On the Statistical Benefits of Temporal Difference Learning
arxiv(2023)
摘要
Given a dataset on actions and resulting long-term rewards, a direct estimation approach fits value functions that minimize prediction error on the training data. Temporal difference learning (TD) methods instead fit value functions by minimizing the degree of temporal inconsistency between estimates made at successive time-steps. Focusing on finite state Markov chains, we provide a crisp asymptotic theory of the statistical advantages of this approach. First, we show that an intuitive inverse trajectory pooling coefficient completely characterizes the percent reduction in mean-squared error of value estimates. Depending on problem structure, the reduction could be enormous or nonexistent. Next, we prove that there can be dramatic improvements in estimates of the difference in value-to-go for two states: TD's errors are bounded in terms of a novel measure - the problem's trajectory crossing time - which can be much smaller than the problem's time horizon.
更多查看译文
关键词
learning,statistical benefits
AI 理解论文
溯源树
样例
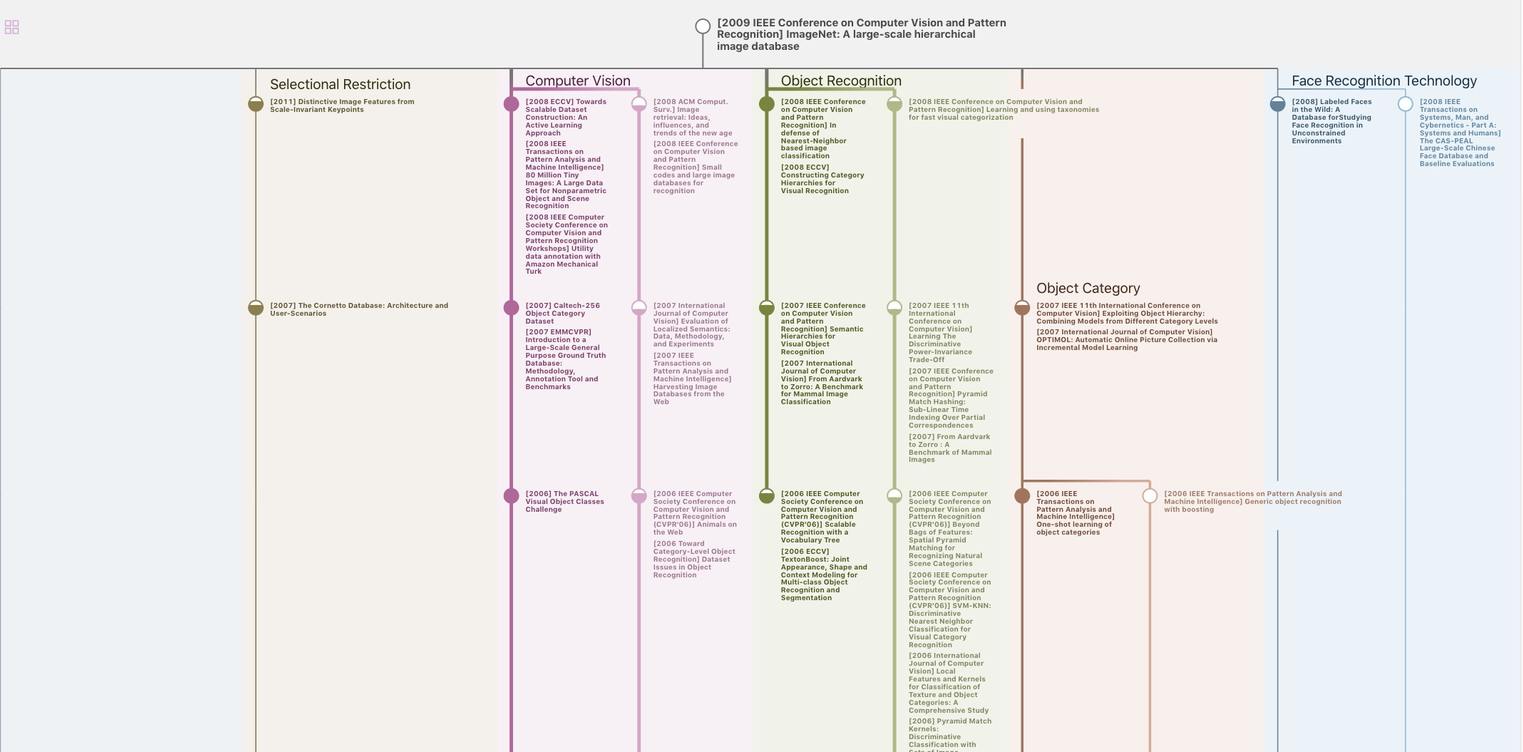
生成溯源树,研究论文发展脉络
Chat Paper
正在生成论文摘要