Revisiting Link Prediction on Heterogeneous Graphs with a Multi-view Perspective
2022 IEEE International Conference on Data Mining (ICDM)(2022)
摘要
In this work, we present a novel approach for link prediction on heterogeneous networks – networks that accommodate multiple types of nodes as well as multiple types of relations among them. Specifically, we propose a multi-view network representation learning framework to incorporate structural intuitions from the underlying graph and enrich the relational representations for link prediction. The method relies on the metapath view, the community view, and the subgraph view between a source and target node pair whose linkage is to be predicted. Furthermore, our proposed model leverages a relation-aware attention mechanism to aggregate the candidate contexts in a principled way. Empirically, we demonstrate that the proposed architecture outperforms state-of-the-art transductive and inductive methods in link prediction by a significant margin. A detailed ablation study and attention weight visualizations suggest that the chosen views are complementary and useful to predict links robustly.
更多查看译文
关键词
link prediction,heterogeneous graphs,multiview learning,community learning
AI 理解论文
溯源树
样例
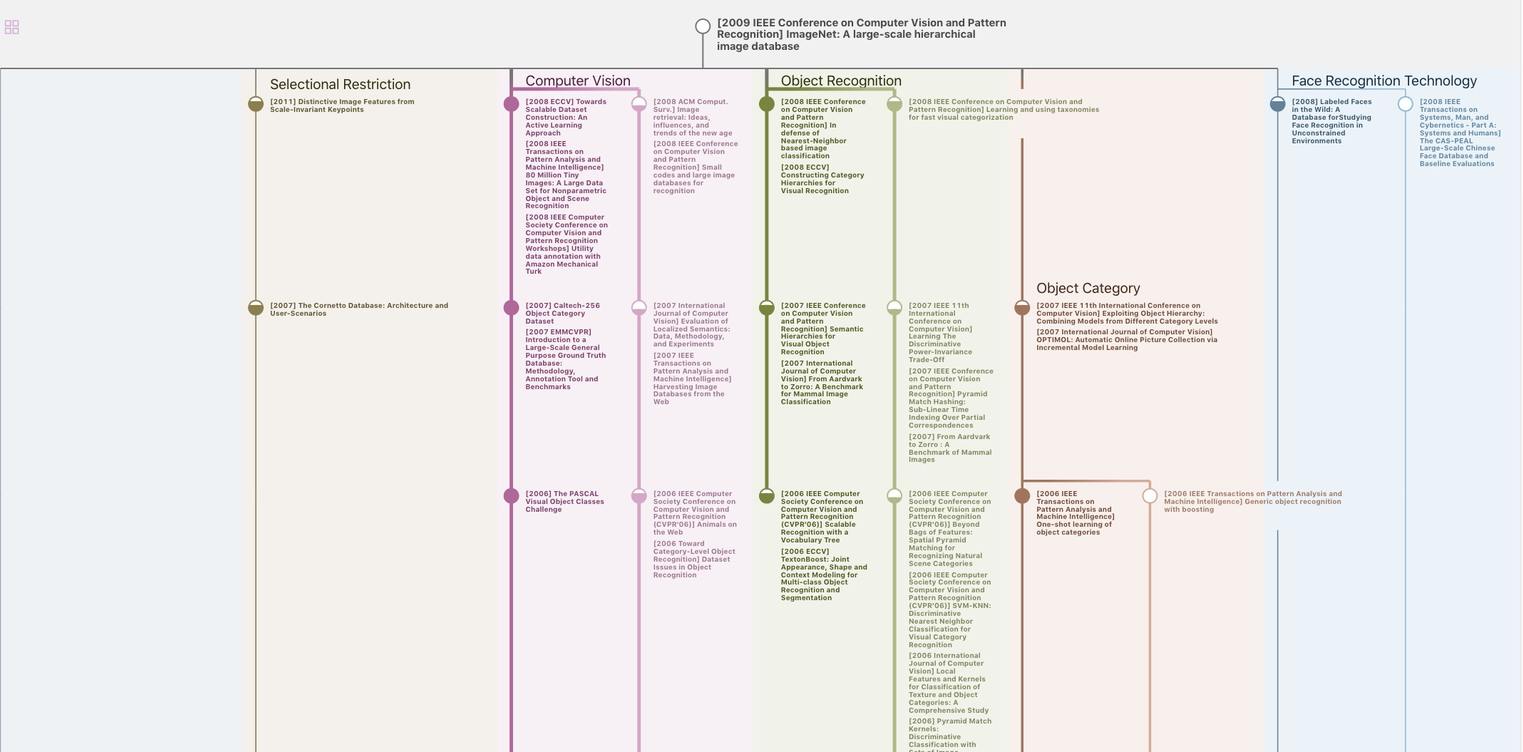
生成溯源树,研究论文发展脉络
Chat Paper
正在生成论文摘要