A Novel Deep Learning Model for End-to-End Characterization of Thin Cracking in SHCCs
RILEM Bookseries Strain Hardening Cementitious Composites(2023)
摘要
Strain hardening cementitious composites (SHCCs), can be designed to exhibit small crack widths. As a result, even after the material is cracked it restricts the flow of deleterious material. This property among many other properties makes it useful to design durable infrastructures. Assessment of durability of SHCCs requires measurements of surface cracks. The process of development of an SHCC mix for any application thus involves the characterization of cracks to understand its durability component at different strain values. Conventionally, this can be done by using digital cameras to document the images of SHCC surfaces during testing and manually analyzing these images to compute different crack characteristics such as width. This process is laborious and time-consuming thus, non-scalable if the number of examples is large. In this work, we designed a novel deep learning model for this purpose called Strain Hardening Segmentation Network (SHSnet). SHSnet has a very high accuracy of 85% while requiring ~ 4M parameters which is one order less than other state-of-the-art networks like U-net. Due to the inherent thinness of the cracks in SHCCs, the amount of examples for training is fairly low (<1%). To this end, we proposed a loss function (PLF) to train the network efficiently. With this, it takes at least 10x less time for computing crack parameters. We further show applications of the SHSnet empowered technique for durability assessment and detecting crack evolution. These results suggest that SHSnet has the potential to facilitate the autonomous characterization of SHCC cracks in many scenarios.
更多查看译文
关键词
thin cracking,novel deep learning model,deep learning,end-to-end
AI 理解论文
溯源树
样例
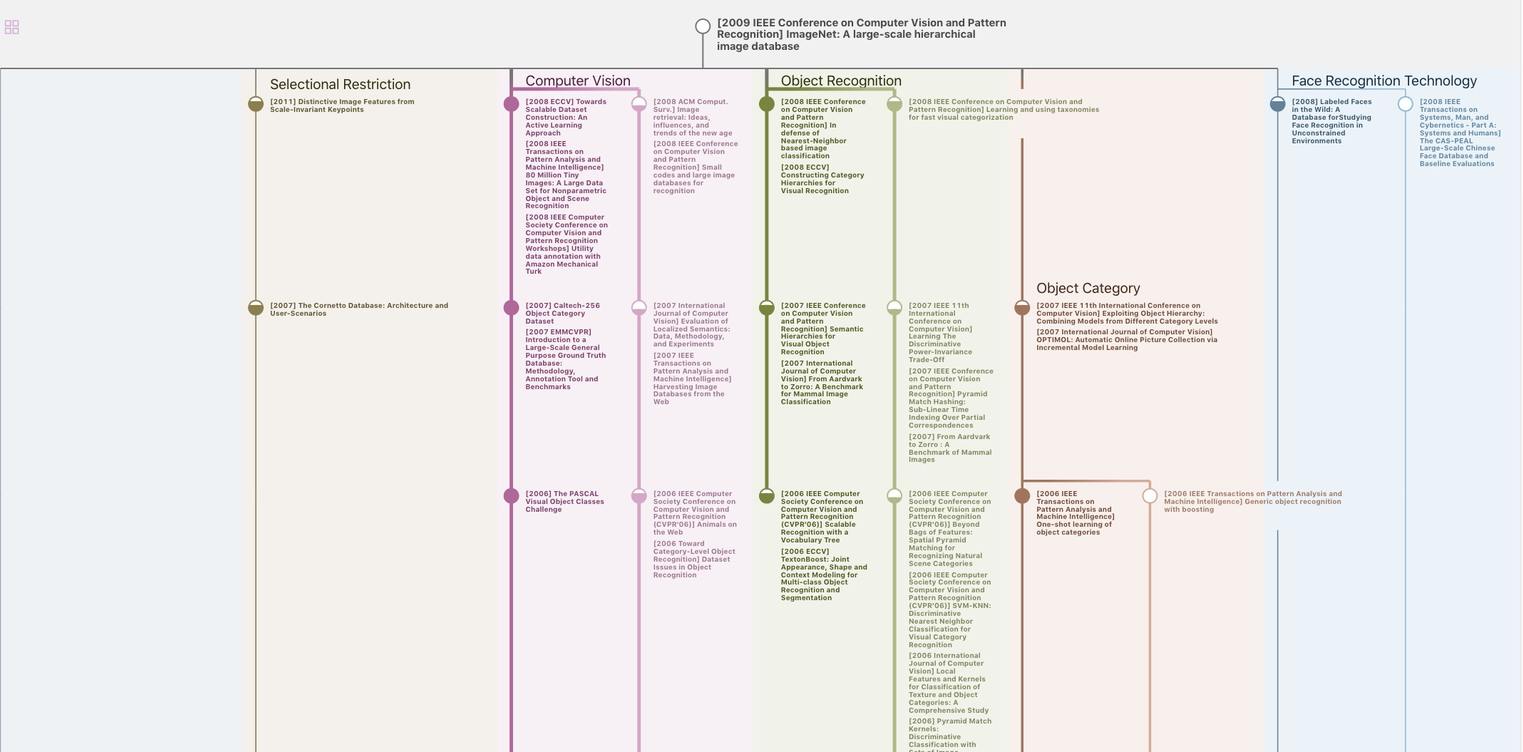
生成溯源树,研究论文发展脉络
Chat Paper
正在生成论文摘要