Research on Scattering Transform of Urban Sound Events Detection Based on Self-Attention Mechanism.
IEEE Access(2022)
摘要
Urban sound event detection can automatically preload relevant information for a robot to ensure that it can be applied to various scene-activity tasks. To address the limitations of timbre similarity and scene recognition by audio collection devices, a fusion model based on the self-attention mechanism is proposed in this paper. The model consists of scattering transform and self-attention model. The scattering transform computes modulation spectrum coefficients of multiple orders through cascades of wavelet convolutions and modulus operators. It is learnable compared with Mel-scale Frequency Cepstral Coefficients (MFCC), and can be used to better restore the semantic features of some sound scenes with similar timbres. The transformer has an outstanding effect on Natural Language Processing (NLP) owing to its self-attention mechanism. In this paper, the self-attention mechanism in its encoder was used in the model, mainly to make the feature granularity consistent to refine the features. In addition, Focal Loss function was adopted in the model to curb the sample distribution imbalance. The Google Command and ESC-50 were used to supplement the scene categories of dataset UrbanSound8K. The model parameters of the learnable filters that performed well on the dataset UrbanSound8K were preserved to fine-tune the other two datasets with insufficient data volume and more target categories. The length of slice duration was further explored the in the model. The experimental results show that the model can achieve better performance in a large range of scene models.
更多查看译文
关键词
Preload information,scattering transform,feature granularity consistency,self-attention mechanism,focal loss
AI 理解论文
溯源树
样例
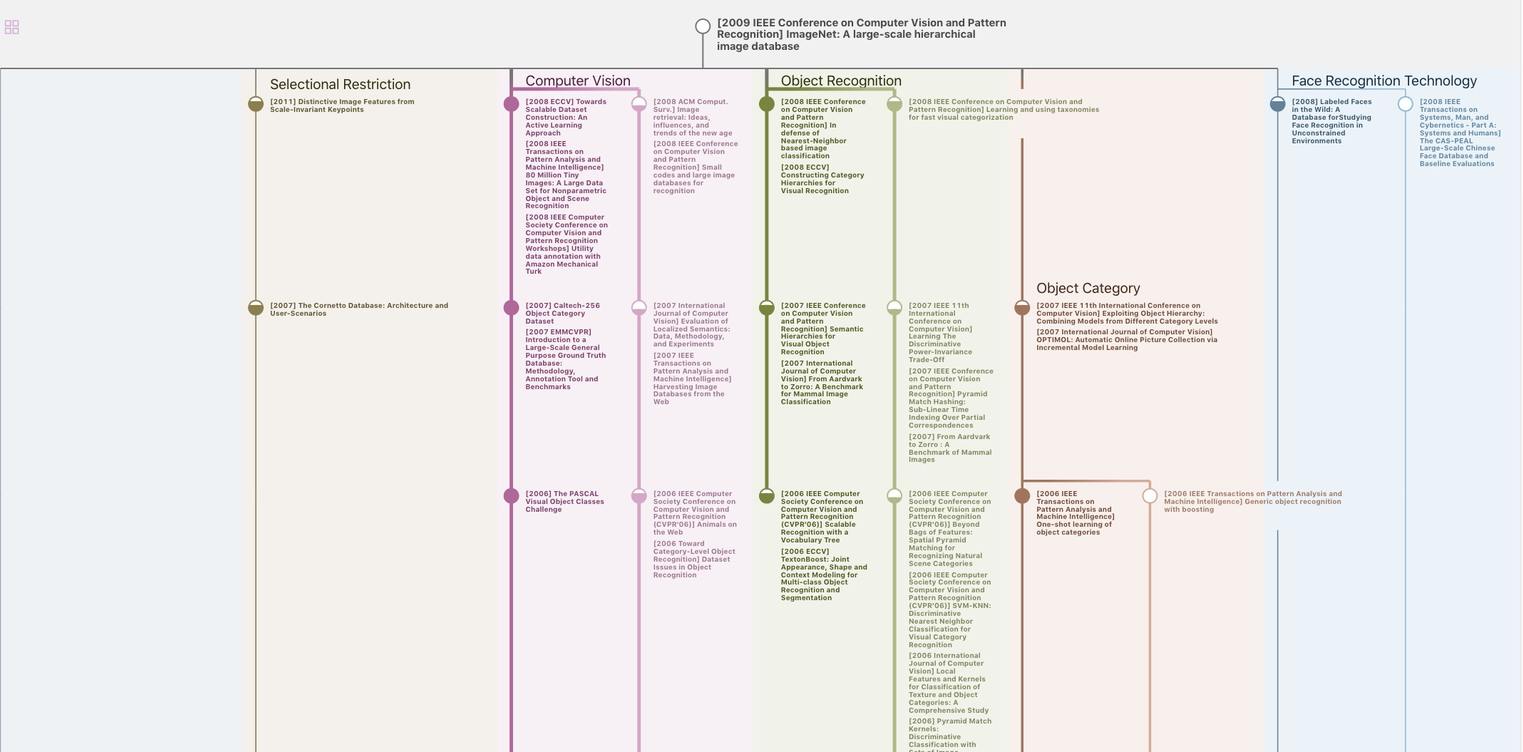
生成溯源树,研究论文发展脉络
Chat Paper
正在生成论文摘要