Considering strategies for SNP selection in genetic and polygenic risk scores.
Frontiers in genetics(2022)
摘要
Genetic risk scores (GRS) and polygenic risk scores (PRS) are weighted sums of, respectively, several or many genetic variant indicator variables. Although they are being increasingly proposed for clinical use, the best ways to construct them are still actively debated. In this commentary, we present several case studies illustrating practical challenges associated with building or attempting to improve score performance when there is expected to be heterogeneity of disease risk between cohorts or between subgroups of individuals. Specifically, we contrast performance associated with several ways of selecting single nucleotide polymorphisms (SNPs) for inclusion in these scores. By considering GRS and PRS as predictors that are measured with error, insights into their strengths and weaknesses may be obtained, and SNP selection approaches play an important role in defining such errors.
更多查看译文
关键词
feature selection,high-dimensional data,instrumental variable methods,measurement error,mendelian randomization,polygenic risk scores,regularized models
AI 理解论文
溯源树
样例
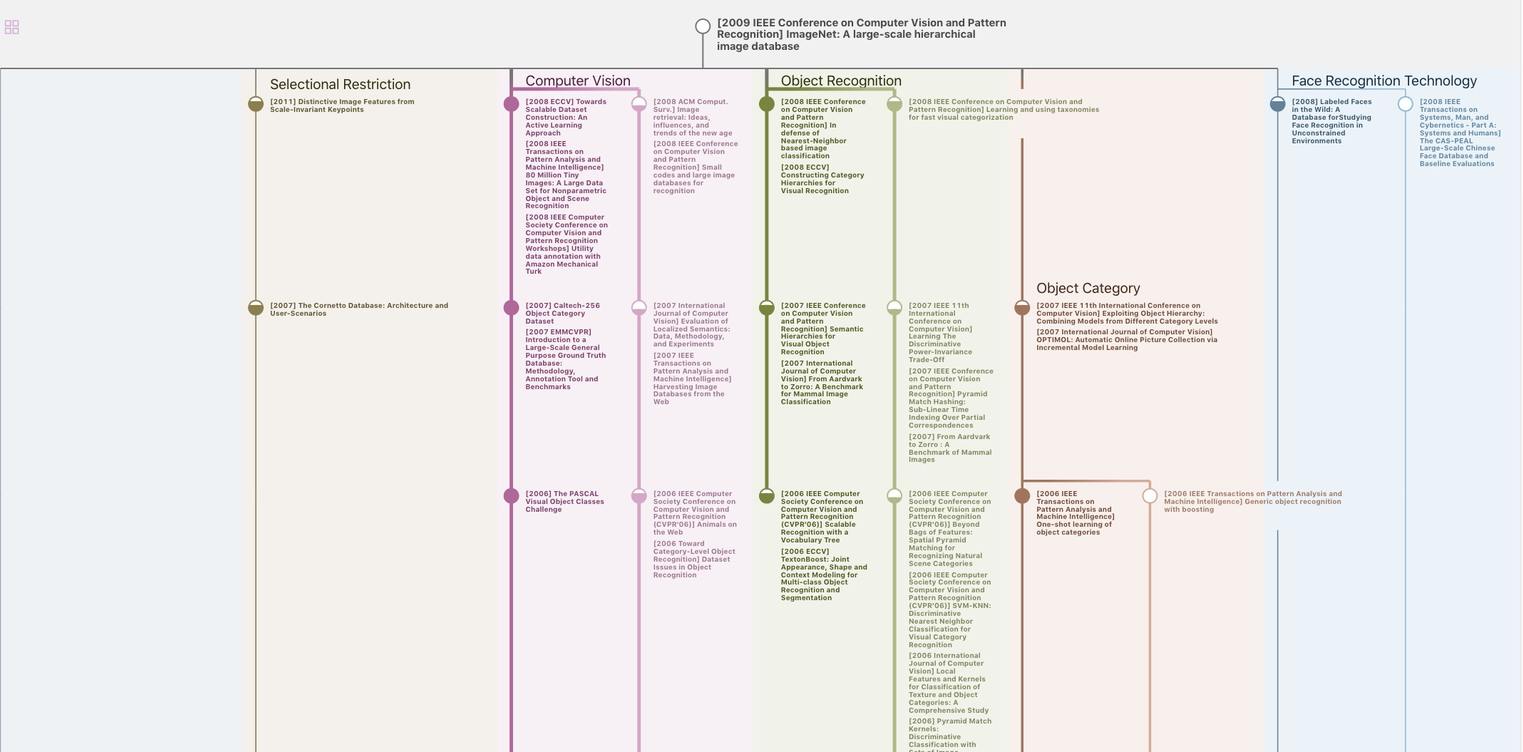
生成溯源树,研究论文发展脉络
Chat Paper
正在生成论文摘要