Efficient Attention via Control Variates
ICLR 2023(2023)
摘要
Random-feature-based attention (RFA) is an efficient approximation of softmax attention with linear runtime and space complexity. However, the approximation gap between RFA and conventional softmax attention is not well studied. Built upon previous progress of RFA, we characterize this gap through the lens of control variates and show that RFA can be decomposed into a sum of multiple control variate estimators for each element in the sequence. This new framework reveals that exact softmax attention can be recovered from RFA by manipulating each control variate. Besides, it allows us to develop a more flexible form of control variates, resulting in a novel attention mechanism that significantly reduces the approximation gap while maintaining linear complexity. Extensive experiments demonstrate that our model outperforms state-of-the-art efficient attention mechanisms on both vision and language tasks.
更多查看译文
关键词
attention mechanism,transformers,random features,control variates,importance sampling
AI 理解论文
溯源树
样例
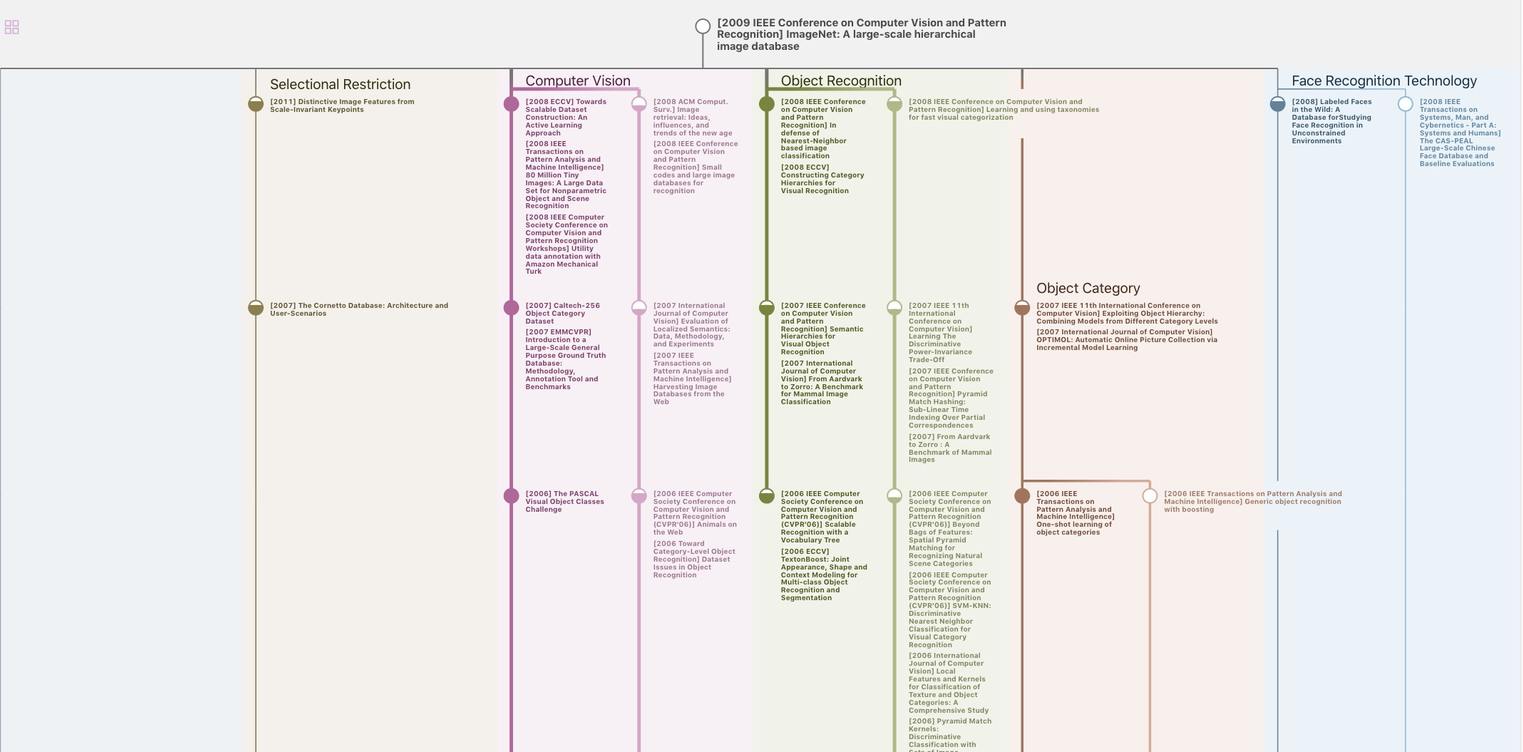
生成溯源树,研究论文发展脉络
Chat Paper
正在生成论文摘要