Nonlinear optimization filters for stochastic time-varying convex optimization
INTERNATIONAL JOURNAL OF ROBUST AND NONLINEAR CONTROL(2024)
摘要
We look at a stochastic time-varying optimization problem and we formulate online algorithms to find and track its optimizers in expectation. The algorithms are derived from the intuition that standard prediction and correction steps can be seen as a nonlinear dynamical system and a measurement equation, respectively, yielding the notion of nonlinear filter design. The optimization algorithms are then based on an extended Kalman filter in the unconstrained case, and on a bilinear matrix inequality condition in the constrained case. Some special cases and variations are discussed, notably the case of parametric filters, yielding certificates based on LPV analysis and, if one wishes, matrix sum-of-squares relaxations. Supporting numerical results are presented from real data sets in ride-hailing scenarios. The results are encouraging, especially when predictions are accurate, a case which is often encountered in practice when historical data is abundant.
更多查看译文
关键词
dissipativity,nonlinear filters,stochastic Optimization,time-varying Optimization
AI 理解论文
溯源树
样例
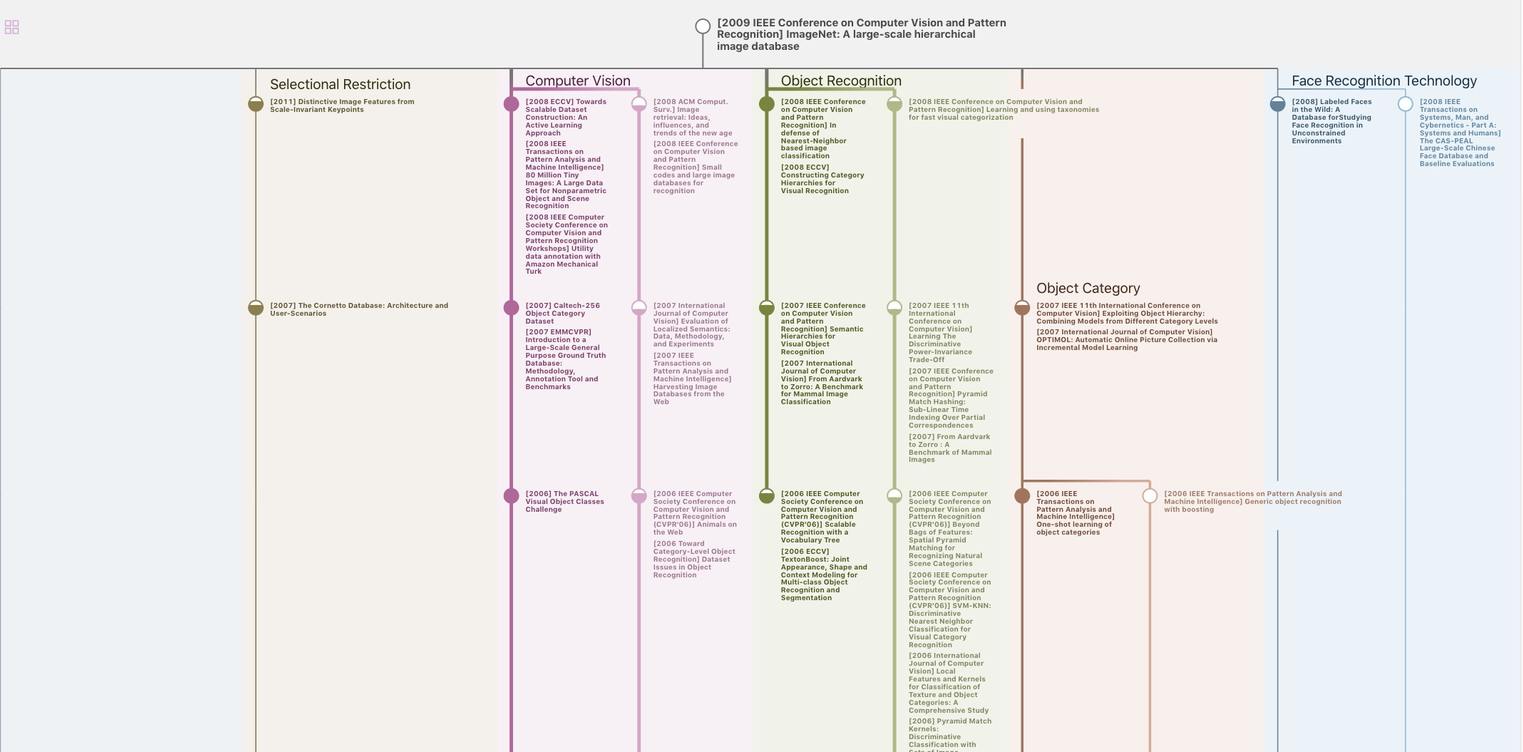
生成溯源树,研究论文发展脉络
Chat Paper
正在生成论文摘要