Interaction-Based Disentanglement of Entities for Object-Centric World Models
ICLR 2023(2023)
摘要
Perceiving the world compositionally in terms of space and time is essential to understanding object dynamics and solving downstream tasks. Object-centric learning using generative models has improved its ability to learn distinct representations of individual objects and predict their interactions, and it is a focal question how to utilize the learned representations to solve untrained, downstream tasks.
However, as models struggle to predict object interactions and track the objects accurately especially for unseen configurations, using object-centric representations in downstream tasks is yet a challenge. This paper proposes STEDIE, a new model that disentangles object representations based on interactions, into interaction-relevant relational features and interaction-irrelevant global features without supervision. Empirical evaluation shows that the proposed model factorizes global features unaffected by interactions from relational features that are necessary to predict outcome of interactions. We also show that STEDIE, by excluding features irrelevant in predicting interactions, achieves better performance in planning tasks and understanding causal relationships. In both tasks, our model not only achieves better performance in terms of reconstruction ability but also utilizes the disentangled representations to solve the tasks in a structured manner.
更多查看译文
关键词
object-centric,object-oriented,world models,self-supervised learning,probabilistic deep learning,structured models,video prediction,physics prediction,planning,variational autoencoders,model-based reinforcement learning,VAEs,unsupervised
AI 理解论文
溯源树
样例
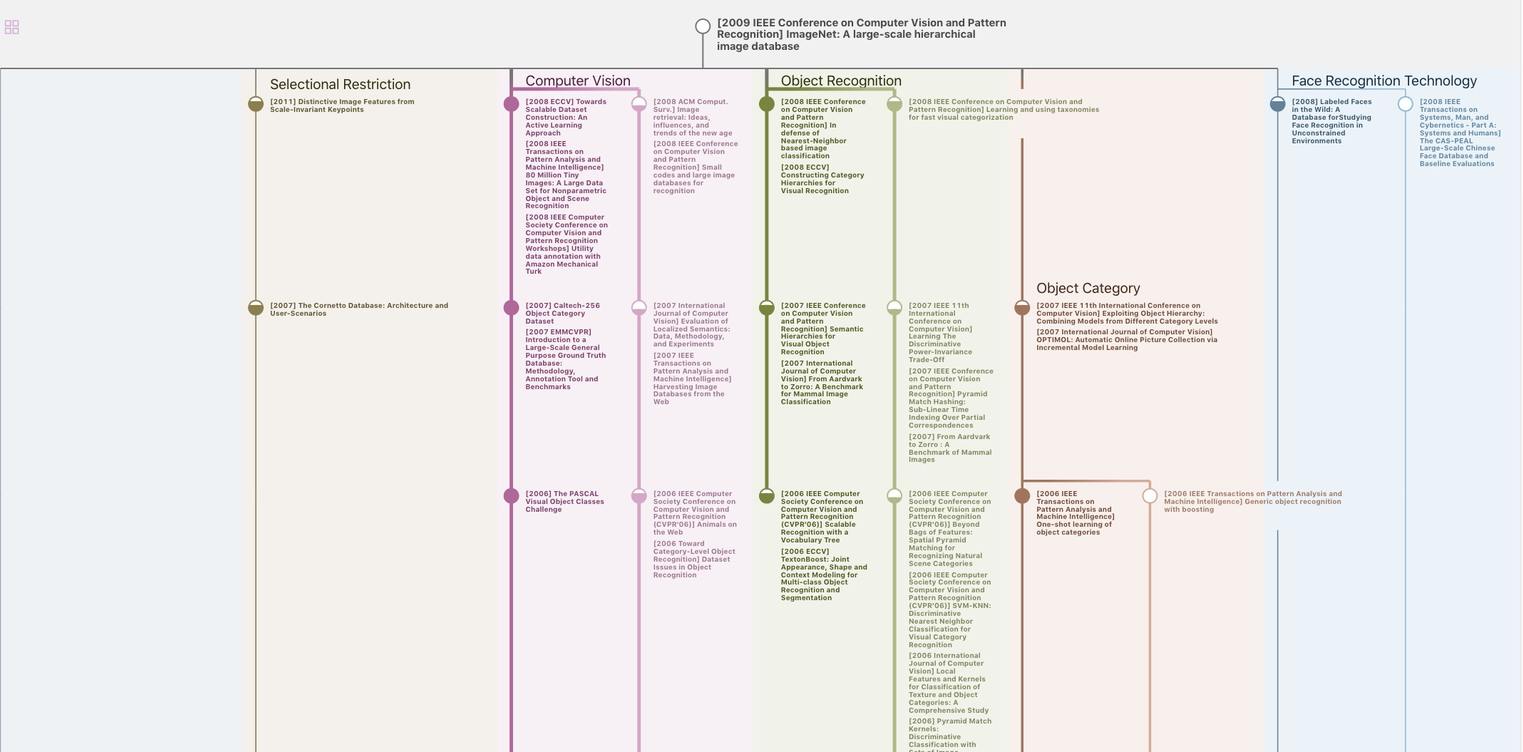
生成溯源树,研究论文发展脉络
Chat Paper
正在生成论文摘要