Online Bias Correction for Task-Free Continual Learning
ICLR 2023(2023)
摘要
Task-free continual learning is the machine-learning setting where a model is trained online with data generated by a non-stationary stream. Conventional wisdom suggests that, in this setting, models are trained using an approach called experience replay, where the risk is computed both with respect to current stream observations and to a small subset of past observations. In this work, we show both theoretically and empirically how experience replay biases the outputs of the model towards recent stream observations. Moreover, we propose a simple approach to correct for this bias online, by changing the way the output layer of the model is optimized. We show that our approach improves significantly the learning performance of experience-replay approaches over a number of different datasets. Our findings suggest that, in contrast to stationary machine-learning problems, the output layer of a model should be optimized separately from its preceding layers when performing experience replay.
更多查看译文
关键词
Task-Free Continual Learning
AI 理解论文
溯源树
样例
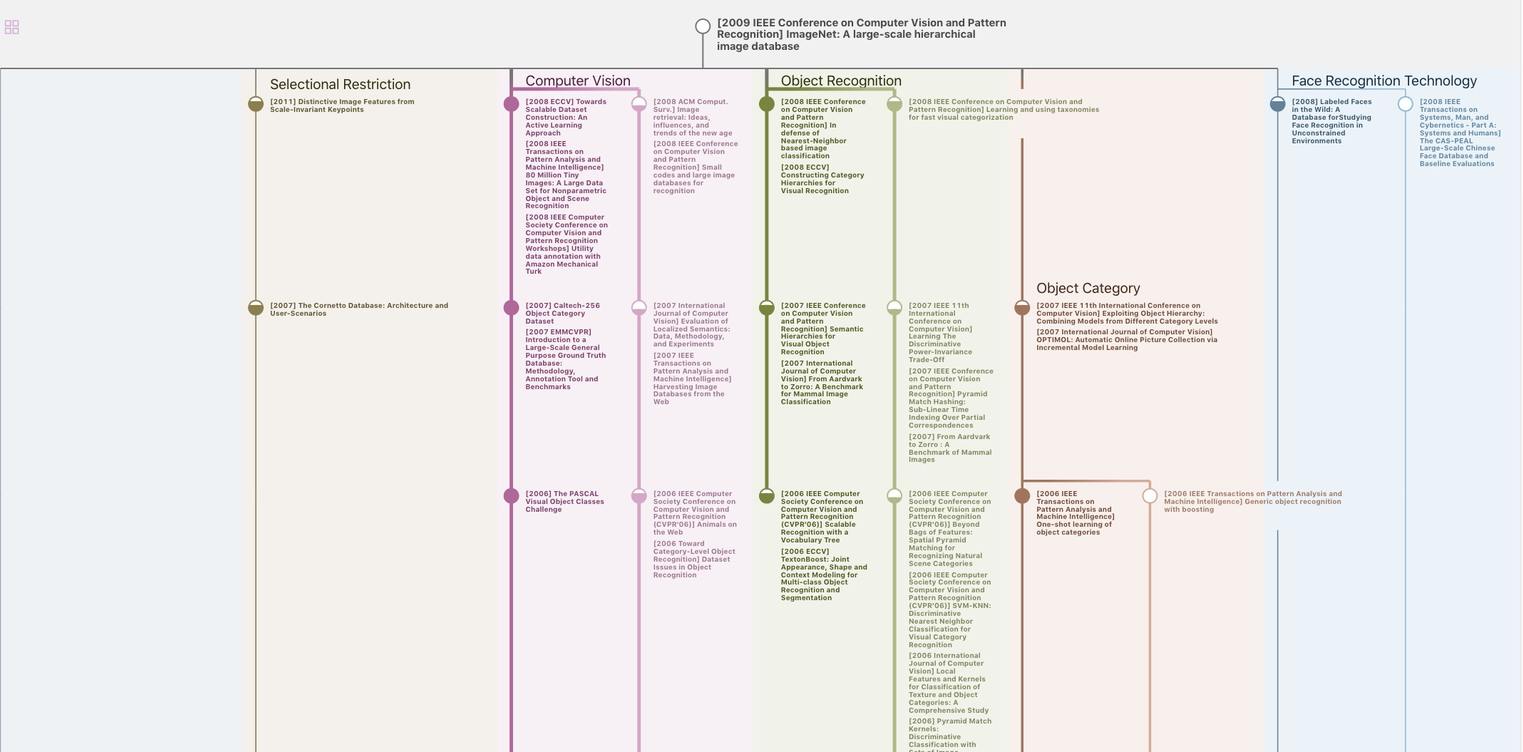
生成溯源树,研究论文发展脉络
Chat Paper
正在生成论文摘要