Potential energy surface prediction of Alumina polymorphs using graph neural network
arxiv(2023)
摘要
The process of design and discovery of new materials can be significantly expedited and simplified if we can learn effectively from available data. Deep learning (DL) approaches have recently received a lot of interest for their ability to speed up the design of novel materials by predicting material properties with precision close to experiments and ab-initio calculations. The application of deep learning to predict materials properties measured by experiments are valuable yet challenging due to the limited amount of experimental data. Most of the existing approaches to predict properties from computational data have also been directed towards specific material properties. In this work, we extend this approach, by proposing Landscape Crystal Graph Convolution Network(LCGCN), an accurate and transferable deep learning framework based on graph convolutional networks. LCGCN directly learns the potential energy surface (PES) from atomic configurations. This approach can enable transferable models that can predict different material properties. We apply this framework to bulk crystals (i.e. Al2O3), and test it by calculating potential energy surfaces at different temperatures and across different phases of crystal.
更多查看译文
关键词
alumina polymorphs,potential energy surface prediction,neural network
AI 理解论文
溯源树
样例
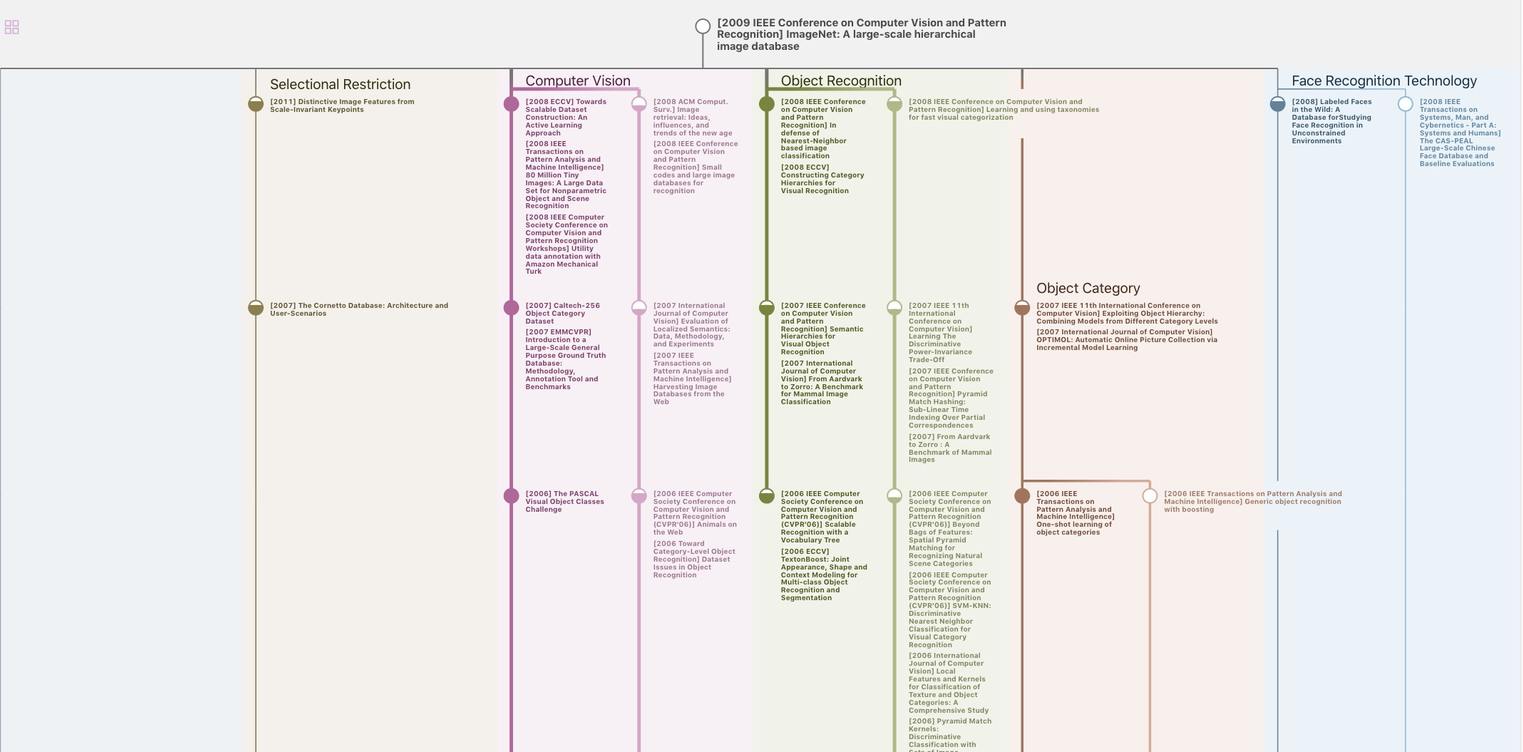
生成溯源树,研究论文发展脉络
Chat Paper
正在生成论文摘要