Stone's theorem for distributional regression in Wasserstein distance
arxiv(2023)
摘要
We extend the celebrated Stone's theorem to the framework of distributional regression. More precisely, we prove that weighted empirical distribution with local probability weights satisfying the conditions of Stone's theorem provide universally consistent estimates of the conditional distributions, where the error is measured by the Wasserstein distance of order p $\ge$ 1. Furthermore, for p = 1, we determine the minimax rates of convergence on specific classes of distributions. We finally provide some applications of these results, including the estimation of conditional tail expectation or probability weighted moment.
更多查看译文
关键词
wasserstein distance,distributional regression,theorem
AI 理解论文
溯源树
样例
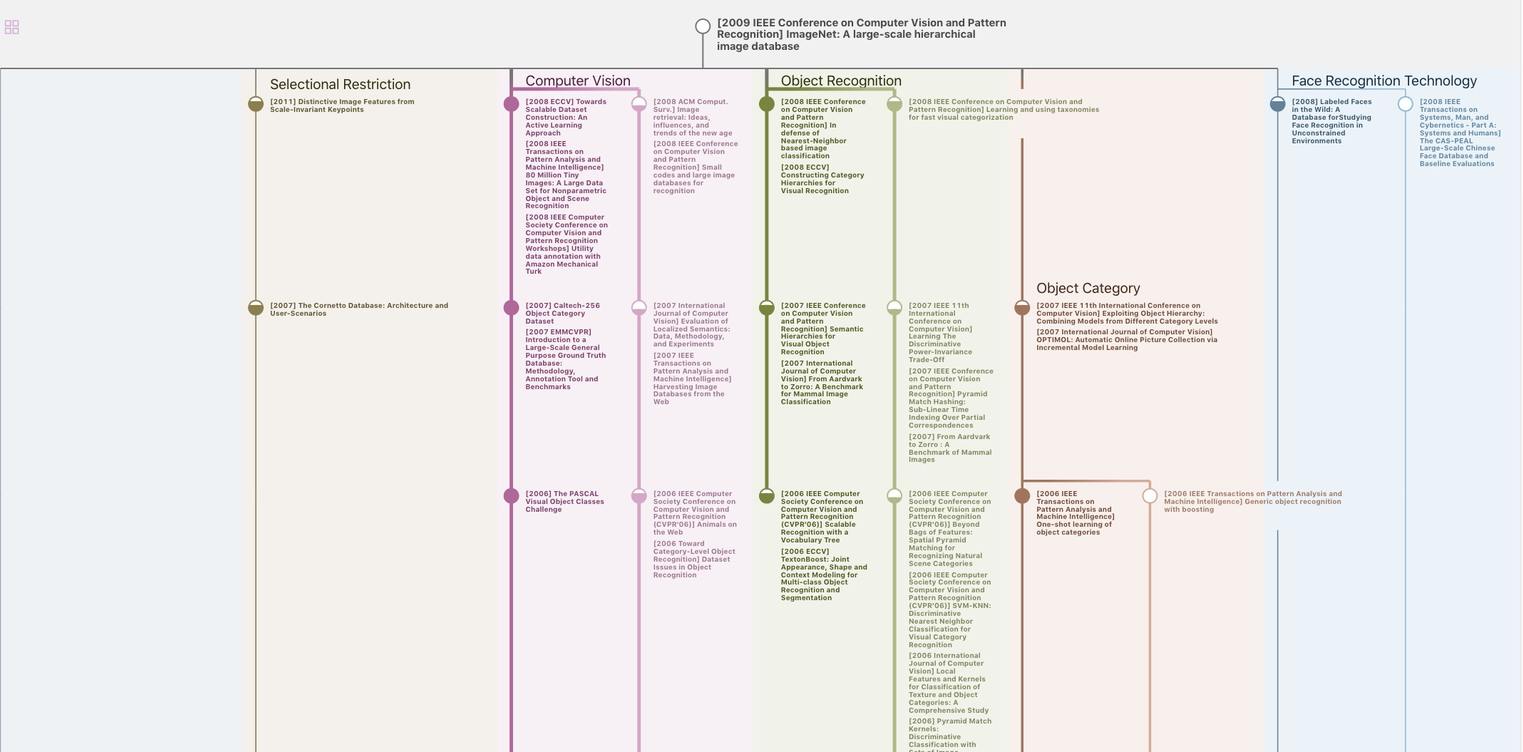
生成溯源树,研究论文发展脉络
Chat Paper
正在生成论文摘要