Feature Correlated Auto Encoder Method for Industrial 4.0 Process Inspection Using Computer Vision and Machine Learning
Procedia Computer Science(2023)
摘要
From the perspective of the Industry 4.0 paradigm, the machine learning (ML) discipline has had a significant influence on the manufacturing sector. The industry 4.0 concept promotes intelligent sensors, gadgets, and equipment to create technology infrastructure sectors that collect information constantly. By analyzing the obtained data, machine learning approaches allow actionable insight to boost industrial productivity without dramatically altering the necessary resources. Furthermore, the capacity of machine learning applications to provide actionable analytics has facilitated the detection of complex manufacturing trends and paved the path for an integrated intelligent process in various activities in the supply chain, including smart and constant inspection, preventative maintenance, quality enhancement, process optimization, supply chain advancement, and workflow scheduling. This paper aims to present recent advances in the field of quality inspection in Industry 4.0 and develop a framework for quality inspection that can be fully utilized in the Industry 4.0 context using adaptive bilateral filtering and Feature Correlated Auto encoder (FCA) machine learning technique. The suggested approach makes full use of information from all sources along the manufacturing chain. Therefore, it complies with quality management standards within the context of Industry 4.0. The suggested model makes use of corrective measures based on data patterns discovered through predictive analysis. Result analysis was shown on some pre-trained deep learning models such as ResNet18, Vgg19, Alexnet, Squeezenet, auto encoder, and FCA and observed that the proposed FCA(Feature Correlated Auto encoder) achieved a better result.
更多查看译文
关键词
Manufacturing,Industry 4.0,Machine Learning,Computer Vision,Image Processing
AI 理解论文
溯源树
样例
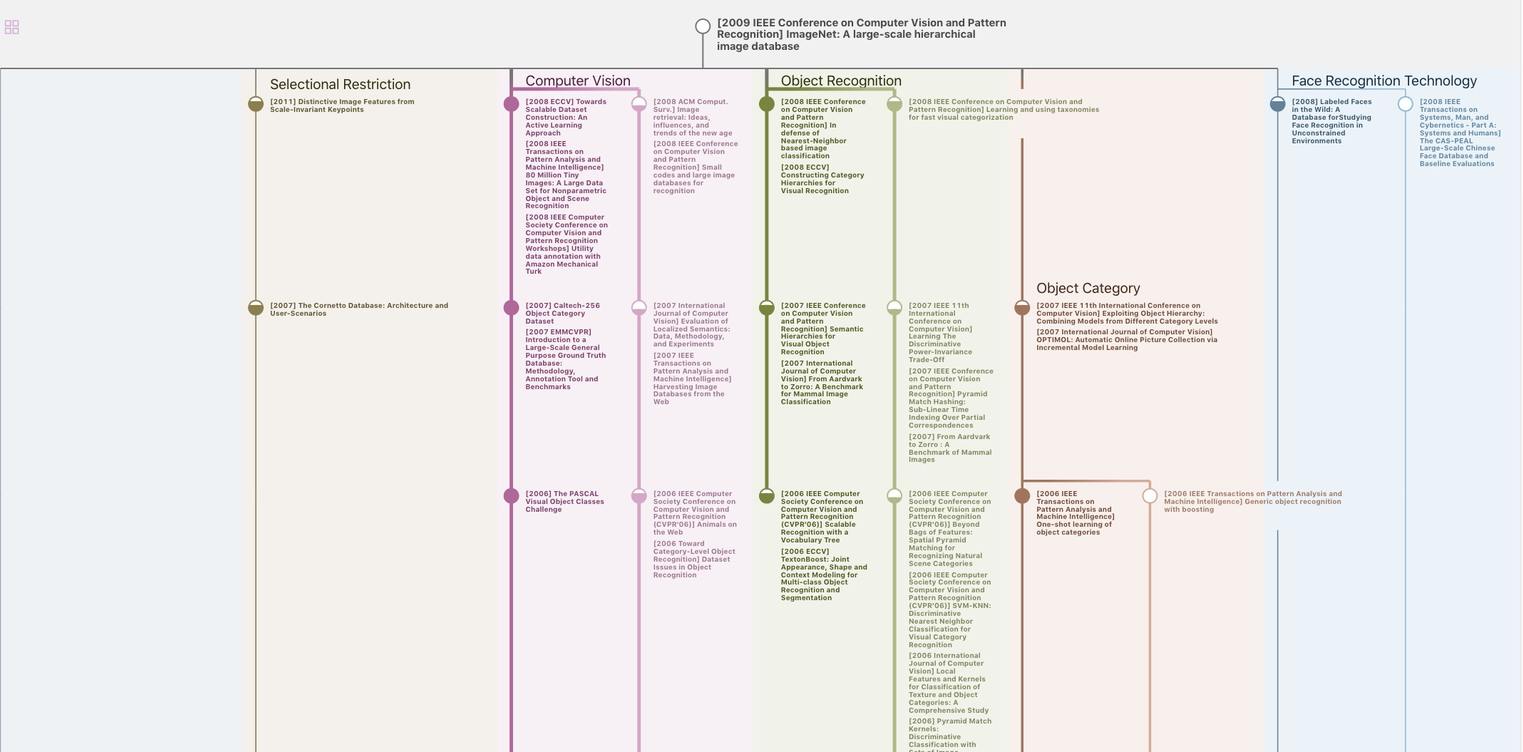
生成溯源树,研究论文发展脉络
Chat Paper
正在生成论文摘要