Dynamic Scheduled Sampling with Imitation Loss for Neural Text Generation
CoRR(2023)
摘要
State-of-the-art neural text generation models are typically trained to maximize the likelihood of each token in the ground-truth sequence conditioned on the previous target tokens. However, during inference, the model needs to make a prediction conditioned on the tokens generated by itself. This train-test discrepancy is referred to as exposure bias. Scheduled sampling is a curriculum learning strategy that gradually exposes the model to its own predictions during training to mitigate this bias. Most of the proposed approaches design a scheduler based on training steps, which generally requires careful tuning depending on the training setup. In this work, we introduce Dynamic Scheduled Sampling with Imitation Loss (DySI), which maintains the schedule based solely on the training time accuracy, while enhancing the curriculum learning by introducing an imitation loss, which attempts to make the behavior of the decoder indistinguishable from the behavior of a teacher-forced decoder. DySI is universally applicable across training setups with minimal tuning. Extensive experiments and analysis show that DySI not only achieves notable improvements on standard machine translation benchmarks, but also significantly improves the robustness of other text generation models.
更多查看译文
关键词
exposure bias,text generation
AI 理解论文
溯源树
样例
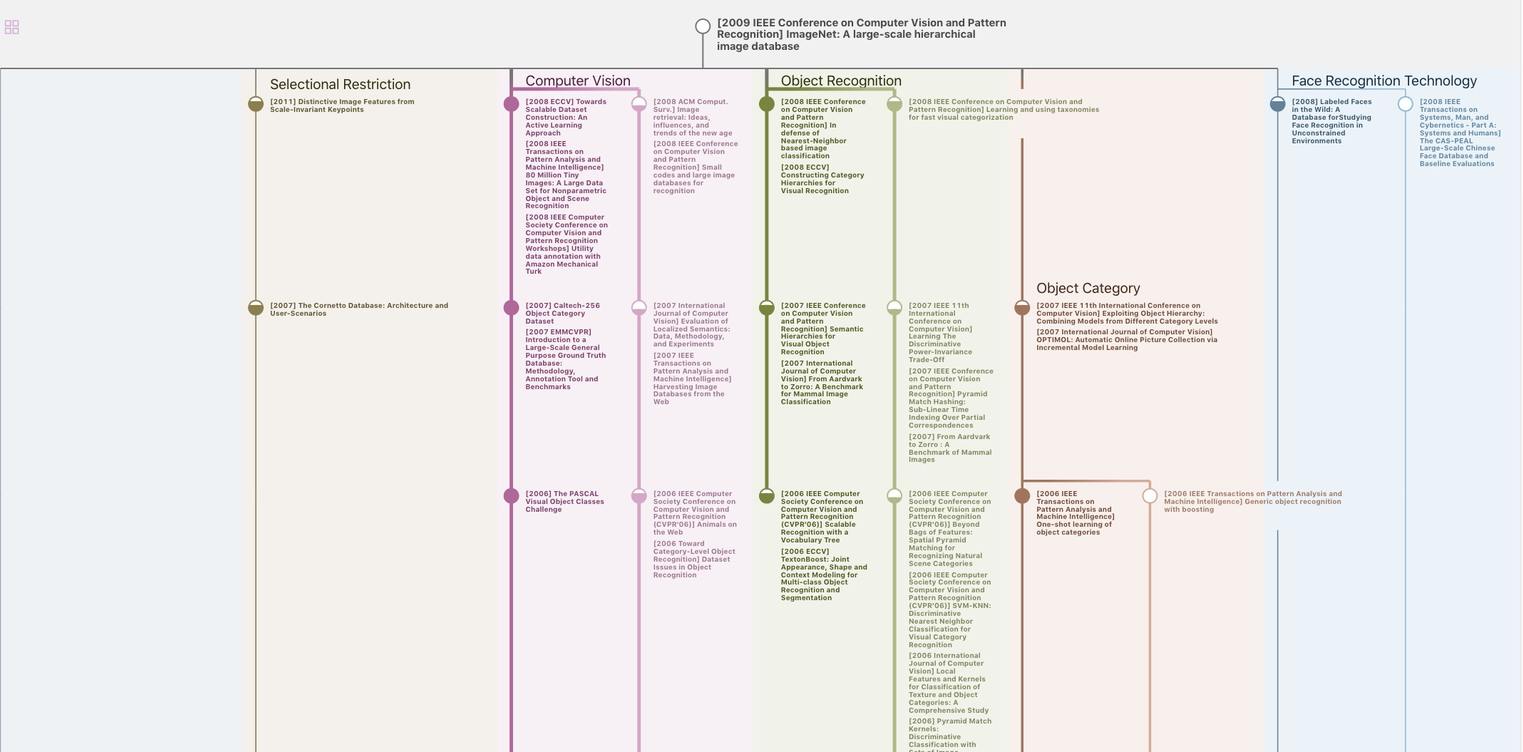
生成溯源树,研究论文发展脉络
Chat Paper
正在生成论文摘要