Composite Slice Transformer: An Efficient Transformer with Composition of Multi-Scale Multi-Range Attentions
ICLR 2023(2023)
摘要
Since the introduction of Transformers, researchers have tackled the notoriously expensive quadratic complexity problem. While significant computational efficiency improvements have been achieved, they come at the cost of reduced accuracy trade-offs. In this paper, we propose Composite Slice Transformer (CST), a Transformer-based network equipped with a composition of multi-scale multi-range attentions, boosting both efficiency and modeling capability.
After stacking fixed-length slices of the input sequence, each layer in CST performs a pair of fine-and-coarse-grained attentions with short-long ranges in a sequential manner, coupled with volatile instant positional embedding, enabling efficient token interactions {\em and} improving expressiveness of the model.
In addition to significantly reduced $O(NL+N^2/L^2)$ complexity for sequence length $N$ and slice length $L$, CST achieves superior performance on a variety of tasks. We show that CST surpasses recently published efficient Transformers on the Long Range Arena benchmark, demonstrating the bidirectional long-range dependency modeling capability of our model. It outperforms the standard Transformer by a margin of $6.9$\% in average accuracy across the five classification tasks of the benchmark, while being of complexity comparable to other efficient transformers. Furthermore, on the word-level autoregressive language modeling task with the WikiText-103 dataset, CST performs competitively against the Transformer model with only $2$\% gap in the test perplexity while outperforming other efficient Transformers.
更多查看译文
关键词
transformer,efficient transformer,efficient attention
AI 理解论文
溯源树
样例
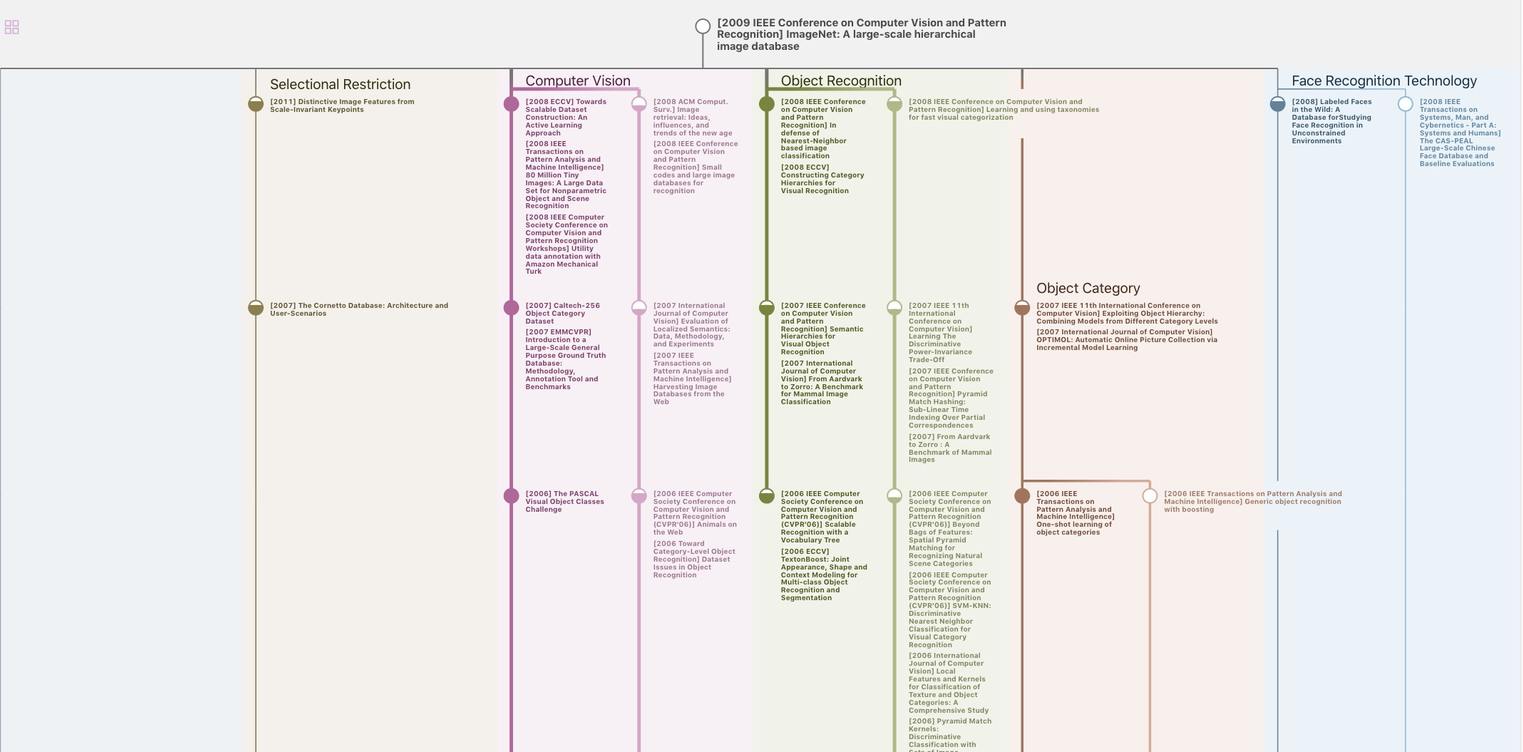
生成溯源树,研究论文发展脉络
Chat Paper
正在生成论文摘要