Deep Neural Network-based Video Processing to Obtain Upper-Extremity Motor Performance Toward Assessment of Cognitive and Motor Function.
IEEE Transactions on Neural Systems and Rehabilitation Engineering(2022)
摘要
Dementia is an increasing global health challenge. Motoric Cognitive Risk Syndrome (MCR) is a predementia stage that can be used to predict future occurrence of dementia. Traditionally, gait speed and subjective memory complaints are used to identify old adults with MCR. Our previous studies indicated that dual-task upper-extremity motor performance (DTUEMP) quantified by a single wrist-worn sensor was correlated with both motor and cognitive function. Therefore, the DTUEMP had a potential to be used in the diagnosis of MCR. Instead of using inertial sensors to capture kinematic data of upper-extremity movements, here we proposed a deep neural network-based video processing model to obtain DTUEMP metrics from a 20-second repetitive elbow flexion-extension test under dual-task condition. In details, we used a deep residual neural network to obtain joint coordinate set of the elbow and wrist in each frame, and then used optical flow method to correct the joint coordinates generated by the neural network. The coordinate sets of all frames in a video recording are used to generate angle sequence which represents rotation angle of the line between the wrist and elbow. Then, the DTUEMP metrics (the mean and SD of flexion and extension phase) are derived from angle sequence. Multi-task learning (MTL) is used to assess cognitive and motor function represented by MMSE and TUG scores based on DTUEMP metrics, with single-task learning (STL) linear model as a benchmark. The results showed a good agreement (r ≥ 0.80 and ICC ≥ 0.58) between the derived DTUEMP metrics from our proposed model and the ones from clinically validated sensor processing model. We also found that there were correlations with statistical significance (p < 0.05) between some of video-derived DTUEMP metrics (i.e. the mean of flexion time and extension time) and clinical cognitive scale (Mini-Mental State Examination, MMSE). Additionally, some of video-derived DTUEMP metrics (i.e. the mean and standard deviation of flexion time and extension time) was also associated with the scores of timed-up and go (TUG) which is a gold standard to measure functional mobility. Mean absolute percentage error (MAPE) of MTL surpassed that of STL (For MMSE, MTL: 18.63%, STL: 23.18%. For TUG, MTL: 17.88%, STL: 22.53%). The experiments with different light conditions and shot angles verified the robustness of our proposed video processing model to extract DTUEMP metrics in potentially various home environments (r ≥ 0.58 and ICC ≥ 0.71). This study shows possibility of replacing sensor processing model with video processing model for analyzing the DTUEMP and a promising future to adjuvant diagnosis of MCR via a mobile platform.
更多查看译文
关键词
Dementia,motoric cognitive risk syndrome,telehealth,tele-medicine,deep residual neural network,mobile health,video processing
AI 理解论文
溯源树
样例
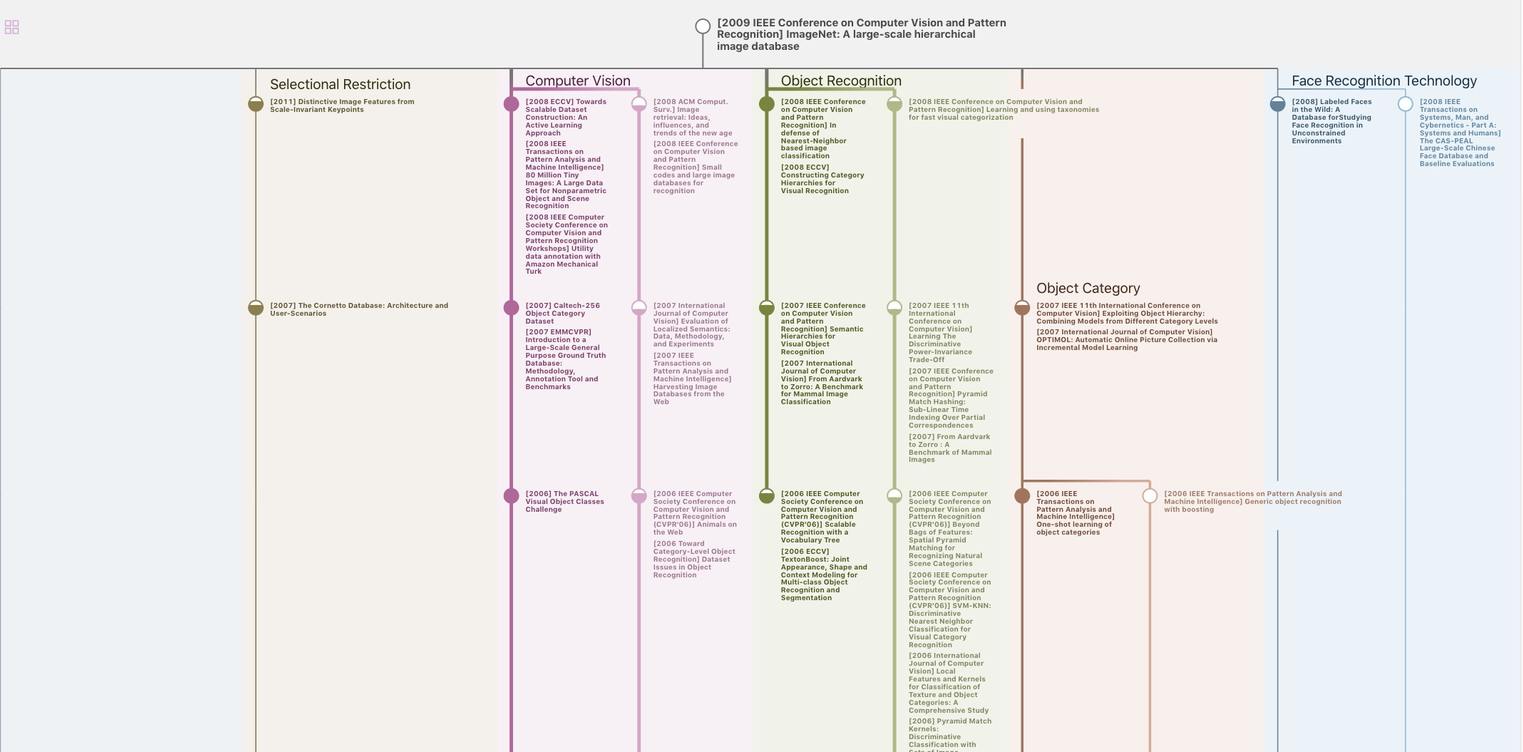
生成溯源树,研究论文发展脉络
Chat Paper
正在生成论文摘要