Sequential Estimation of Gaussian Process-Based Deep State-Space Models
arxiv(2023)
摘要
We consider the problem of sequential estimation of the unknowns of state-space and deep state-space models that include estimation of functions and latent processes of the models. The proposed approach relies on Gaussian and deep Gaussian processes that are implemented via random feature-based Gaussian processes. In these models, we have two sets of unknowns, highly nonlinear unknowns (the values of the latent processes) and conditionally linear unknowns (the constant parameters of the random feature-based Gaussian processes). We present a method based on particle filtering where the parameters of the random feature-based Gaussian processes are integrated out in obtaining the predictive density of the states and do not need particles. We also propose an ensemble version of the method, with each member of the ensemble having its own set of features. With several experiments, we show that the method can track the latent processes up to a scale and rotation.
更多查看译文
关键词
Deep state-space models,deep Gaussian pro-cesses,sparse Gaussian processes,random features,ensembles,particle filtering,Bayesian linear regression
AI 理解论文
溯源树
样例
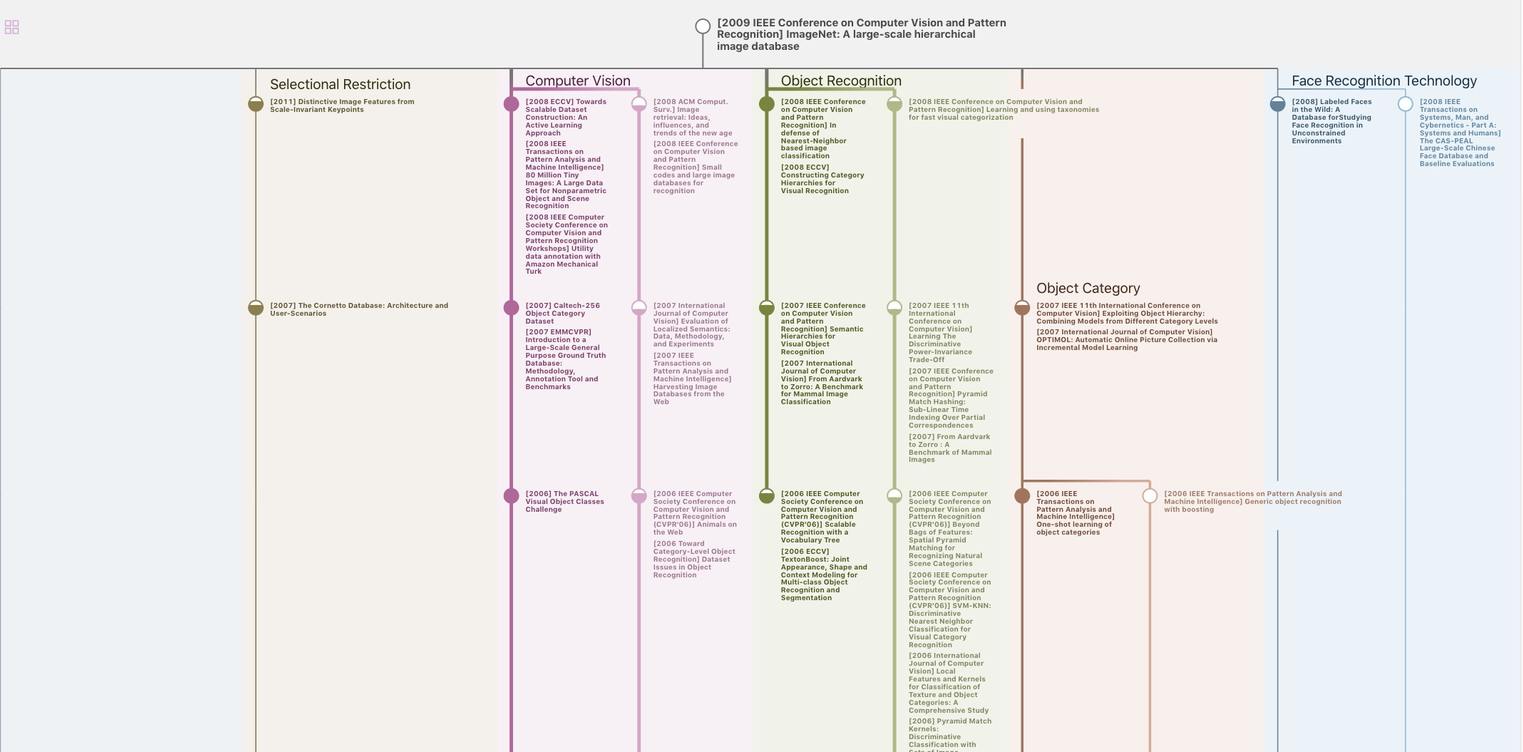
生成溯源树,研究论文发展脉络
Chat Paper
正在生成论文摘要