A Clustering-based model for the Generation of Diversified Recommendations
2022 IEEE Silchar Subsection Conference (SILCON)(2022)
摘要
The primary goal of a recommender system is to generate accurate recommendations according to the user’s interests. But the user’s satisfaction increases when they get a chance to view the diverse categories of items. There exist several works on the generation of diverse recommendations but the performance of these methods often gets limited due to the issues such as cold start, filter bubble long tail, and grey sheep. Moreover, these methods do not consider the user’s preference regarding exploration and exploitation while generating the recommendations. To this extent, this work proposes a model known as the iterative clustering-based diversity model, which can generate diverse recommendations and also solve the above-said issues. It groups the items based on the item description using the TF-IDF algorithm. The model generates two recommendations in such a way that one recommendation is similar and the other is different in comparison with the last interaction made by the user. The model has been evaluated on the benchmark dataset and has achieved promising results.
更多查看译文
关键词
Recommender systems,diversity,filter bubble,grey sheep,long tail problem,exploration exploitation
AI 理解论文
溯源树
样例
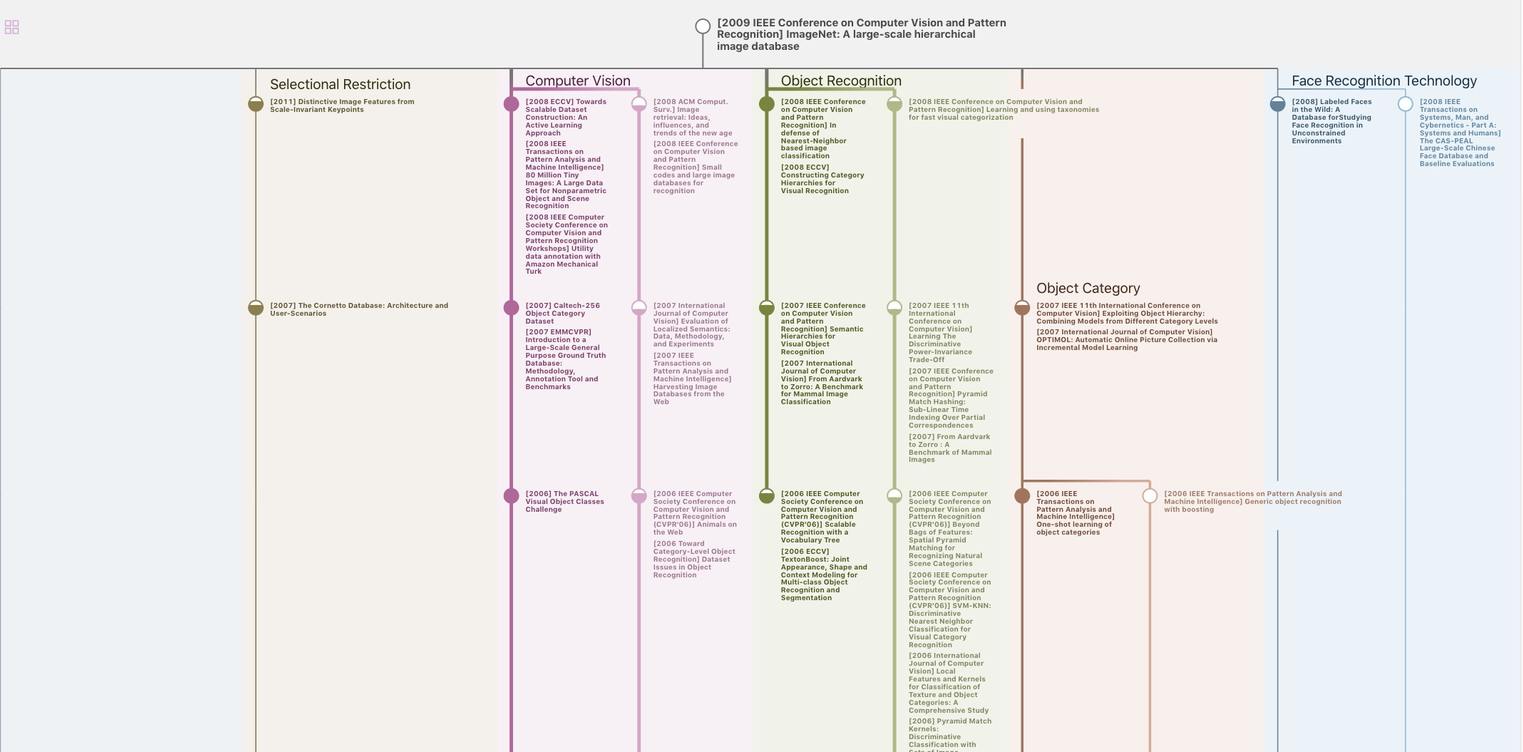
生成溯源树,研究论文发展脉络
Chat Paper
正在生成论文摘要