Semantic Adversarial Attacks on Face Recognition Through Significant Attributes
International Journal of Computational Intelligence Systems(2023)
摘要
Face recognition systems are susceptible to adversarial attacks, where adversarial facial images are generated without awareness of the intrinsic attributes of the images in existing works. They change only a single attribute indiscriminately. To this end, we propose a new Semantic Adversarial Attack using StarGAN (SAA-StarGAN), which manipulates the facial attributes that are significant for each image. Specifically, we apply the cosine similarity or probability score to predict the most significant attributes. In the probability score method, we train the face verification model to perform an attribute prediction task to get a class probability score for each attribute. Then, we calculate the degree of change in the probability value in an image before and after altering the attribute. Therefore, we perform the prediction process and then alter either one or more of the most significant facial attributes under white-box or black-box settings. Experimental results illustrate that SAA-StarGAN outperforms transformation-based, gradient-based, stealthy-based, and patch-based attacks under impersonation and dodging attacks. Besides, our method achieves high attack success rates on various models in the black-box setting. In the end, the experiments confirm that the prediction of the most important attributes significantly impacts the success of adversarial attacks in both white-box and black-box settings and could improve the transferability of the generated adversarial examples.
更多查看译文
关键词
Adversarial examples,Image-to-image translation,Face verification,Feature fusion,Black-box attack,Attack transferability
AI 理解论文
溯源树
样例
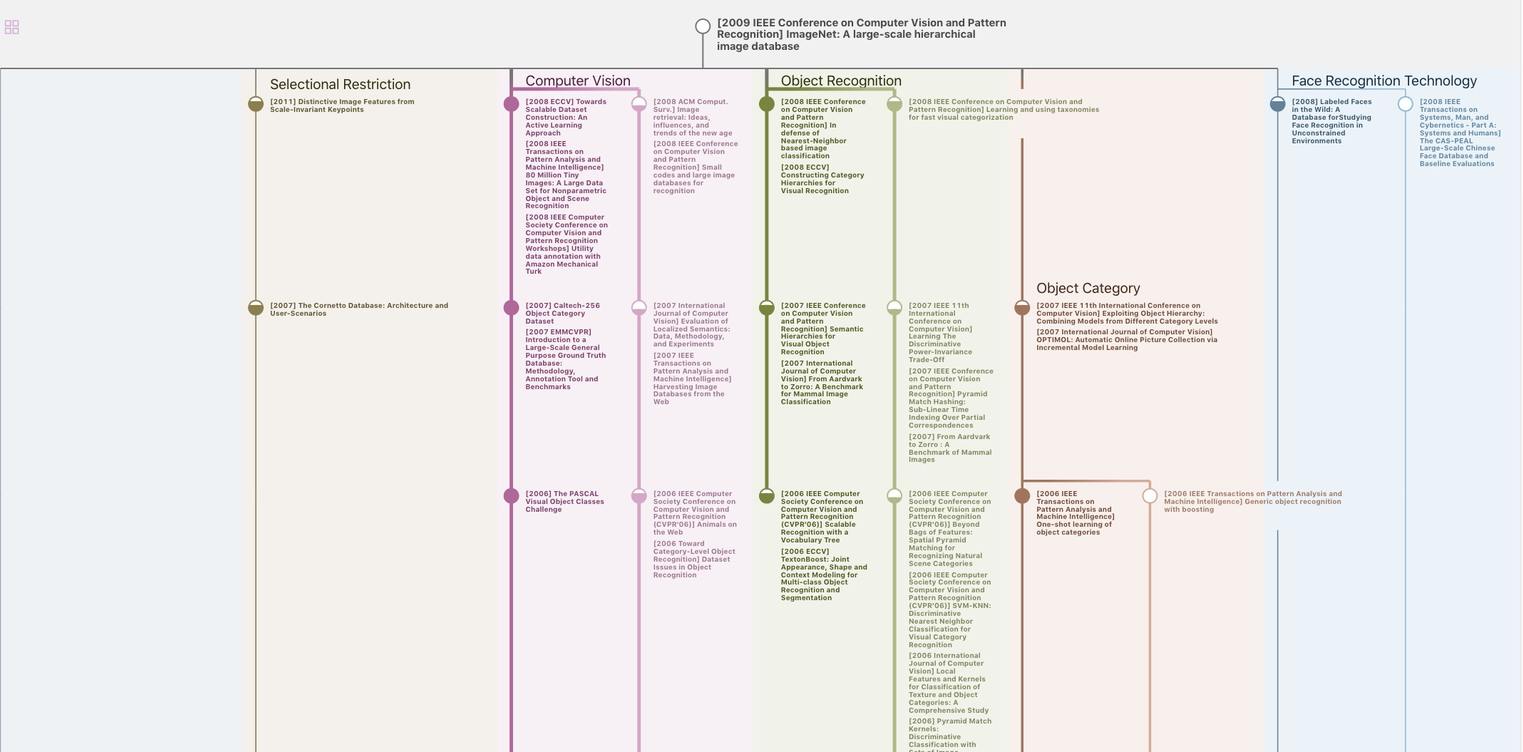
生成溯源树,研究论文发展脉络
Chat Paper
正在生成论文摘要