ReGANIE: Rectifying GAN Inversion Errors for Accurate Real Image Editing
arxiv(2023)
摘要
The StyleGAN family succeed in high-fidelity image generation and allow for flexible and plausible editing of generated images by manipulating the semantic-rich latent style space.However, projecting a real image into its latent space encounters an inherent trade-off between inversion quality and editability. Existing encoder-based or optimization-based StyleGAN inversion methods attempt to mitigate the trade-off but see limited performance. To fundamentally resolve this problem, we propose a novel two-phase framework by designating two separate networks to tackle editing and reconstruction respectively, instead of balancing the two. Specifically, in Phase I, a W-space-oriented StyleGAN inversion network is trained and used to perform image inversion and editing, which assures the editability but sacrifices reconstruction quality. In Phase II, a carefully designed rectifying network is utilized to rectify the inversion errors and perform ideal reconstruction. Experimental results show that our approach yields near-perfect reconstructions without sacrificing the editability, thus allowing accurate manipulation of real images. Further, we evaluate the performance of our rectifying network, and see great generalizability towards unseen manipulation types and out-of-domain images.
更多查看译文
关键词
rectifying reganie inversion errors,accurate real image editing,real image
AI 理解论文
溯源树
样例
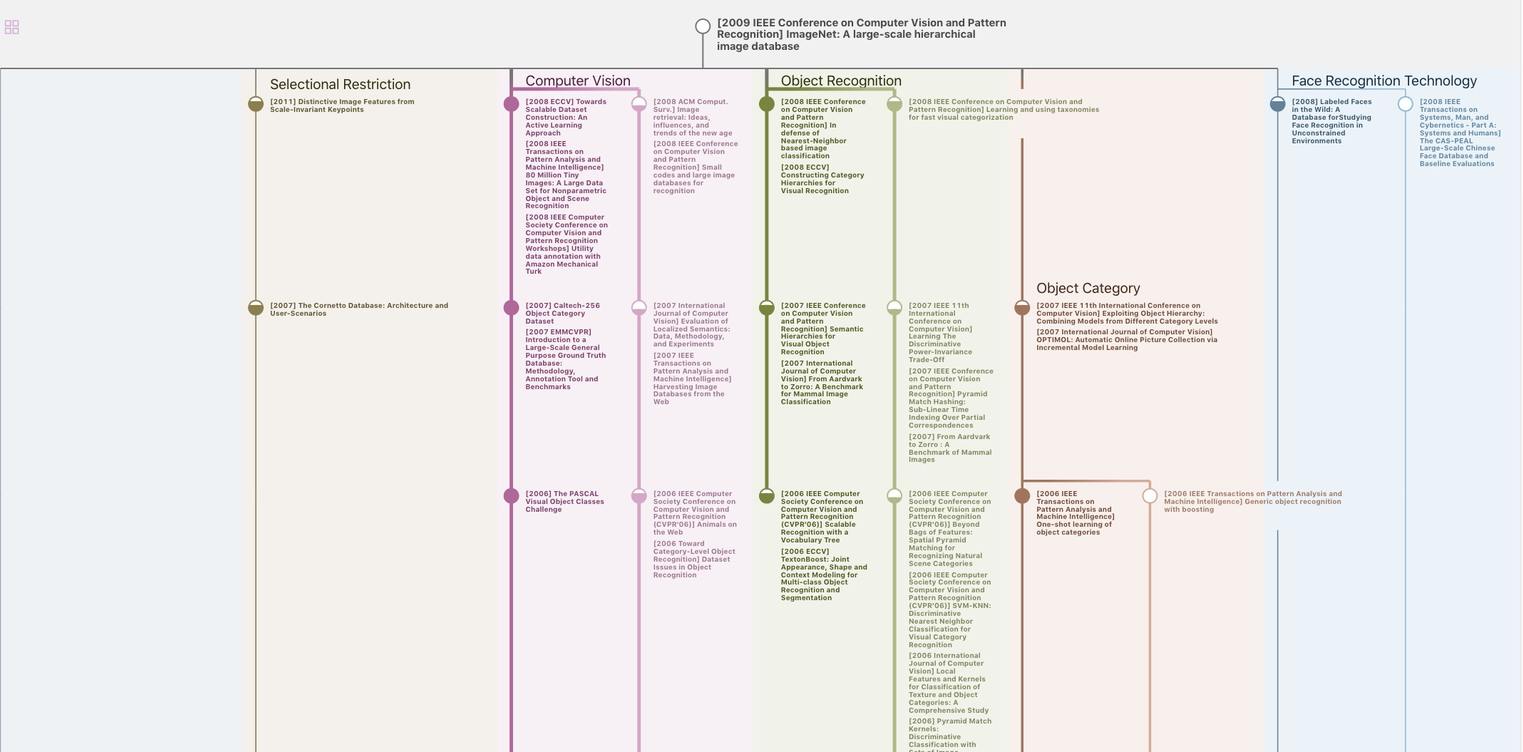
生成溯源树,研究论文发展脉络
Chat Paper
正在生成论文摘要