Root canal treatment planning by automatic tooth and root canal segmentation in dental CBCT with deep multi-task feature learning.
Medical image analysis(2023)
摘要
Accurate and automatic segmentation of individual tooth and root canal from cone-beam computed tomography (CBCT) images is an essential but challenging step for dental surgical planning. In this paper, we propose a novel framework, which consists of two neural networks, DentalNet and PulpNet, for efficient, precise, and fully automatic tooth instance segmentation and root canal segmentation from CBCT images. We first use the proposed DentalNet to achieve tooth instance segmentation and identification. Then, the region of interest (ROI) of the affected tooth is extracted and fed into the PulpNet to obtain precise segmentation of the pulp chamber and the root canal space. These two networks are trained by multi-task feature learning and evaluated on two clinical datasets respectively and achieve superior performances to several comparing methods. In addition, we incorporate our method into an efficient clinical workflow to improve the surgical planning process. In two clinical case studies, our workflow took only 2 min instead of 6 h to obtain the 3D model of tooth and root canal effectively for the surgical planning, resulting in satisfying outcomes in difficult root canal treatments.
更多查看译文
关键词
CBCT,Root canal,Segmentation,Surgical planning,Tooth
AI 理解论文
溯源树
样例
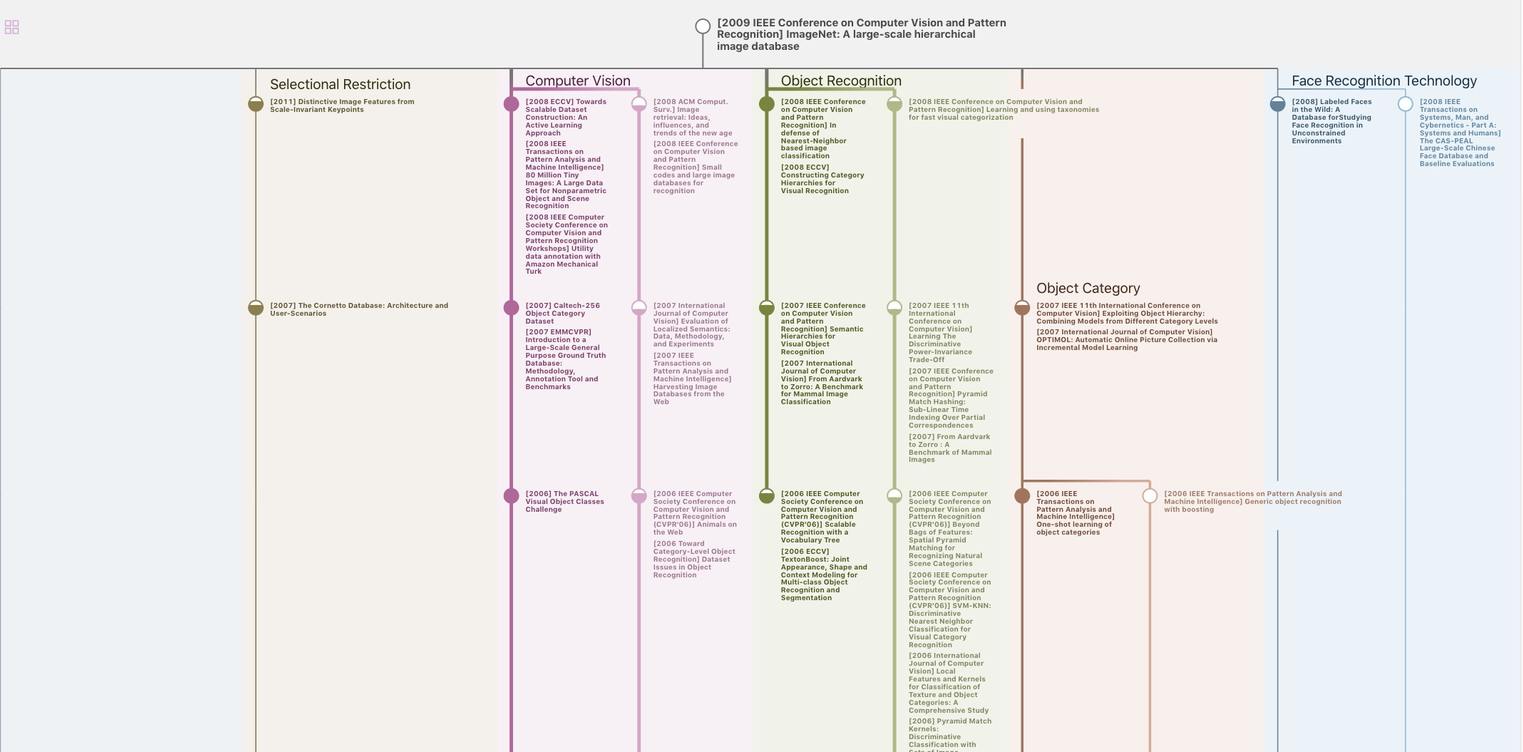
生成溯源树,研究论文发展脉络
Chat Paper
正在生成论文摘要