Is Model Ensemble Necessary? Model-based RL via a Single Model with Lipschitz Regularized Value Function
ICLR 2023(2023)
摘要
Probabilistic dynamics model ensemble is widely used in existing model-based reinforcement learning methods as it outperforms a single dynamics model in both asymptotic performance and sample efficiency. In this paper, we provide both practical and theoretical insights on the empirical success of the probabilistic dynamics model ensemble through the lens of Lipschitz continuity. We find that, for a value function, the stronger the Lipschitz condition is, the smaller the gap between the true dynamics- and learned dynamics-induced Bellman operators is, thus enabling the converged value function to be closer to the optimal value function. Hence, we hypothesize that the key functionality of the probabilistic dynamics model ensemble is to regularize the Lipschitz condition of the value function using generated samples. To test this hypothesis, we devise two practical robust training mechanisms through computing the adversarial noise and regularizing the value network's spectral norm to directly regularize the Lipschitz condition of the value functions. Empirical results show that combined with our mechanisms, model-based RL algorithms with a single dynamics model outperform those with an ensemble of probabilistic dynamics models. These findings not only support the theoretical insight, but also provide a practical solution for developing computationally efficient model-based RL algorithms.
更多查看译文
关键词
Model-based Reinforcement Learning,Probabilistic Dynamics Model Ensemble,Lipschitz Regularization
AI 理解论文
溯源树
样例
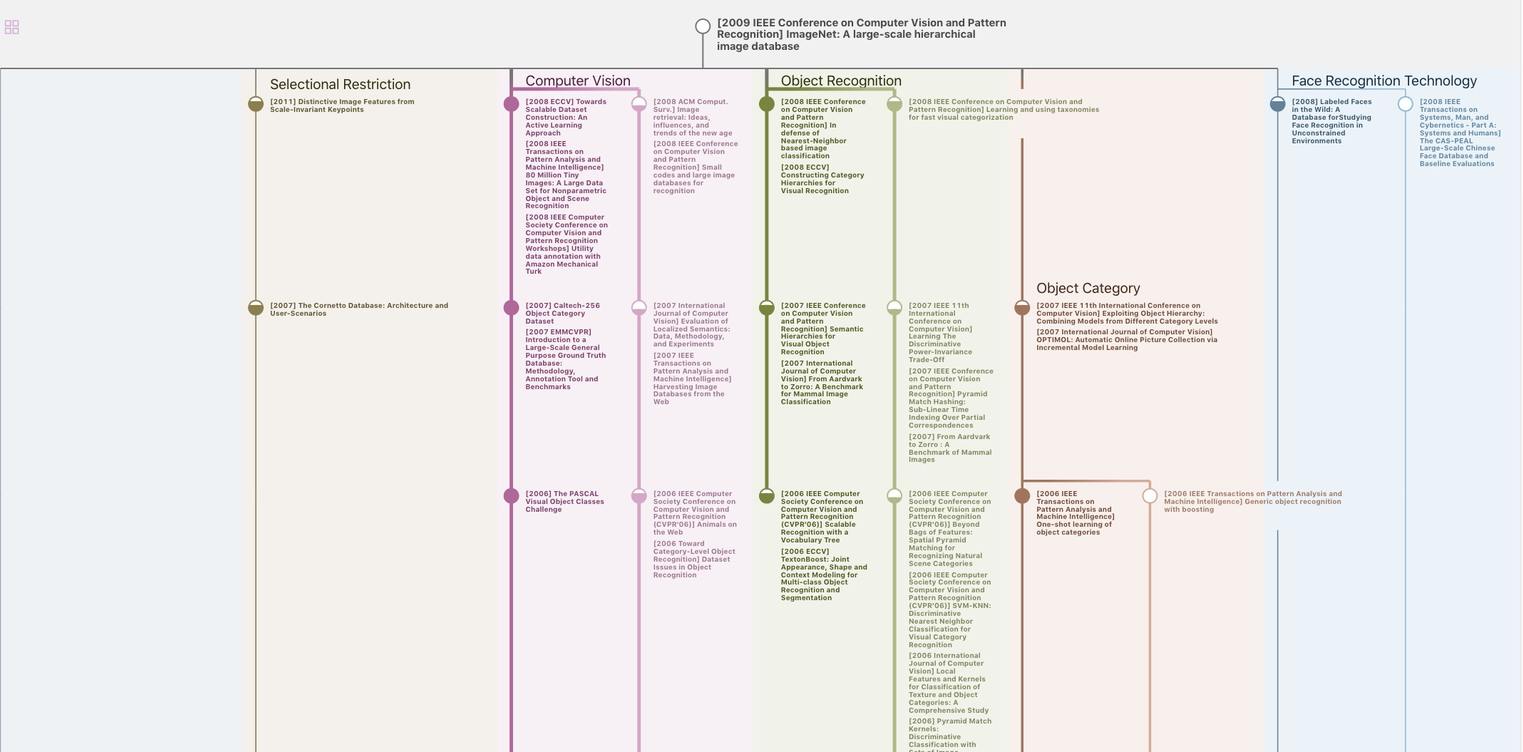
生成溯源树,研究论文发展脉络
Chat Paper
正在生成论文摘要