Friend-training: Learning from Models of Different but Related Tasks
arxiv(2023)
摘要
Current self-training methods such as standard self-training, co-training, tri-training, and others often focus on improving model performance on a single task, utilizing differences in input features, model architectures, and training processes. However, many tasks in natural language processing are about different but related aspects of language, and models trained for one task can be great teachers for other related tasks. In this work, we propose friend-training, a cross-task self-training framework, where models trained to do different tasks are used in an iterative training, pseudo-labeling, and retraining process to help each other for better selection of pseudo-labels. With two dialogue understanding tasks, conversational semantic role labeling and dialogue rewriting, chosen for a case study, we show that the models trained with the friend-training framework achieve the best performance compared to strong baselines.
更多查看译文
关键词
learning,models,related,friend-training
AI 理解论文
溯源树
样例
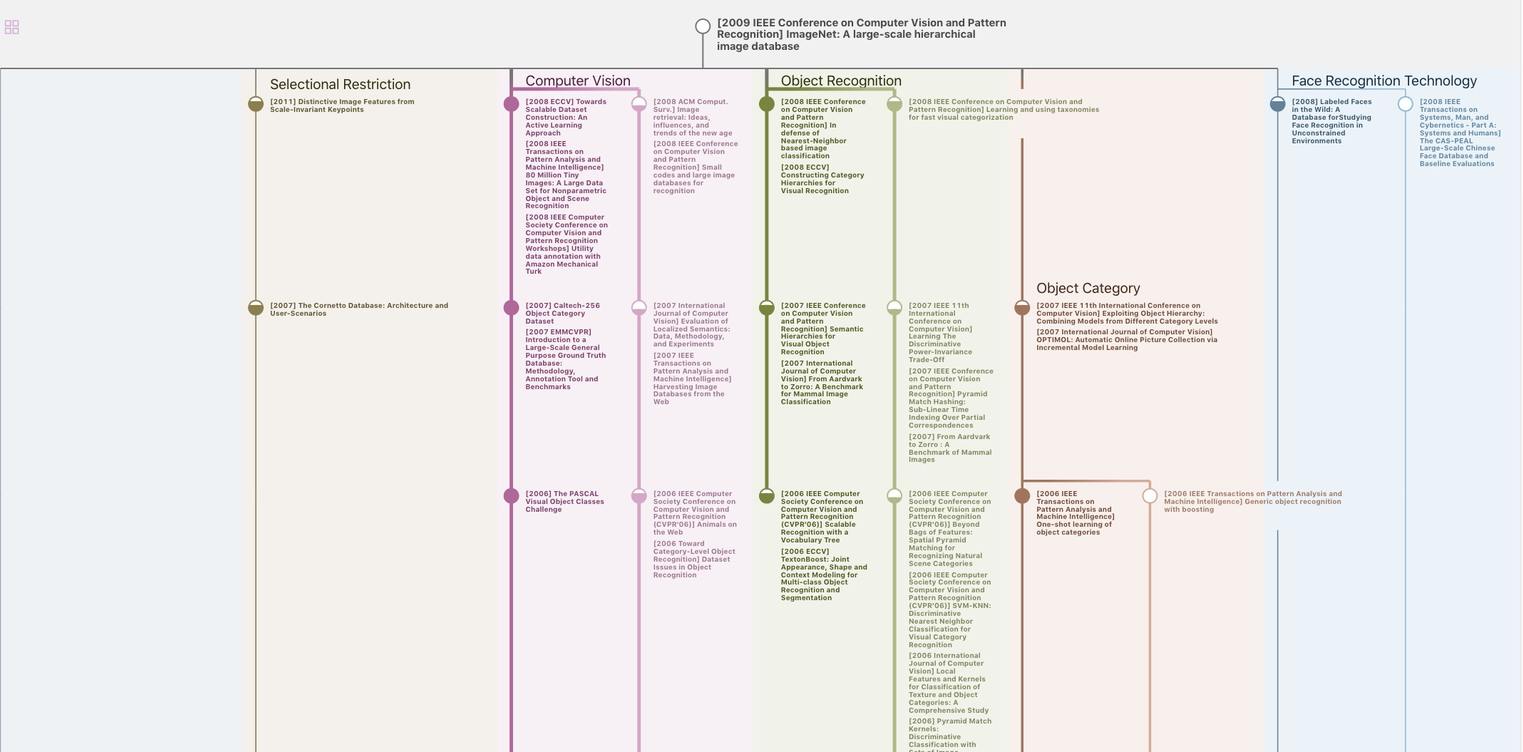
生成溯源树,研究论文发展脉络
Chat Paper
正在生成论文摘要