Multi-domain image generation and translation with identifiability guarantees
ICLR 2023(2023)
Abstract
Multi-domain image generation and unpaired image-to-to-image translation are two important and related computer vision problems. The common technique for the two tasks is the learning of a joint distribution from multiple marginal distributions. However, it is well known that there can be infinitely many joint distributions that can derive the same marginals. Hence, it is necessary to formulate suitable constraints to address this highly ill-posed problem. Inspired by the recent advances in nonlinear Independent Component Analysis (ICA) theory, we propose a new method to learn the joint distribution from the marginals by enforcing a specific type of minimal change across domains. We provide the formulations of minimal changes and some other assumptions, under which the true joint distribution across domains is identifiable. We also provide a practical implementation of multi-domain image generation and a technique to improve unpaired image-to-image translation. We apply our method to five multi-domain image generation and six image-to-image translation tasks. The superior performance of our model supports our theory and demonstrates the effectiveness of our method.
MoreTranslated text
Key words
multi-domain image generation,image translation,identifiability,Nonlinear ICA
AI Read Science
Must-Reading Tree
Example
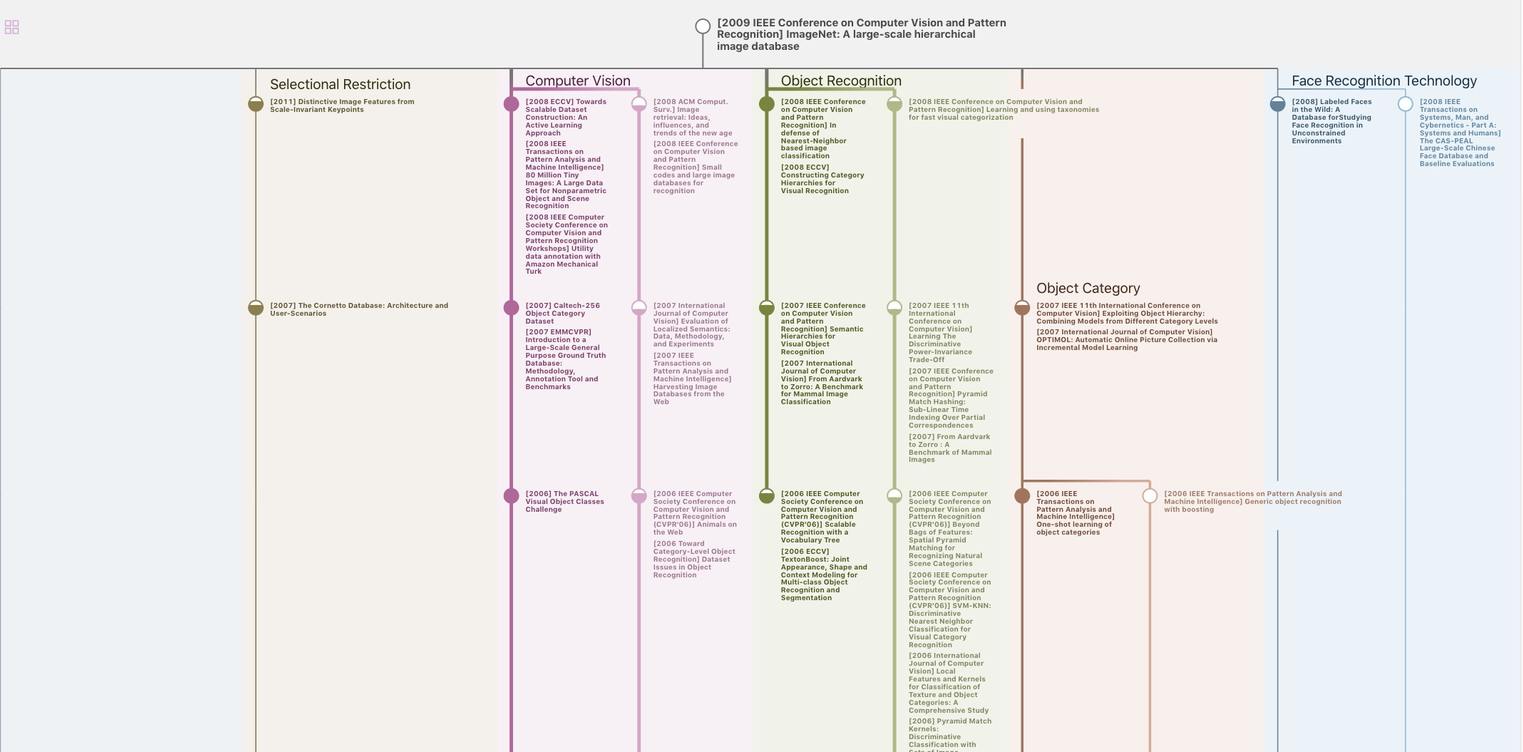
Generate MRT to find the research sequence of this paper
Chat Paper
Summary is being generated by the instructions you defined