Two for One: Diffusion Models and Force Fields for Coarse-Grained Molecular Dynamics
arxiv(2023)
摘要
Coarse-grained (CG) molecular dynamics enables the study of biological processes at temporal and spatial scales that would be intractable at an atomistic resolution. However, accurately learning a CG force field remains a challenge. In this work, we leverage connections between score-based generative models, force fields and molecular dynamics to learn a CG force field without requiring any force inputs during training. Specifically, we train a diffusion generative model on protein structures from molecular dynamics simulations, and we show that its score function approximates a force field that can directly be used to simulate CG molecular dynamics. While having a vastly simplified training setup compared to previous work, we demonstrate that our approach leads to improved performance across several small- to medium-sized protein simulations, reproducing the CG equilibrium distribution, and preserving dynamics of all-atom simulations such as protein folding events.
更多查看译文
关键词
diffusion models,dynamics,molecular,force fields,coarse-grained
AI 理解论文
溯源树
样例
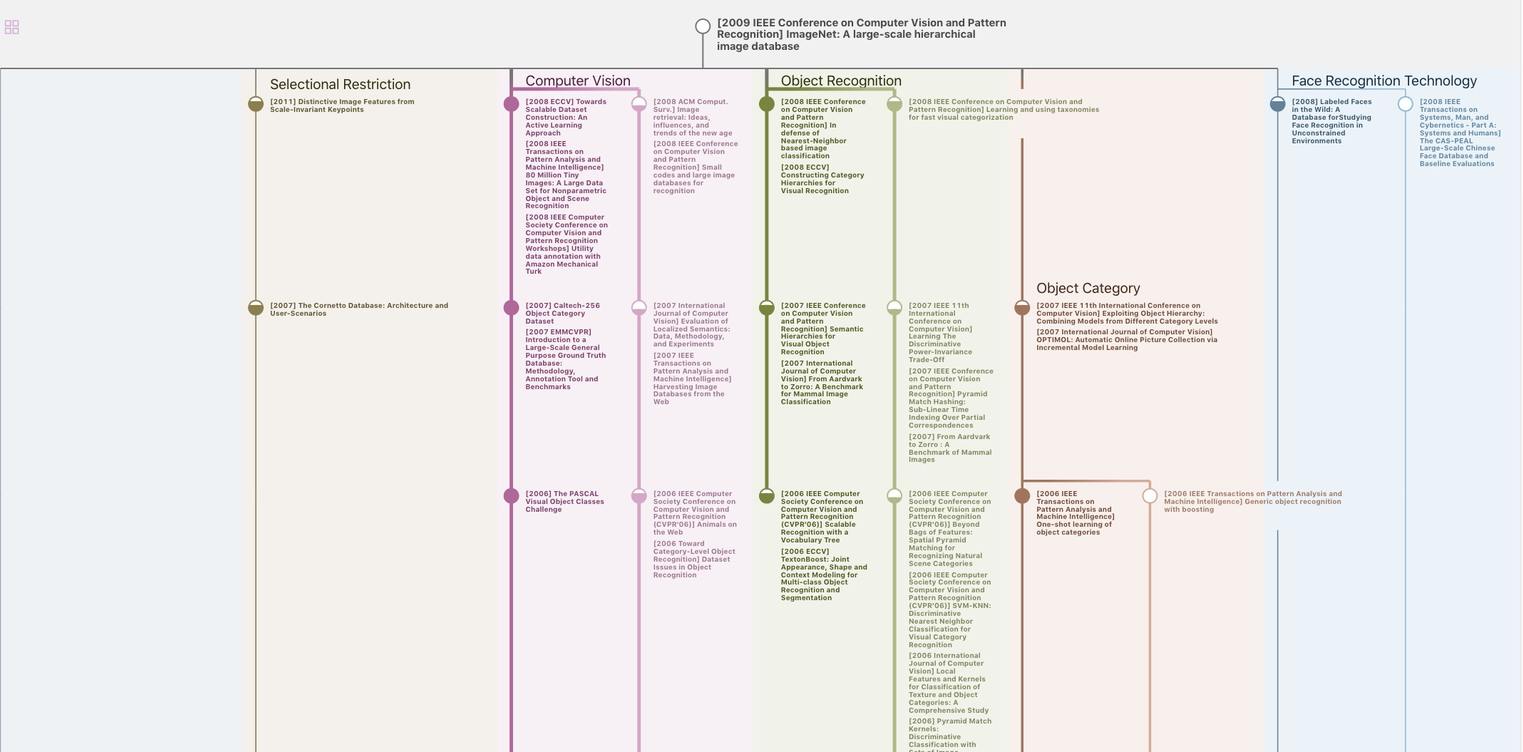
生成溯源树,研究论文发展脉络
Chat Paper
正在生成论文摘要