Pursuit of the Ultimate Regression Model for Samarium(III), Europium(III), and LiCl Using Laser-Induced Fluorescence, Design of Experiments, and a Genetic Algorithm for Feature Selection.
ACS omega(2023)
摘要
Laser-induced fluorescence spectroscopy, Raman scattering, and partial least squares regression models were optimized for the quantification of samarium (0-150 μg mL), europium (0-75 μg mL), and lithium chloride (0.1-12 M) with a transformational preprocessing strategy. Selecting combinations of preprocessing methods to optimize the prediction performance of regression models is frequently a major bottleneck for chemometric analysis. Here, we propose an optimization tool using an innovative combination of optimal experimental designs for selecting preprocessing transformation and a genetic algorithm (GA) for feature selection. A D-optimal design containing 26 samples (i.e., combinations of preprocessing strategies) and a user-defined design (576 samples) did not statistically lower the root mean square error of the prediction (RMSEP). The greatest improvement in prediction performance was achieved when a GA was used for feature selection. This feature selection greatly lowered RMSEP statistics by an average of 53%, resulting in the top models with percent RMSEP values of 0.91, 3.5, and 2.1% for Sm(III), Eu(III), and LiCl, respectively. These results indicate that preprocessing corrections (e.g., scatter, scaling, noise, and baseline) alone cannot realize the optimal regression model; feature selection is a more crucial aspect to consider. This unique approach provides a powerful tool for approaching the true optimum prediction performance and can be applied to numerous fields of spectroscopy and chemometrics to rapidly construct models.
更多查看译文
关键词
ultimate regression model,selection,genetic algorithm,fluorescence,laser-induced
AI 理解论文
溯源树
样例
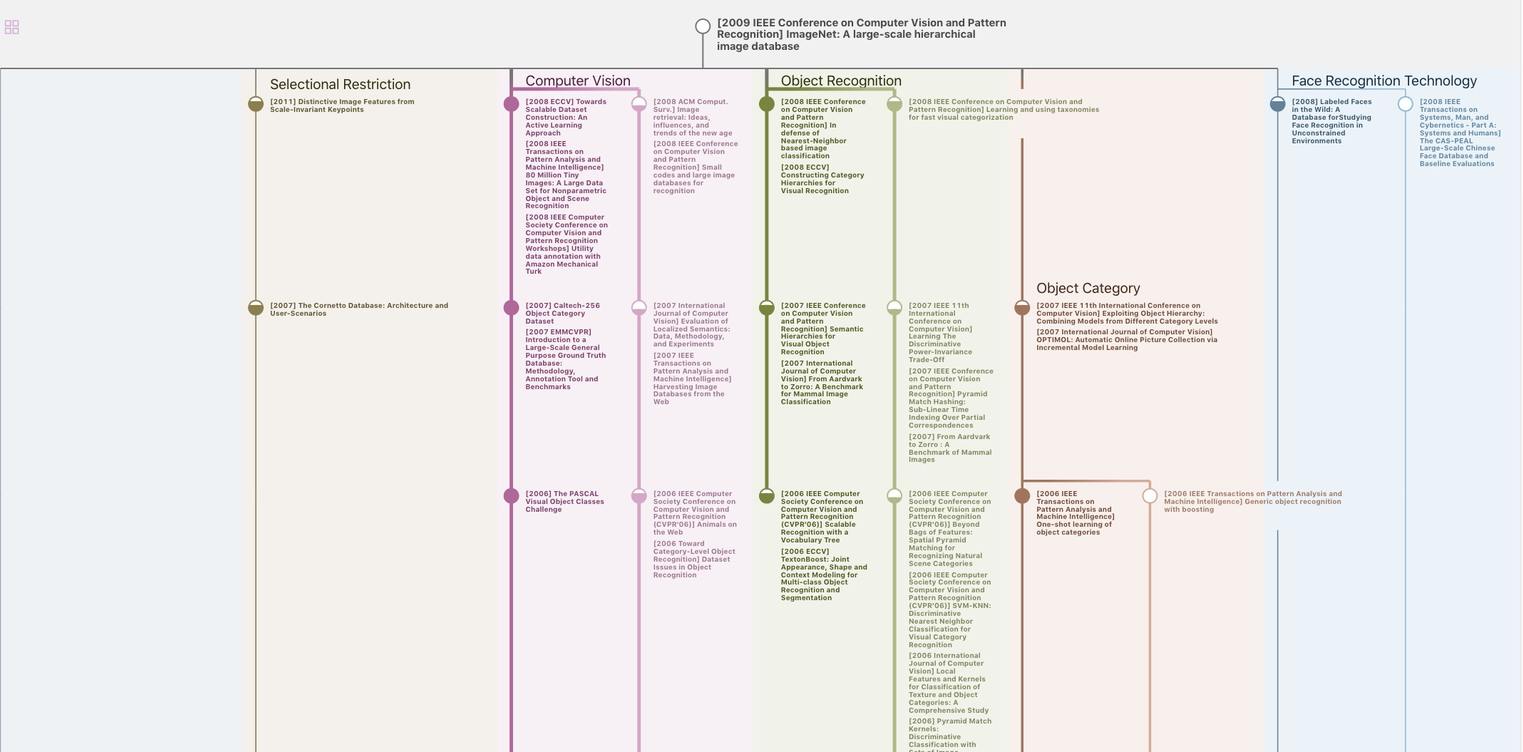
生成溯源树,研究论文发展脉络
Chat Paper
正在生成论文摘要