Pattern Recognition Based Auto-Reclosing Scheme Using Bi-Directional Long Short-Term Memory Network.
IEEE Access(2022)
摘要
Precise knowledge of secondary arc extinction instant and fault nature (temporary or permanent) is necessary for auto-reclosing after a single line-to-ground fault. Existing intelligent reclosing schemes rely on the extraction of appropriate features using a signal processing module (SPM) during online data monitoring. The value of features varied greatly under different operating scenarios as well as the computational burden is greatly enhanced owing to SPM which significantly impacts the performance of the auto-reclosing scheme. Hence, in this study bi-directional long short-term memory (Bi-LSTM) network is designed which integrates feature extraction and classification process. Thus, the proposed scheme is directly incorporated into the incoming voltage data without using any SPM/ filtering technique. The open-source test system provided by the developers of Hydro-Quebec, Canada is used for training and testing. Around 4860 different signals are collected by varying power system parameters and secondary arc conditions to develop dataset A. The Bi-LSTM model is tested under no noise, low noise of SNR 30, and high noise of SNR 10. To ensure the efficacy of the proposed scheme, uni-directional long short-term memory (U-LSTM), gated recurrent unit (GRU), and machine learning models are also trained on the same dataset. Later, for validation, a second dataset B is developed by varying surge impedance loading, frequency-dependent transmission lines, and arc resistance. Then the efficiency of pre-trained artificial neural networks (ANNs) is validated on this unseen dataset. The testing and validation on both datasets confirm superior efficiency of Bi-LSTM in comparison to U-LSTM, GRU, and other models.
更多查看译文
关键词
Artificial neural network (ANN) based reclosing,bi-directional long short-term memory (Bi-LSTM),patterns based reclosing,secondary arc,single-phase auto-reclosing
AI 理解论文
溯源树
样例
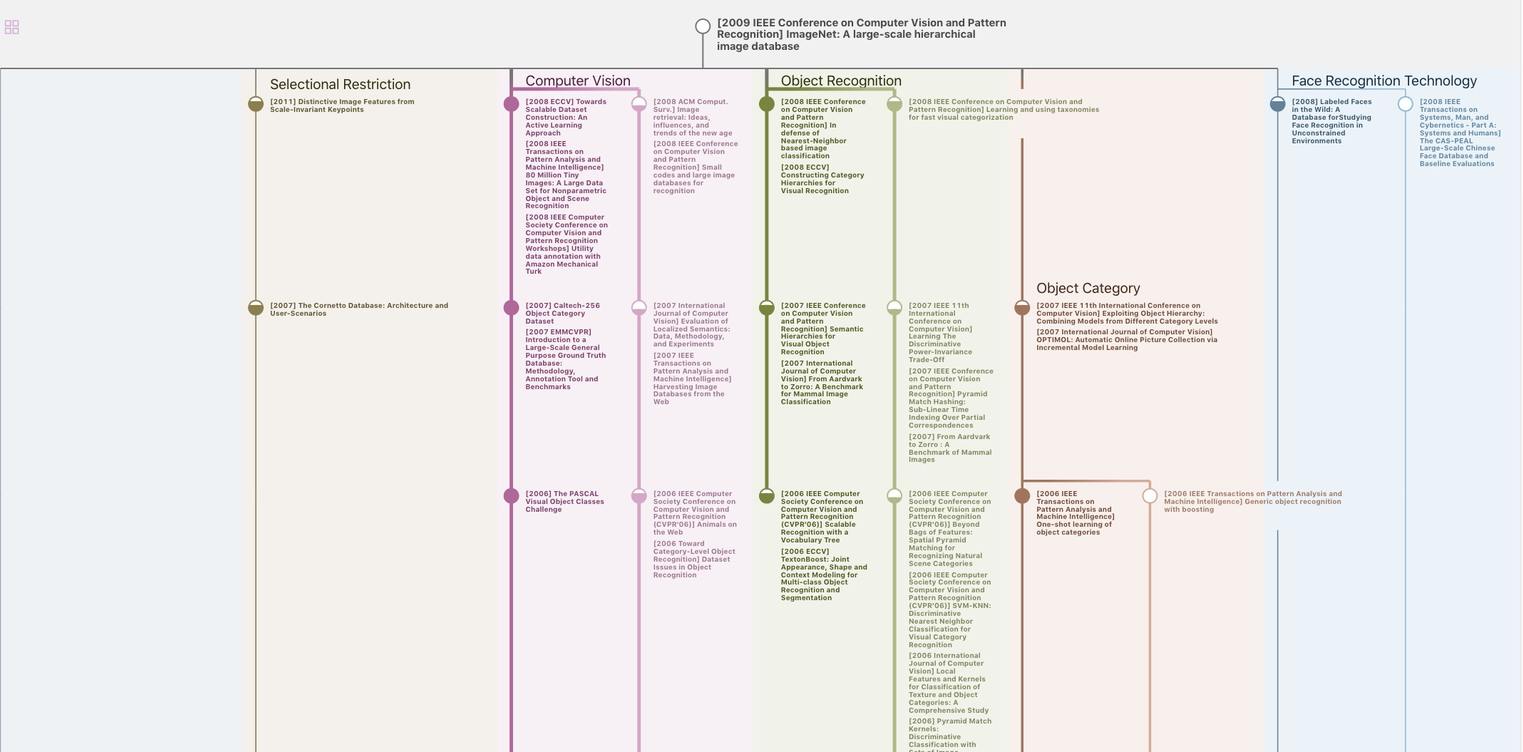
生成溯源树,研究论文发展脉络
Chat Paper
正在生成论文摘要