A Theoretical Justification for Image Inpainting using Denoising Diffusion Probabilistic Models
arxiv(2023)
摘要
We provide a theoretical justification for sample recovery using diffusion based image inpainting in a linear model setting. While most inpainting algorithms require retraining with each new mask, we prove that diffusion based inpainting generalizes well to unseen masks without retraining. We analyze a recently proposed popular diffusion based inpainting algorithm called RePaint (Lugmayr et al., 2022), and show that it has a bias due to misalignment that hampers sample recovery even in a two-state diffusion process. Motivated by our analysis, we propose a modified RePaint algorithm we call RePaint$^+$ that provably recovers the underlying true sample and enjoys a linear rate of convergence. It achieves this by rectifying the misalignment error present in drift and dispersion of the reverse process. To the best of our knowledge, this is the first linear convergence result for a diffusion based image inpainting algorithm.
更多查看译文
关键词
denoising
AI 理解论文
溯源树
样例
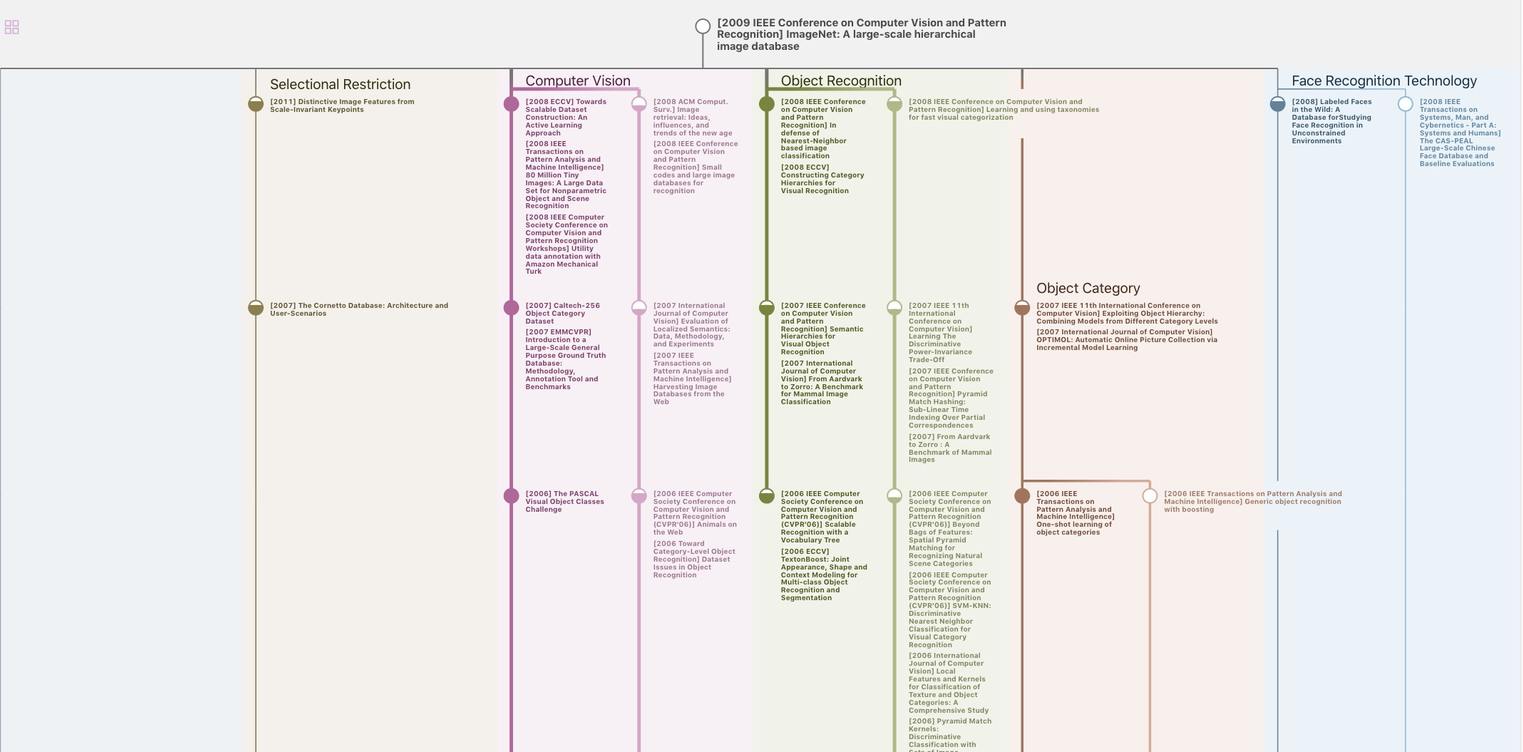
生成溯源树,研究论文发展脉络
Chat Paper
正在生成论文摘要