Learning to Extrapolate: A Transductive Approach
ICLR 2023(2023)
摘要
Machine learning systems, especially overparameterized deep neural networks, can generalize to novel testing instances drawn from the same distribution as the training data. However, they fare poorly when evaluated on out-of-support testing points. In this work, we tackle the problem of developing machine learning systems that retain the power of overparametrized function approximators, while enabling extrapolation to out-of-support testing points when possible. This is accomplished by noting that under certain conditions, a "transductive" reparameterization can convert an out-of-support extrapolation problem into a problem of within-support combinatorial generalization. We propose a simple strategy based on bilinear embeddings to enable this type of combinatorial generalization, thereby addressing the out-of-support extrapolation problem. We instantiate a simple, practical algorithm applicable to various supervised learning problems and imitation learning tasks.
更多查看译文
关键词
transductive approach,learning
AI 理解论文
溯源树
样例
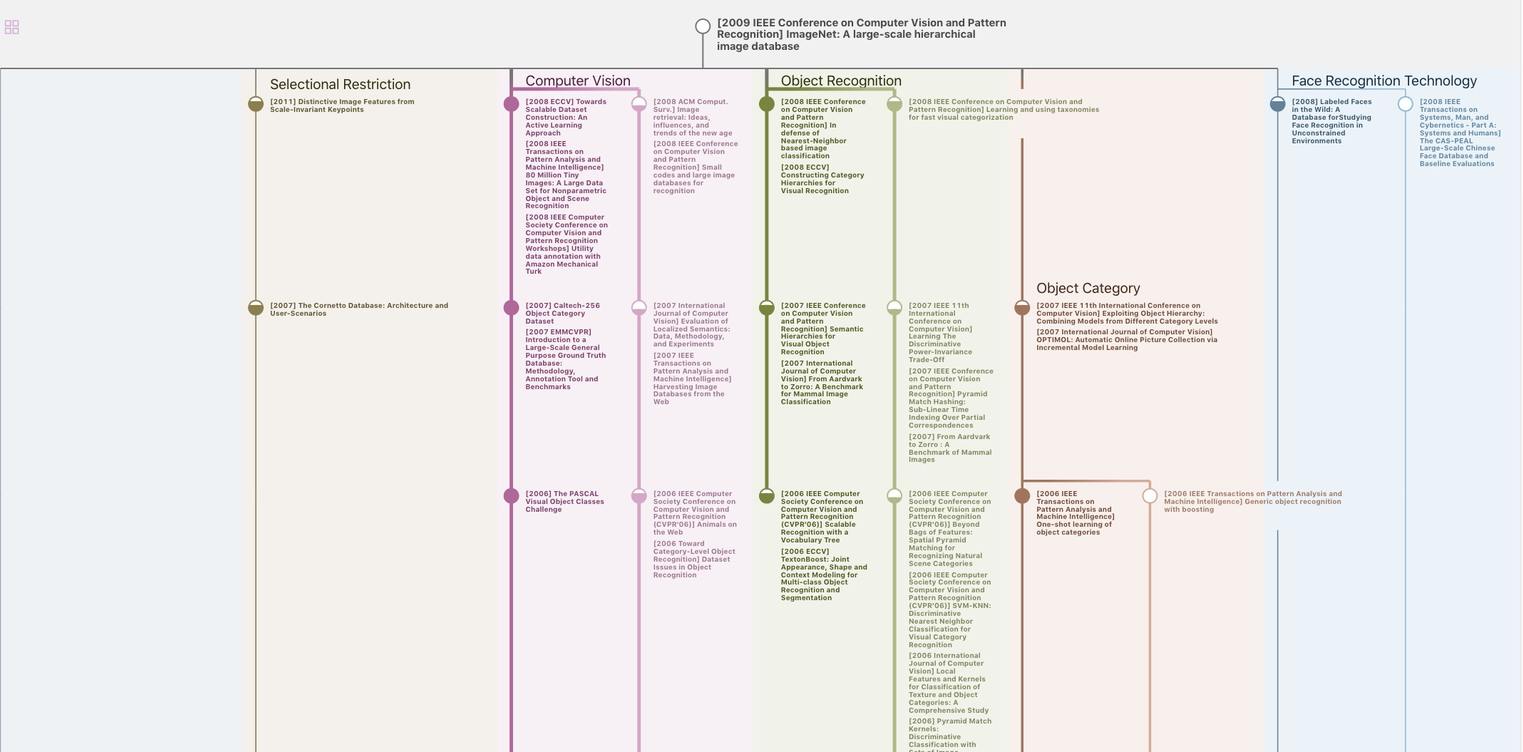
生成溯源树,研究论文发展脉络
Chat Paper
正在生成论文摘要