Semantics-enhanced Temporal Graph Networks for Content Caching and Energy Saving
ICC 2023 - IEEE INTERNATIONAL CONFERENCE ON COMMUNICATIONS(2023)
摘要
The enormous amount of network equipment and users implies a tremendous growth of Internet traffic for multimedia services. To mitigate the traffic pressure, architectures with in-network storage have been proposed to cache popular content at devices in close proximity to users in order to decrease the number of backhaul hops. Meanwhile, the reduced transmission distance also contributes to energy saving. However, due to limited storage, only a fraction of the content can be cached, while caching the most popular content is cost-effective. Correspondingly, it becomes essential to devise an effective popularity prediction method. In this regard, some existing efforts manifest the effectiveness of dynamic graph neural network (DGNN) models, but it remains challenging to tackle sparse datasets. Herein, we first propose a reformative temporal graph network, named STGN, to address the challenge and improve prediction performance. Specifically, the STGN model leverages extra semantic messages to help establish implicit paths within the sparse interaction graph and enhance the temporal and structural learning of a DGNN model. Furthermore, we devise a user-specific attention mechanism to aggregate various semantics in a fine-grained manner. Finally, extensive simulations verify the superiority of our STGN models and demonstrate the potential in terms of energy-saving.
更多查看译文
关键词
Content caching,popularity prediction,dynamic graph neural network,semantics,energy saving
AI 理解论文
溯源树
样例
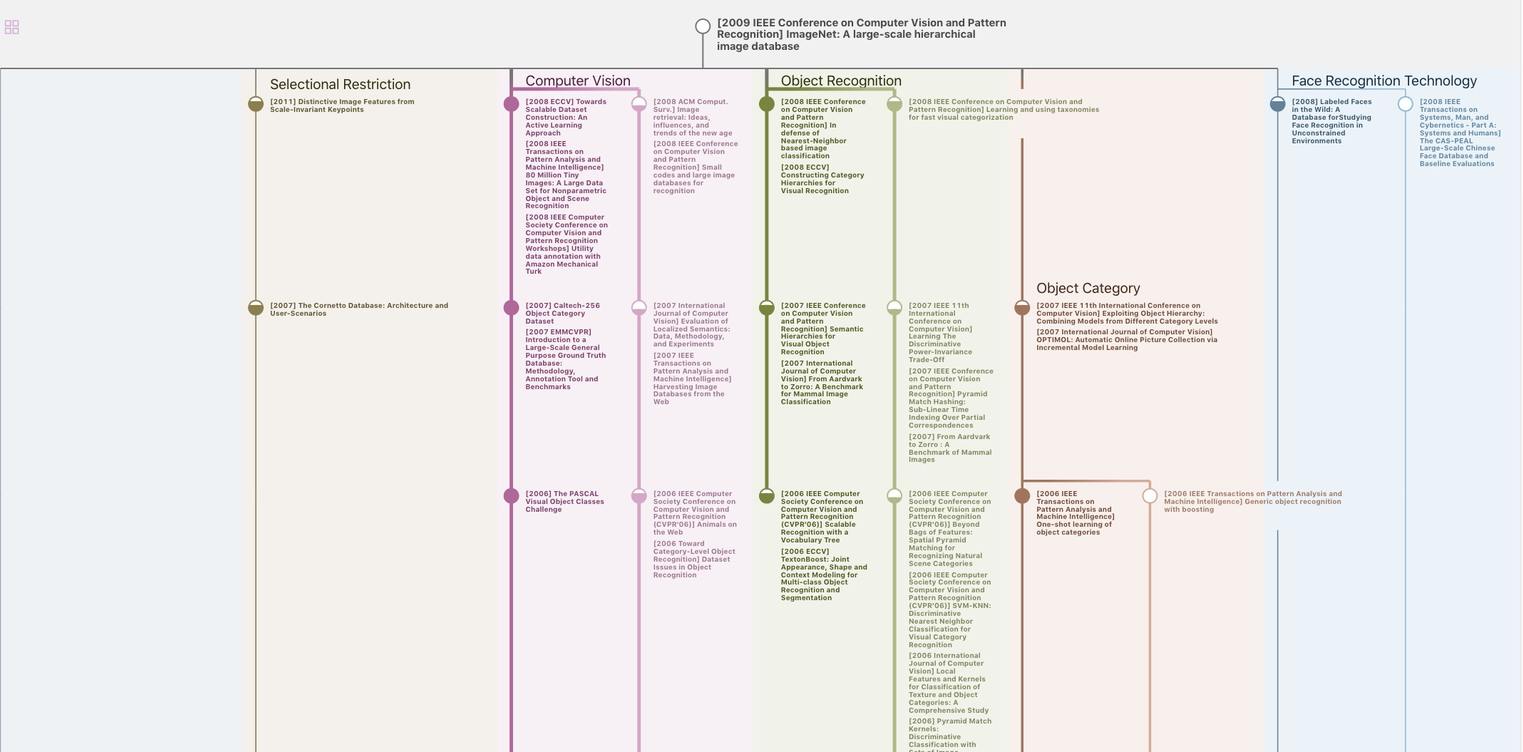
生成溯源树,研究论文发展脉络
Chat Paper
正在生成论文摘要