Truthful Incentive Mechanism for Federated Learning with Crowdsourced Data Labeling
arxiv(2023)
摘要
Federated learning (FL) has emerged as a promising paradigm that trains machine learning (ML) models on clients' devices in a distributed manner without the need of transmitting clients' data to the FL server. In many applications of ML, the labels of training data need to be generated manually by human agents. In this paper, we study FL with crowdsourced data labeling where the local data of each participating client of FL are labeled manually by the client. We consider the strategic behavior of clients who may not make desired effort in their local data labeling and local model computation and may misreport their local models to the FL server. We characterize the performance bounds on the training loss as a function of clients' data labeling effort, local computation effort, and reported local models. We devise truthful incentive mechanisms which incentivize strategic clients to make truthful efforts and report true local models to the server. The truthful design exploits the non-trivial dependence of the training loss on clients' efforts and local models. Under the truthful mechanisms, we characterize the server's optimal local computation effort assignments. We evaluate the proposed FL algorithms with crowdsourced data labeling and the incentive mechanisms using experiments.
更多查看译文
关键词
Federated Learning,Crowdsourcing,Incentive Mechanism
AI 理解论文
溯源树
样例
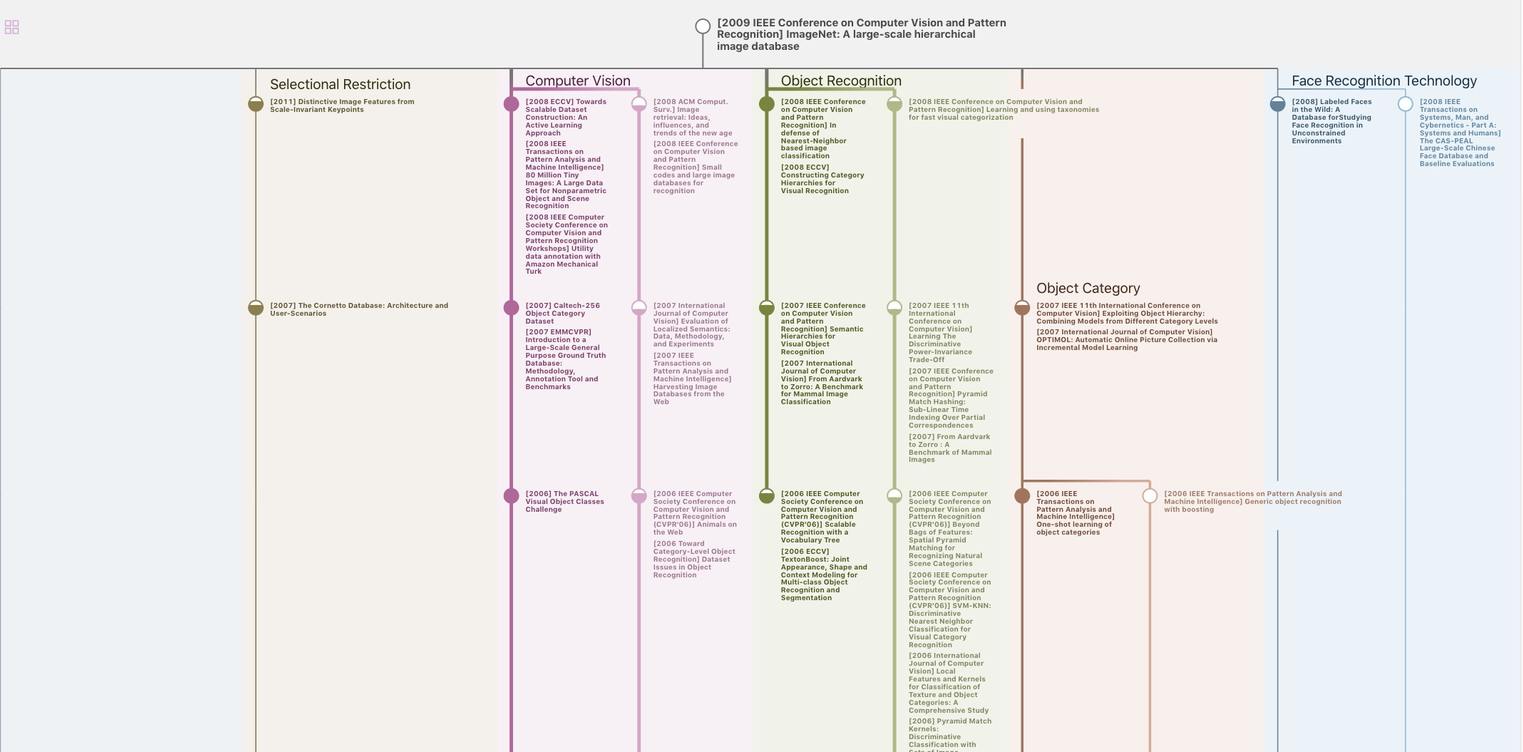
生成溯源树,研究论文发展脉络
Chat Paper
正在生成论文摘要