Optimizing a Bayesian method for estimating the Hurst exponent in behavioral sciences
arxiv(2023)
摘要
The Bayesian Hurst-Kolmogorov (HK) method estimates the Hurst exponent of a time series more accurately than the age-old detrended fluctuation analysis (DFA), especially when the time series is short. However, this advantage comes at the cost of computation time. The computation time increases exponentially with $N$, easily exceeding several hours for $N = 1024$, limiting the utility of the HK method in real-time paradigms, such as biofeedback and brain-computer interfaces. To address this issue, we have provided data on the estimation accuracy of $H$ for synthetic time series as a function of \textit{a priori} known values of $H$, the time series length, and the simulated sample size from the posterior distribution -- a critical step in the Bayesian estimation method. The simulated sample from the posterior distribution as small as $n = 25$ suffices to estimate $H$ with reasonable accuracy for a time series as short as $256$ measurements. Using a larger simulated sample from the posterior distribution -- i.e., $n > 50$ -- provides only marginal gain in accuracy, which might not be worth trading off with computational efficiency. We suggest balancing the simulated sample size from the posterior distribution of $H$ with the computational resources available to the user, preferring a minimum of $n = 50$ and opting for larger sample sizes based on time and resource constraints
更多查看译文
关键词
hurst exponent,bayesian method
AI 理解论文
溯源树
样例
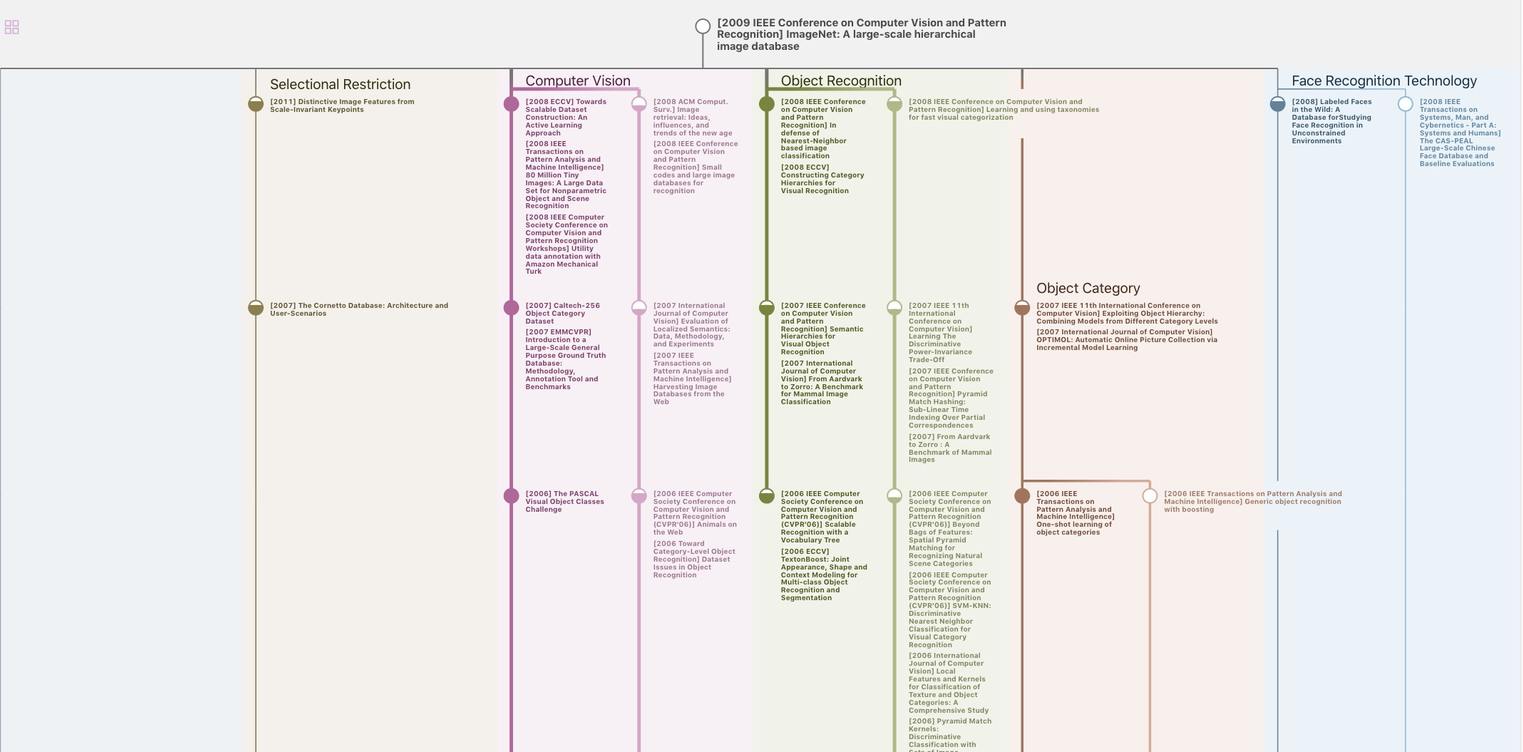
生成溯源树,研究论文发展脉络
Chat Paper
正在生成论文摘要