Energy Efficiency of Training Neural Network Architectures: An Empirical Study
arxiv(2023)
摘要
The evaluation of Deep Learning models has traditionally focused on criteria such as accuracy, F1 score, and related measures. The increasing availability of high computational power environments allows the creation of deeper and more complex models. However, the computations needed to train such models entail a large carbon footprint. In this work, we study the relations between DL model architectures and their environmental impact in terms of energy consumed and CO$_2$ emissions produced during training by means of an empirical study using Deep Convolutional Neural Networks. Concretely, we study: (i) the impact of the architecture and the location where the computations are hosted on the energy consumption and emissions produced; (ii) the trade-off between accuracy and energy efficiency; and (iii) the difference on the method of measurement of the energy consumed using software-based and hardware-based tools.
更多查看译文
关键词
training neural network architectures,energy efficiency,neural network
AI 理解论文
溯源树
样例
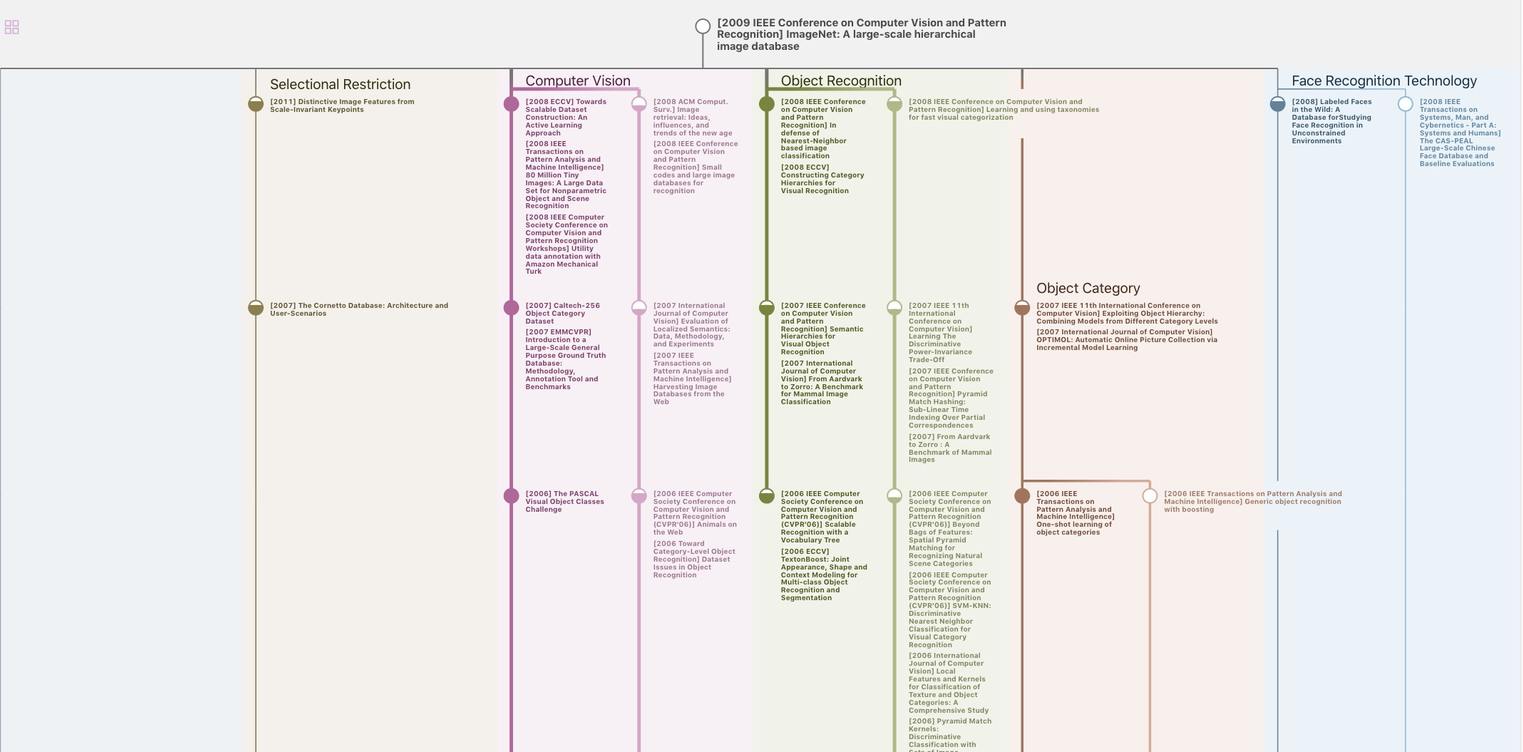
生成溯源树,研究论文发展脉络
Chat Paper
正在生成论文摘要