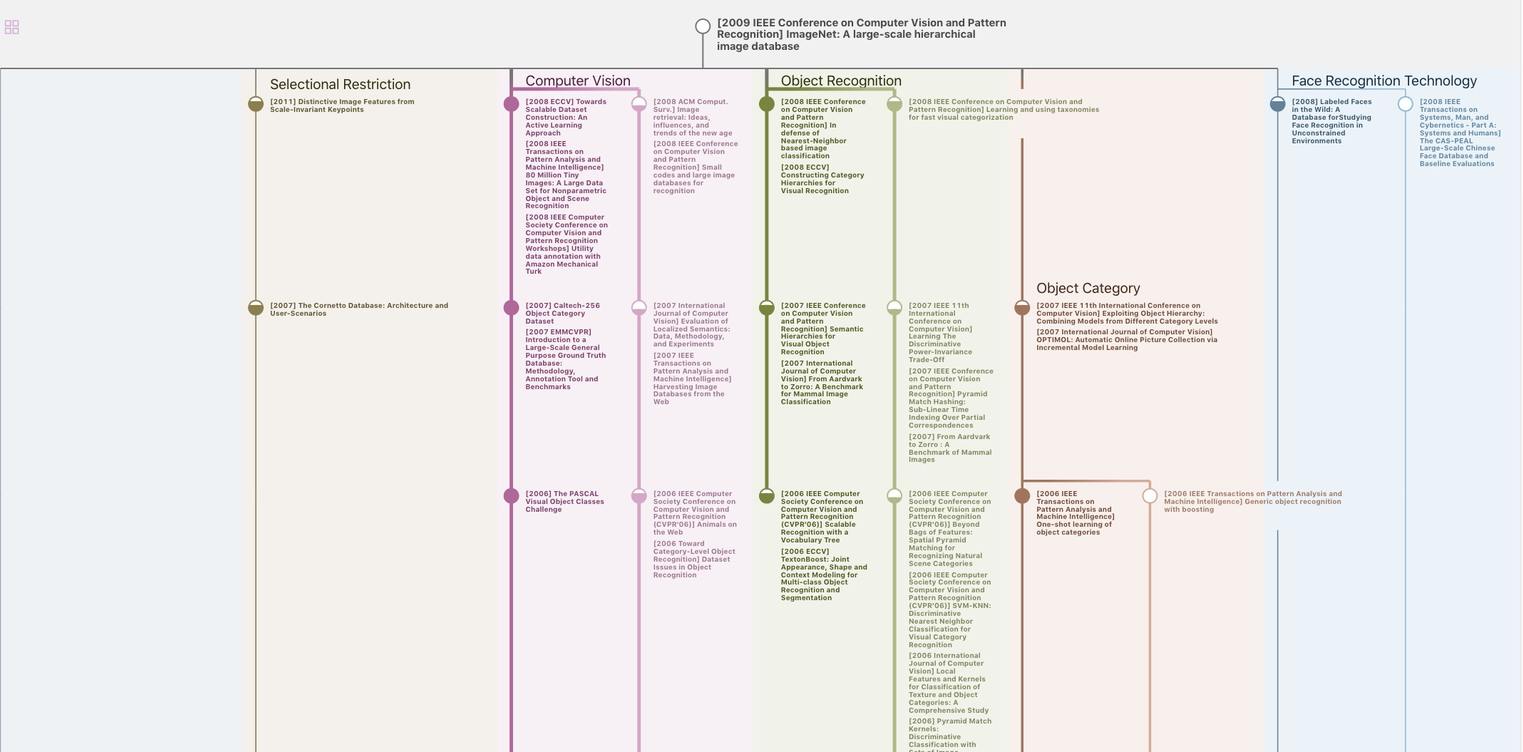
生成溯源树,研究论文发展脉络
Chat Paper
正在生成论文摘要