SymmetryGrasp: Symmetry-Aware Antipodal Grasp Detection From Single-View RGB-D Images.
IEEE Robotics Autom. Lett.(2022)
摘要
Symmetry is ubiquitous in everyday objects. Humans tend to grasp objects by recognizing the symmetric regions. In this letter, we investigate how symmetry could boost robotic grasp detection. To this end, we present a learning-based method for detecting grasp from single-view RGB-D images. The key insight is to explicitly incorporate symmetry estimation into grasp detection, improving the quality of the detected grasps. Specifically, we first introduce a new grasp parameterization in grasp detection for parallel grippers based on symmetry. Based on this representation, a symmetry-aware grasp detection network method is present to simultaneously estimate object symmetry and detect grasp. We find that the learning of grasp detection greatly benefits from symmetry estimation, improving the training efficiency and the grasp quality. Besides, to facilitate the cross-instance generality of grasping unseen objects, we propose Principal-directional scale-Invariant Feature Transformer (PIFT), a plug-and-play module, that allows spatial deformation of points during the feature aggregation. The module essentially learns feature invariance to anisotropic scaling along the shape principal directions. Extensive experiments demonstrate the effectiveness of the proposed method. In particular, it outperforms previous methods, achieving state-of-the-art performance in terms of grasp quality on GraspNet-1-Billion and success rate on a real robot grasping experiment.
更多查看译文
关键词
Grasping,Robots,Grippers,Three-dimensional displays,Feature extraction,Robot kinematics,Geometry,RGB-D perception,deep learning in grasping and manipulation,deep learning for visual perception
AI 理解论文
溯源树
样例
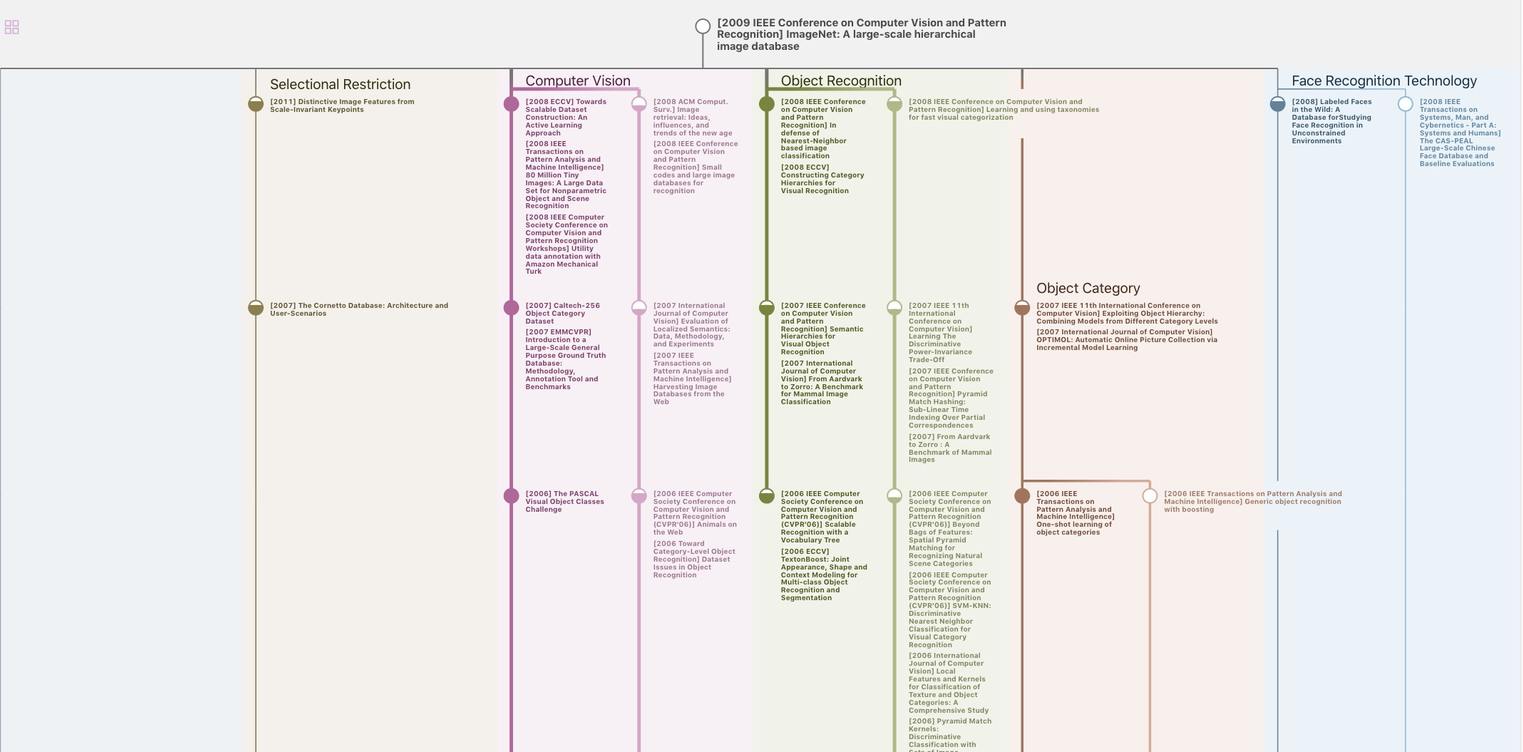
生成溯源树,研究论文发展脉络
Chat Paper
正在生成论文摘要