Online Adaptive CNN: a Session-to-session Transfer Learning Approach for Non-stationary EEG
2022 IEEE Symposium Series on Computational Intelligence (SSCI)(2022)
摘要
The convolutional neural network (CNN) automatically learns EEG representations in higher and nonlinear space via backpropagation and outputs the predictions in an end-to-end manner. Owing to these advantages, CNN has been used to decode electroencephalogram (EEG) and drive brain computer interface (BCI). However, its applications in BCI-assisted post-stroke neurorehabilitation remain limited for it is unable to address the inherent session-to-session non-stationarity in the EEG between the initial calibration session and subsequent online sessions. In this paper, we present a simple but effective online adaptive CNN (aCNN) to address the non-stationarity in multi-session EEG by progressively updating the subject-specific model. The performance of the proposed aCNN is evaluated on two neurorehabilitation datasets with a large population of post-stroke patients (33 patients with a total of 358 EEG sessions). Results indicate that, our proposed aCNN reaches at least as good a performance as the widely used online adaptive Filter Bank Common Spatial Patterns (aFBCSP) and with significantly higher accuracies than that for DeepConv and offline FBCSP algorithms. Our results support, for the first time, the use of a CNN-based adaptive learning method to decode non-stationary EEG signals for BCI-assisted post-stroke rehabilitation.
更多查看译文
关键词
online adaptive CNN,brain computer interface,non-stationarity,neurorehabilitation
AI 理解论文
溯源树
样例
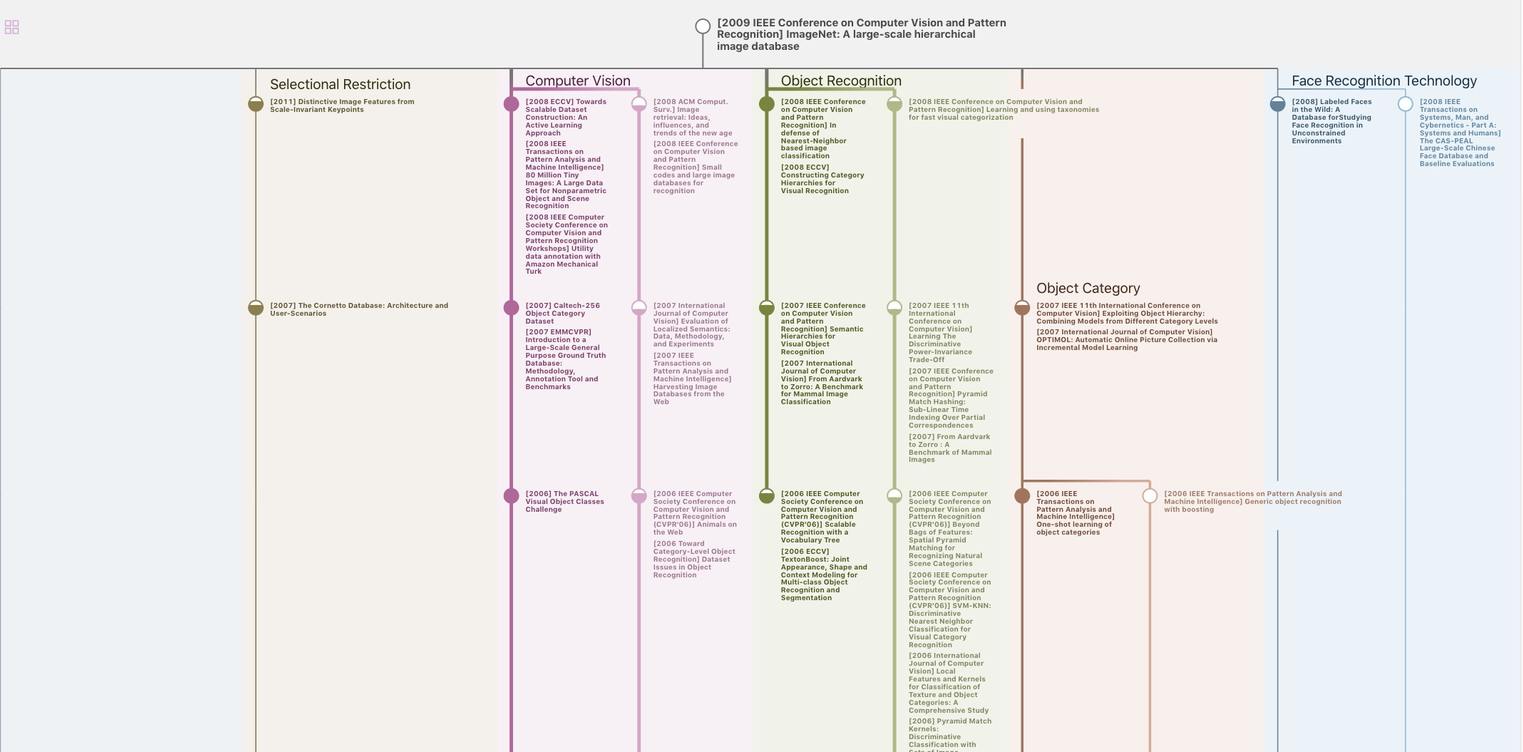
生成溯源树,研究论文发展脉络
Chat Paper
正在生成论文摘要