Towards end-to-end formation control for robotic fish via deep reinforcement learning with non-expert imitation
Ocean Engineering(2023)
摘要
Collaboration of multiple robotic fish can accomplish various underwater tasks effectively. However, controlling robotic fish to maintain a specific formation remains a huge challenge, especially in complex and changing flow fields. This paper presents an end-to-end formation control approach in the leader–follower topology by combining deep reinforcement learning and imitation learning. First, we build a high-fidelity environment based on computational fluid dynamics (CFD) to generate samples for training the formation controller. In this environment, we maneuver the robotic fish by adjusting the maximum swing of its tail. Then, we model the formation control problem as a Markov decision process (MDP), where a compound reward function is tailored to guide the training. To improve the learning efficiency of the deep reinforcement learning (DRL) based controller, we propose a novel DRL algorithm on the top of deep Q-networks (DQN) and behavior cloning, which we call dueling double DQN (D3QN) with imitation. Combining with the designed imitation-based action selection strategy, this algorithm significantly reduce the blindness of agent exploration at the beginning of training. A series of experiments demonstrate the advantages of the proposed algorithm in terms of control accuracy, training efficiency, as well as generalization ability for different formation configurations.
更多查看译文
关键词
Deep reinforcement learning,Formation control,Robotic fish,Imitation learning
AI 理解论文
溯源树
样例
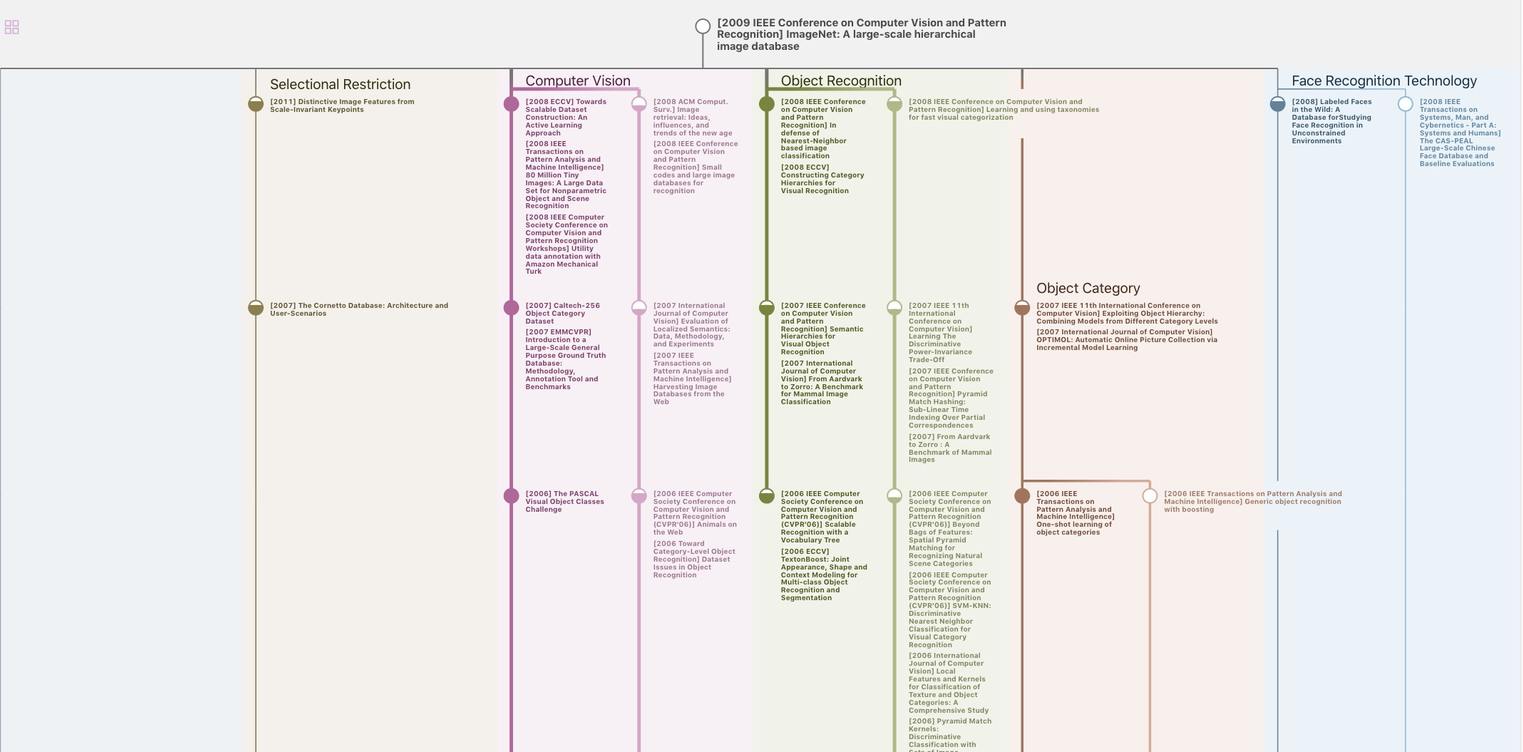
生成溯源树,研究论文发展脉络
Chat Paper
正在生成论文摘要