Multiclass Objects Localization Using Deep Learning Technique in Autonomous Vehicle
2022 6th International Conference on Computation System and Information Technology for Sustainable Solutions (CSITSS)(2022)
摘要
In a conventional automobile, the vehicle is controlled by a human brain. The eyes, which gather visual data from surrounding traffic, are the most important human sense. This visual input is then sent to the brain through the eyes. The eyes then communicate this visual information to the brain, which is in charge of operating the car by applying brakes, accelerating, or turning left or right. Autonomous vehicles or self-driving cars, on the other hand, rely on sensors, LiDAR, RADAR, and cameras to gather traffic data. This data from multiple sensors and cameras must be processed for the car to travel smoothly and securely. Intelligent software that can simulate a human brain, classify, and localize numerous sorts of traffic objects in real- time is required for autonomous vehicles. The image is the major input of an autonomous vehicle, and the program must detect the object from the input image. Object localization, or predicting the true position of a recognized object in an image, is a critical problem. The software can only predict the next step or decide what distance to keep between the car and other traffic objects, as well as whether to brake and accelerate, after exact object localization. We are proposing an object localization method using YOLOv5 as an object detector and LiDAR to calculate the distance. We created a custom dataset of 6000 images of five different classes and achieved 89.2% mAP value and at a .95 IoU threshold, our model achieved 52.1 mAP. We have also compared several conceivable computer vision challenges and real-time deep learning models in object localization in this work.
更多查看译文
关键词
Object localization,Deep learning,YOLOv5,SSD,Faster RCNN,Self-driving car,autonomous
AI 理解论文
溯源树
样例
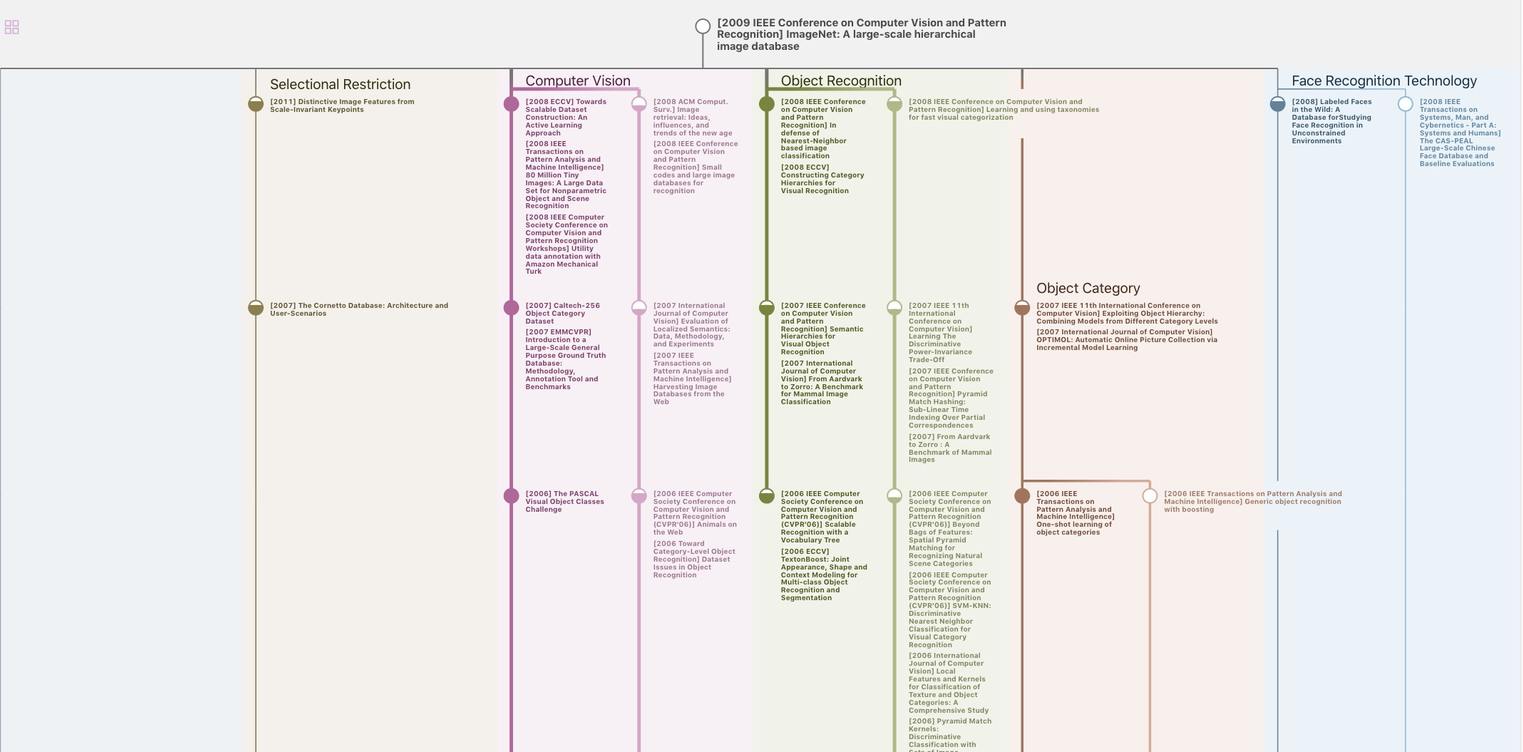
生成溯源树,研究论文发展脉络
Chat Paper
正在生成论文摘要