CNN-Based Transformer Model for Fault Detection in Power System Networks
IEEE TRANSACTIONS ON INSTRUMENTATION AND MEASUREMENT(2023)
摘要
Fault detection and localization in electrical power lines has long been a crucial challenge for electrical engineers as it allows the detected fault to be isolated and recovered promptly. These faults, if neglected, can rupture the normal operation of the network and drastically damage the power lines and the equipment attached to it. The wastage of power and money due to these faults can be harmful to the economy of an industry or even a country. Therefore, efficient fault detection mechanisms have become crucial for the well-being of this power-hungry world. This research presents an end-to-end deep learning strategy to detect and localize symmetrical and unsymmetrical faults as well as high-impedance faults (HIFs) in a distribution system. This research proposes a novel deep convolutional neural network (CNN) transformer model to automatically detect the type and phase of the fault as well as the location of the fault. The proposed model utilizes 1-D deep CNNs for feature extraction and transformer encoder for sequence learning. The transformer encoder utilizes an attention mechanism to integrate the sequence embeddings and focus on significant time steps to learn long-term dependence to extract the context of the temporal current data. The different faults were simulated in MATLAB Simulink using IEEE 14-bus distribution system. The proposed models were found to produce better performance on the test database when evaluated using F1-Score, Matthews correlation coefficient (MCC), and accuracy. The models also produced better predictions on HIFs compared to conventional fault-detection techniques.
更多查看译文
关键词
Feature extraction,Transformers,Data models,Fault detection,Deep learning,Impedance,Databases,fault detection,high-impedance fault (HIF),transformer model
AI 理解论文
溯源树
样例
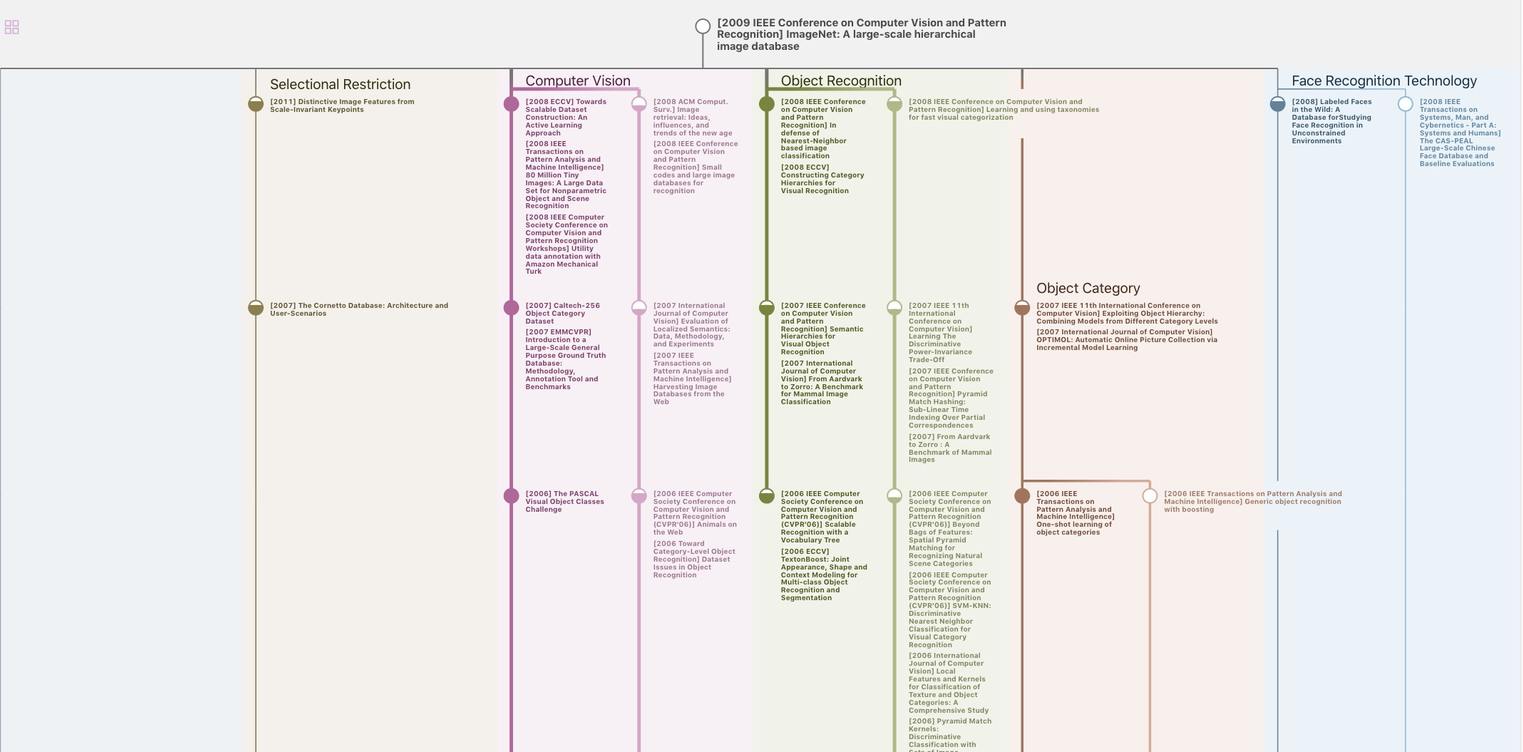
生成溯源树,研究论文发展脉络
Chat Paper
正在生成论文摘要