Rethinking Monocular Height Estimation from a Classification Task Perspective Leveraging the Vision Transformer
IEEE geoscience and remote sensing letters(2022)
摘要
Height estimation from a single remote sensing image has great potential in generating digital surface models (DSMs) efficiently for a quick Earth surface reconstruction. Recently, convolutional neural networks (CNNs) have emerged as a powerful method to deal with this ill-posed problem. Most existing methods formulate height estimation as a regression problem due to the continuity of object height. However, it is difficult for the model to regress the object heights exactly to the ground-truth values with a wide range. In this letter, we reformulate the height estimation task as a classification task to improve the model performance. Specifically, we discretize the continuous ground-truth height into bins and assign each pixel to a single label according to the bin subdivision. In addition, we propose to generate a unique bin subdivision for each input image adaptively by viewing bin generation as a set-to-set problem. Compared with the fixed bin subdivision method, a specific bin subdivision for each input image makes the model adaptively focus on the height range that is more probable to occur in the scene of the input image. In our experiments, we qualitatively and quantitatively demonstrate that the proposed method outperforms the state-of-the-art approaches on both the Vaihingen and Potsdam datasets.
更多查看译文
关键词
Digital surface models (DSMs),monocular height estimation,regression to classification
AI 理解论文
溯源树
样例
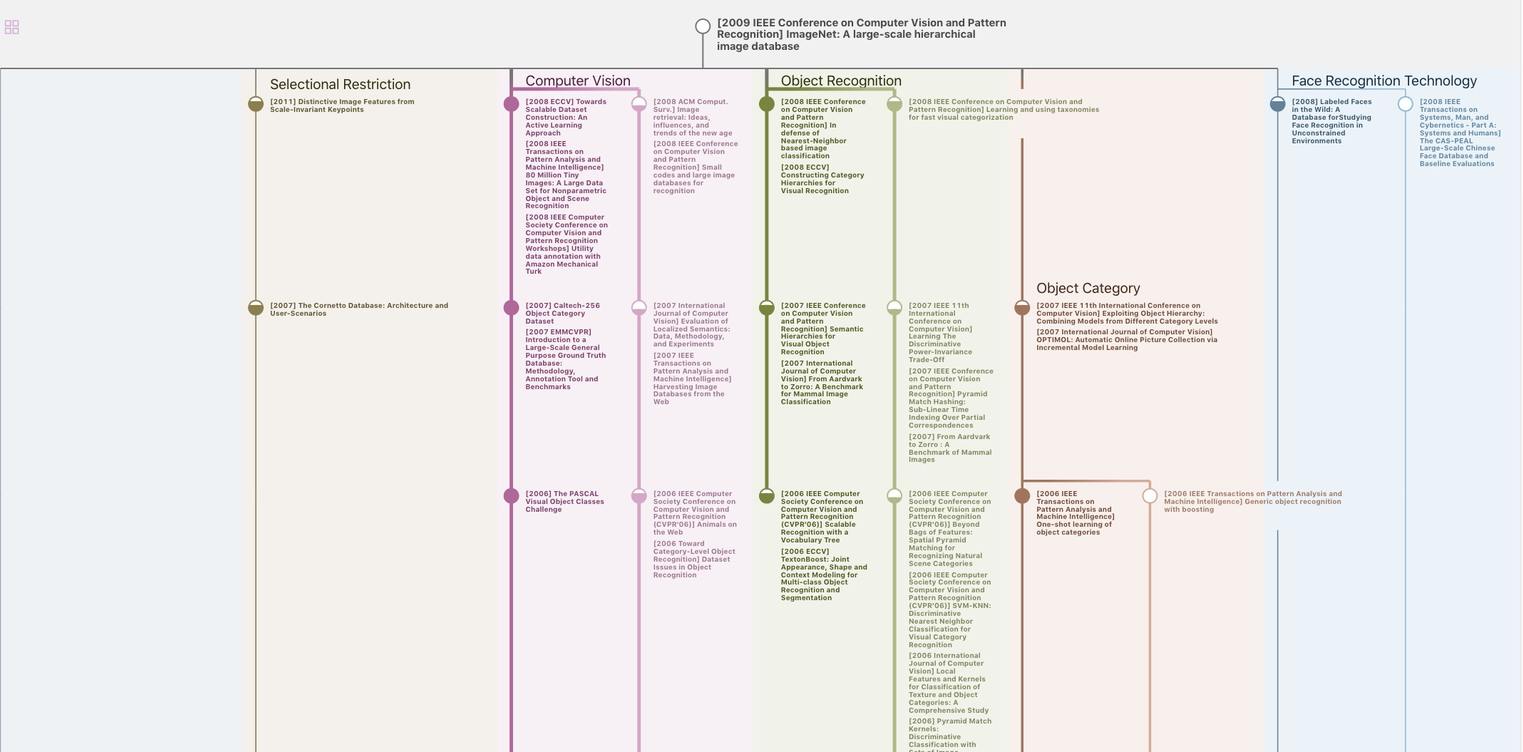
生成溯源树,研究论文发展脉络
Chat Paper
正在生成论文摘要