Post-Robustifying Deep Anomaly Detection Ensembles by Model Selection
2022 IEEE International Conference on Data Mining (ICDM)(2022)
摘要
Anomaly detection has been a major research area in machine learning with deep ensemble models showing exceptional performance. However, formal verification of robustness for anomaly detection in general, and ensemble models in particular, has been mostly neglected. Moreover, given an already trained, non-robust model, there is no way to adapt it for robustness as a post-processing step as of yet. By harnessing properties of ensemble methods - in particular of the DEAN model - we are the first to post-robustify a model via submodel selection. Beyond this new capability, our method significantly increases verification scalability by employing the inherent properties of ensemble methods. Our experiments show that the DEAN model is most suitable for our method: it proves to be the most robust from the start, allows for post-robustification and keeps a stable runtime across all datasets considered.
更多查看译文
关键词
Robustness,formal verification,anomaly detection,ensemble methods
AI 理解论文
溯源树
样例
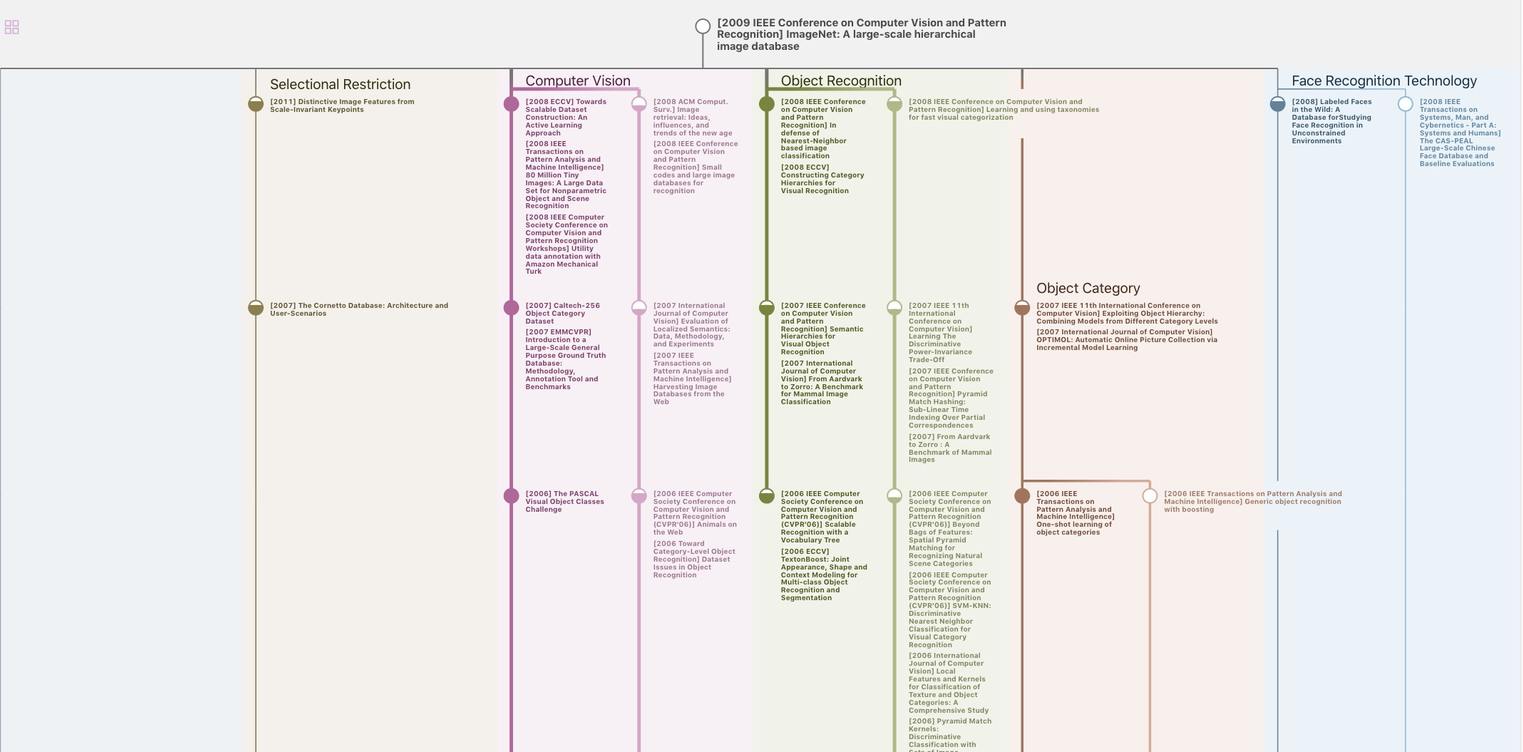
生成溯源树,研究论文发展脉络
Chat Paper
正在生成论文摘要