Inferred networks, machine learning, and health data.
PloS one(2023)
摘要
This paper presents a network science approach to investigate a health information dataset, the Sexual Acquisition and Transmission of HIV Cooperative Agreement Program (SATHCAP), to uncover hidden relationships that can be used to suggest targeted health interventions. From the data, four key target variables are chosen: HIV status, injecting drug use, homelessness, and insurance status. These target variables are converted to a graph format using four separate graph inference techniques: graphical lasso, Meinshausen Bühlmann (MB), k-Nearest Neighbors (kNN), and correlation thresholding (CT). The graphs are then clustered using four clustering methods: Louvain, Leiden, and NBR-Clust with VAT and integrity. Promising clusters are chosen using internal evaluation measures and are visualized and analyzed to identify marker attributes and key relationships. The kNN and CT inference methods are shown to give useful results when combined with NBR-Clust clustering. Examples of cluster analysis indicate that the methodology produces results that will be relevant to the public health community.
更多查看译文
关键词
health data,networks,machine learning
AI 理解论文
溯源树
样例
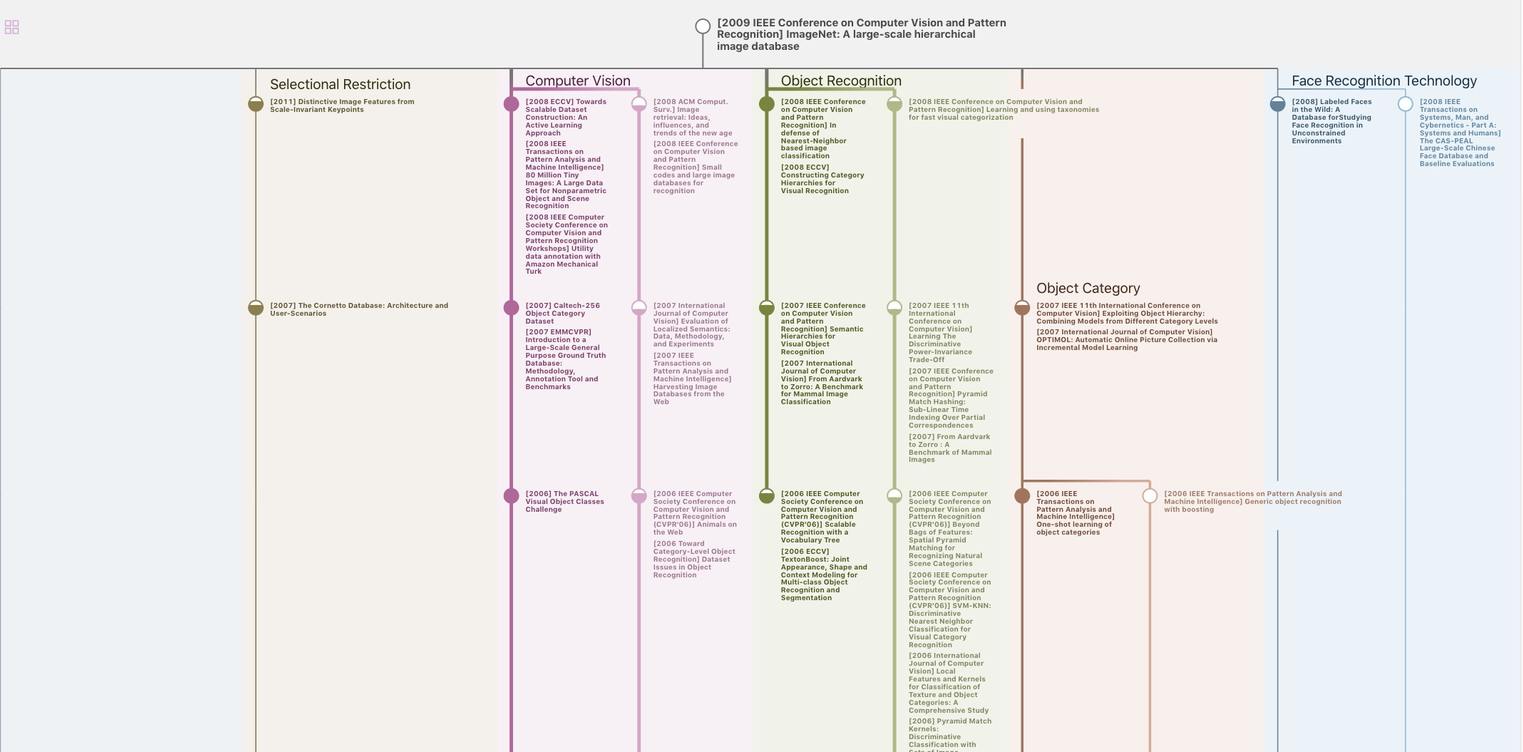
生成溯源树,研究论文发展脉络
Chat Paper
正在生成论文摘要