Automated Continual Learning of Defect Identification in Coherent Diffraction Imaging
2022 IEEE/ACM International Workshop on Artificial Intelligence and Machine Learning for Scientific Applications (AI4S)(2022)
摘要
X-ray Bragg coherent diffraction imaging (BCDI) is widely used for materials characterization. However, obtaining X-ray diffraction data is difficult and computationally intensive. Here, we introduce a machine learning approach to identify crystalline line defects in samples from the raw coherent diffraction data. To automate this process, we compose a workflow coupling coherent diffraction data generation with training and inference of deep neural network defect classifiers. In particular, we adopt a continual learning approach, where we generate training and inference data as needed based on the accuracy of the defect classifier instead of all training data generated a priori. The results show that our approach improves the accuracy of defect classifiers while using much fewer samples of data.
更多查看译文
关键词
HPC workflows,defect identification,continual learning,catastrophic forgetting
AI 理解论文
溯源树
样例
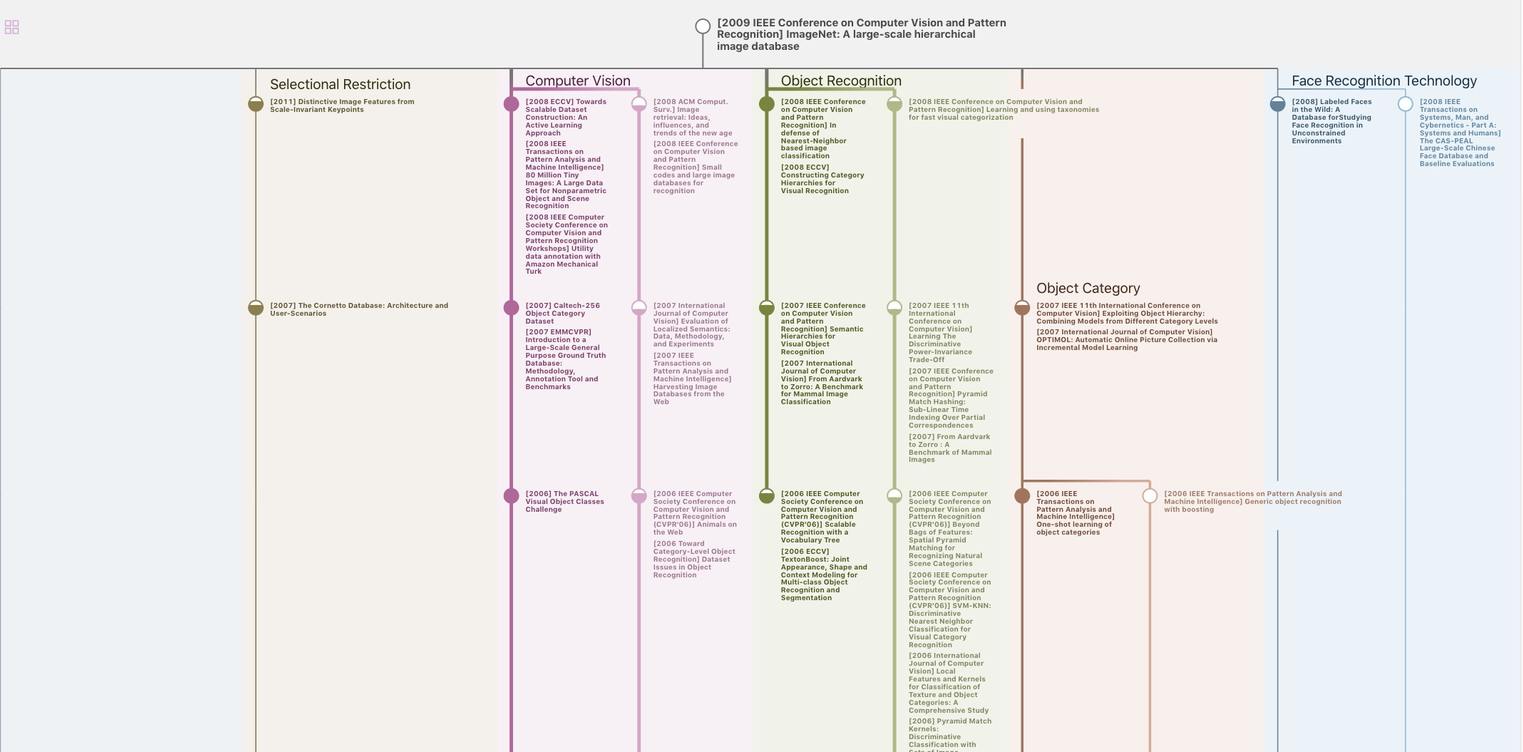
生成溯源树,研究论文发展脉络
Chat Paper
正在生成论文摘要