Algorithm Selection across Algorithm Configurators A Case Study on Multi-objective Optimization
2022 IEEE Symposium Series on Computational Intelligence (SSCI)(2022)
摘要
The present work utilizes Algorithm Selection for automatically specifying the parameter tuning method for a given tuning task. The idea of parameter tuning is motivated by the premature algorithm designs and their sub-optimal or poor parameter value choices. The decisions both on the designs and the parameter values are largely given based on the experiences of the developers or the target problem domain experts after a limited number of trials. While the existing tuning approaches tend to offer improvements over the default parameter values for varying algorithms, they can be computationally expensive. Additionally, there is no a single, ultimate parameter tuning strategy. These facts suggest to choose the most effective tuning algorithm for a specific scenario. This study utilizes an existing Algorithm Selection system to address this problem. The idea is to allocate potentially the most effective tuning method for a given task instead of relying on a single tuner. On that note, a group of well-known parameter configuration approaches are accommodated as the candidate methods to tune NSGA-II for solving a suite of multi-objective optimization benchmarks, refer-encing a recent article. The computational analysis revealed that Algorithm Selection outperforms those constituent parameter tuning methods when each is used as a standalone manner. Additionally, the dis/-similarity analysis carried on the problem instances / benchmarks give hints on the diversity level of the benchmarks. Furthermore, a similar inspection reported on the parameter tuning procedures show the behavioural resemblance between them.
更多查看译文
关键词
algorithm selection,algorithm configuration,parameter tuning,multi-objective optimization
AI 理解论文
溯源树
样例
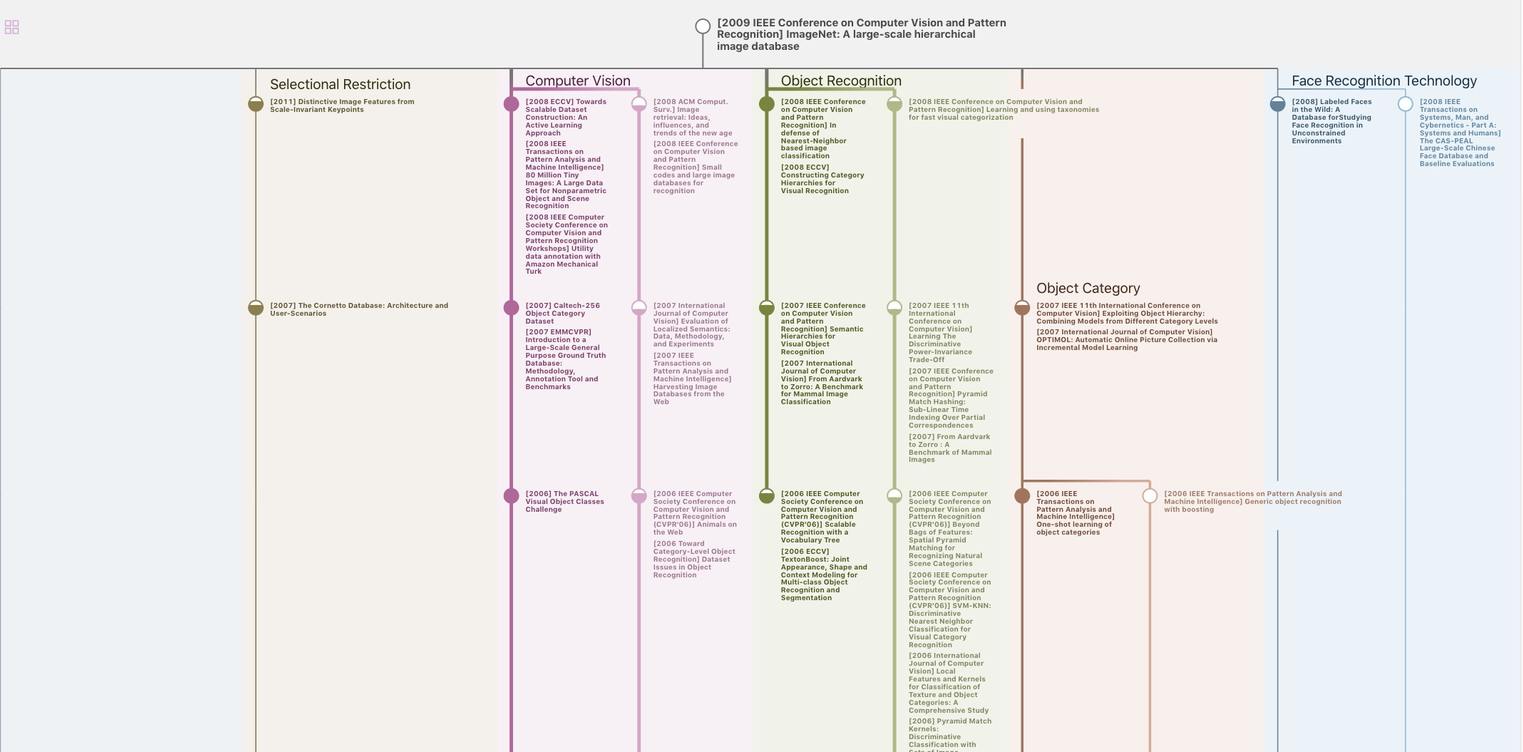
生成溯源树,研究论文发展脉络
Chat Paper
正在生成论文摘要