ReLOAD: Reinforcement Learning with Optimistic Ascent-Descent for Last-Iterate Convergence in Constrained MDPs
ICML(2023)
Abstract
In recent years, Reinforcement Learning (RL) has been applied to real-world problems with increasing success. Such applications often require to put constraints on the agent's behavior. Existing algorithms for constrained RL (CRL) rely on gradient descent-ascent, but this approach comes with a caveat. While these algorithms are guaranteed to converge on average, they do not guarantee last-iterate convergence, i.e., the current policy of the agent may never converge to the optimal solution. In practice, it is often observed that the policy alternates between satisfying the constraints and maximizing the reward, rarely accomplishing both objectives simultaneously. Here, we address this problem by introducing Reinforcement Learning with Optimistic Ascent-Descent (ReLOAD), a principled CRL method with guaranteed last-iterate convergence. We demonstrate its empirical effectiveness on a wide variety of CRL problems including discrete MDPs and continuous control. In the process we establish a benchmark of challenging CRL problems.
MoreTranslated text
Key words
reinforcement learning,ascent-descent,last-iterate
AI Read Science
Must-Reading Tree
Example
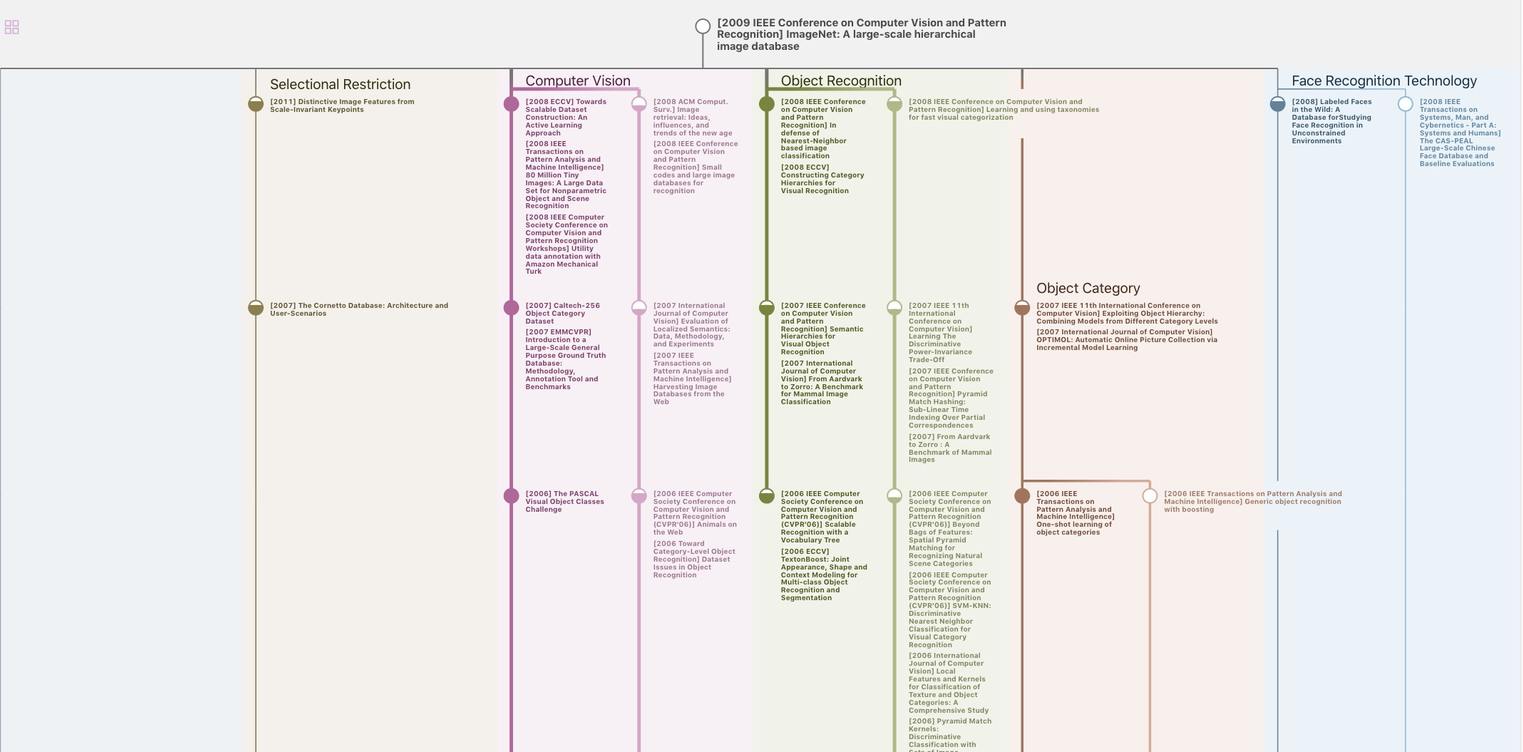
Generate MRT to find the research sequence of this paper
Chat Paper
Summary is being generated by the instructions you defined