Interpreting Robustness Proofs of Deep Neural Networks
arXiv (Cornell University)(2023)
Abstract
In recent years numerous methods have been developed to formally verify the robustness of deep neural networks (DNNs). Though the proposed techniques are effective in providing mathematical guarantees about the DNNs behavior, it is not clear whether the proofs generated by these methods are human-interpretable. In this paper, we bridge this gap by developing new concepts, algorithms, and representations to generate human understandable interpretations of the proofs. Leveraging the proposed method, we show that the robustness proofs of standard DNNs rely on spurious input features, while the proofs of DNNs trained to be provably robust filter out even the semantically meaningful features. The proofs for the DNNs combining adversarial and provably robust training are the most effective at selectively filtering out spurious features as well as relying on human-understandable input features.
MoreTranslated text
Key words
robustness proofs,neural networks
AI Read Science
Must-Reading Tree
Example
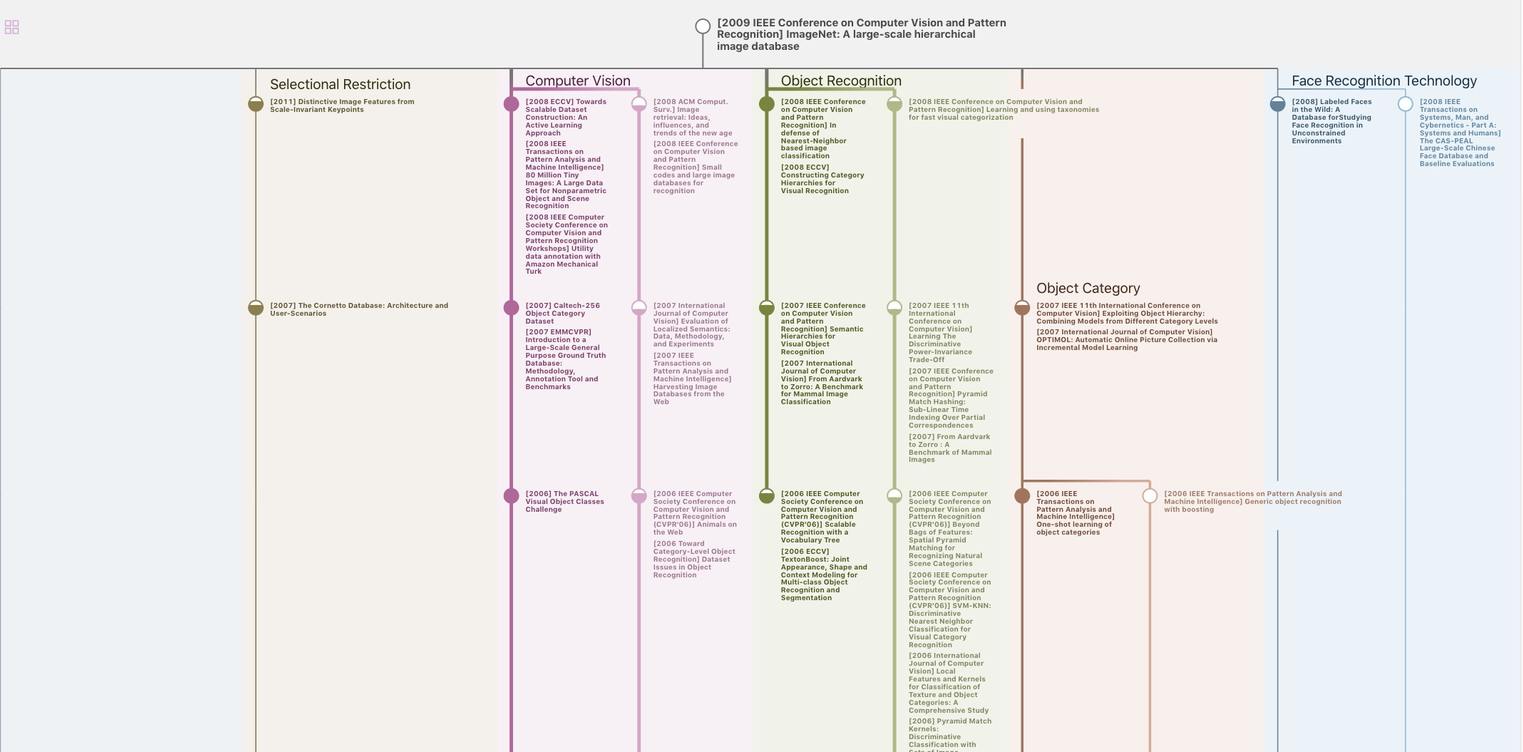
Generate MRT to find the research sequence of this paper
Chat Paper
Summary is being generated by the instructions you defined