Quality-Similar Diversity via Population Based Reinforcement Learning
ICLR 2023(2023)
摘要
Diversity is a growing research topic in Reinforcement Learning (RL). Previous research on diversity has mainly focused on promoting diversity to encourage exploration and thereby improve quality (the cumulative reward), maximizing diversity subject to quality constraints, or jointly maximizing quality and diversity, known as the quality-diversity problem. In this work, we present the quality-similar diversity problem that features diversity among policies of similar qualities. In contrast to task-agnostic diversity, we focus on task-specific diversity defined by a set of user-specified Behavior Descriptors (BDs). A BD is a scalar function of a trajectory (e.g., the fire action rate for an Atari game), which delivers the type of diversity the user prefers. To derive the gradient of the user-specified diversity with respect to a policy, which is not trivially available, we introduce a set of BD estimators and connect it with the classical policy gradient theorem. Based on the diversity gradient, we develop a population-based RL algorithm to adaptively and efficiently optimize the population diversity at multiple quality levels throughout training. Extensive results on MuJoCo and Atari demonstrate that our algorithm significantly outperforms previous methods in terms of generating user-specified diverse policies across different quality levels.
更多查看译文
关键词
quality diversity,reinforcement learning,user-defined,population
AI 理解论文
溯源树
样例
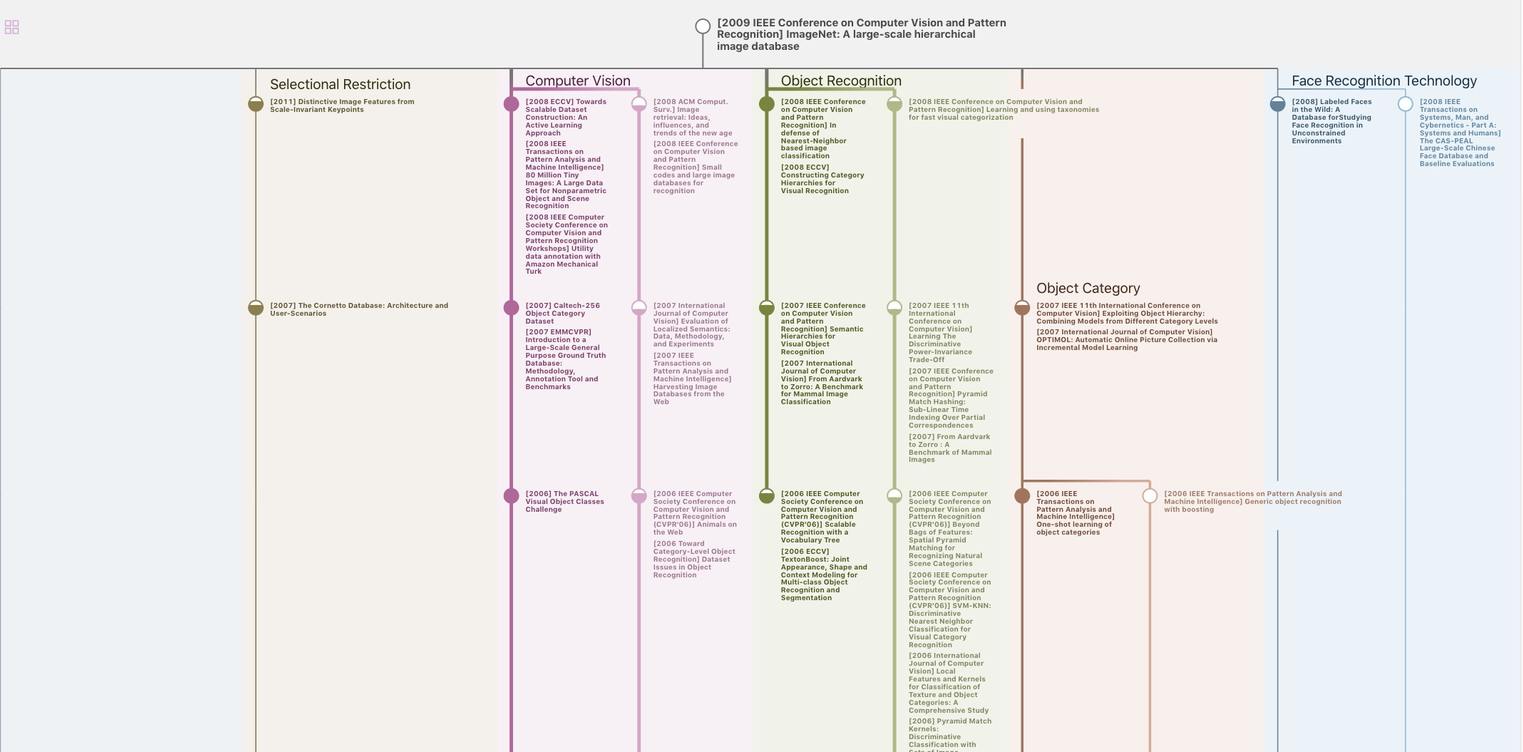
生成溯源树,研究论文发展脉络
Chat Paper
正在生成论文摘要